diff --git a/data/.ipynb_checkpoints/compiled_data-checkpoint.csv b/data/.ipynb_checkpoints/compiled_data-checkpoint.csv
deleted file mode 100644
index 97cf63c..0000000
--- a/data/.ipynb_checkpoints/compiled_data-checkpoint.csv
+++ /dev/null
@@ -1,314 +0,0 @@
-user,radius (cm),angle (deg)
-0,1.49,3.2
-0,6.21,45.4
-0,11.56,340.1
-0,4.12,273.4
-0,7.48,205.7
-0,9.43,133.2
-0,8.11,159.5
-0,5.59,21.4
-0,4.91,82.3
-1,10.74,115.8
-1,3.7,295
-1,2.4,300
-1,4.2,134
-1,0.6,236
-1,0.5,18
-1,3.3,16
-1,7.6,224
-1,6.1,290
-1,2.3,224
-2,4.9,8
-2,7.5,74
-2,4.5,56
-2,5.25,102
-2,6,92
-2,2,134
-2,4.5,16
-2,4,66
-2,13.25,284
-2,3,84
-3,7,290
-3,1.5,18
-3,6,63
-3,5,115
-3,4.5,122
-3,5.5,137
-3,0.5,205
-3,7,76
-3,2.5,117
-3,4,101
-4,2.5,281
-4,3,340
-4,5.5,185
-4,8,67
-4,3.5,7
-4,2.5,255
-4,3,290
-4,3.5,295
-4,4.5,250
-4,8.5,275
-5,10.5,304
-5,1.2,120
-5,16.6,90
-5,14.3,152
-5,14.4,150
-5,4.6,210
-5,5.9,100
-5,3.7,86
-5,14.2,10
-5,7.3,65
-6,10.1,88
-6,1,30
-6,4.5,60
-6,6,101
-6,6.1,110
-6,1.4,160
-6,0.8,200
-6,4,250
-6,3.7,273
-6,2.3,320
-7,1.4,340
-7,11,260
-7,12,53
-7,12.5,88
-7,8.2,138
-7,3.6,130
-7,2,15
-7,6.2,175
-7,9.5,225
-7,12.5,302
-8,4,268
-8,10,98
-8,4.6,83
-8,2.7,102
-8,3.4,42
-8,2.8,24
-8,3.4,-6
-8,3.5,-42
-8,2.9,-46
-8,3.8,-47
-9,5.6,-84
-9,4,310
-9,4,140
-9,8,155
-9,9,75
-9,12,85
-9,13,65
-9,15,110
-9,11,200
-9,14,190
-10,8,290
-10,8,140
-10,2.2,15
-10,9.7,97
-10,6,122
-10,7.5,330
-10,5.9,100
-10,3.1,190
-10,9.8,150
-10,10.2,45
-11,4.8,22
-11,10,92
-11,5.1,92
-11,5.4,122
-11,3.1,164
-11,2.6,338
-11,3.4,234
-11,5.7,295
-11,7.9,263
-11,8.9,259
-12,12.4,242
-12,8.2,85
-12,5.3,92
-12,3.1,105
-12,2.6,11
-12,6.4,65
-12,1.1,112
-12,3.5,121
-12,4.8,92
-12,3.2,76
-13,2,90
-13,6.6,216
-13,2.4,272
-13,3,320
-13,0.5,16
-13,4.3,44
-13,2.6,60
-13,1.1,124
-13,2.4,358
-13,6.1,86
-14,3.4,174
-14,14,128
-14,5,220
-14,12.5,338
-14,15.5,290
-14,13.5,284
-14,15,236
-14,4,290
-14,3,25
-14,6.5,85
-15,16.5,105
-15,2,50
-15,2.7,164
-15,4,194
-15,5,330
-15,5.2,262
-15,6,90
-15,8.1,305
-15,8.5,48
-15,8.5,136
-16,10,240
-16,3,275
-16,7,61
-16,2,51
-16,6,255
-16,12,64
-16,4,77
-16,4,184
-16,5,111
-16,8,119
-17,11,15
-17,3.2,0
-17,4.1,12
-17,2,20
-17,2.2,38
-17,3.2,39
-17,1.5,50
-17,2.9,52
-17,9.4,88
-17,3.9,180
-18,10.2,241
-18,5.5,20
-18,2,200
-18,7,170
-18,4.5,70
-18,6.5,330
-18,4,290
-18,1,240
-18,1.5,90
-18,6,120
-19,4.5,280
-19,2.4,15
-19,6.7,45
-19,3.5,121
-19,6.9,126
-19,4.5,129
-19,3.8,134
-19,4.3,221
-19,5,257
-19,2.4,322
-20,1.8,337
-20,7.75,9
-20,7.6,82.5
-20,5.4,122
-20,5.8,154.5
-20,7.2,211
-20,4.5,220
-20,3.6,260
-20,5,278
-20,3.8,316
-21,6.5,339
-21,10,86
-21,6.5,102
-21,8,124
-21,13,78
-21,3.5,270
-21,4,180
-21,17,251
-21,9,93
-21,4.5,90
-22,6,270
-22,5,8.5
-23,2.5,317
-23,7,35
-23,9,166
-23,2,112
-23,14,330
-23,13,240
-23,5,105
-23,11,60
-23,4,52
-23,8,262
-24,13,155
-24,8.9,9
-24,5,82
-24,5.4,290
-24,5.8,20
-24,7.2,220
-24,4.5,180
-24,3.6,270
-24,5,278
-24,3.8,316
-25,6.5,339
-25,4,95
-25,12,90
-25,11.5,67
-25,12.5,35
-25,11,30
-25,6,357
-25,13,300
-25,18,308
-25,10,182
-26,13,235
-26,3,155
-26,1.5,50
-26,1.6,172
-26,0.75,80
-26,2.5,12.5
-26,1.5,145
-26,1.75,95
-26,2.25,170
-26,1.75,110
-27,0.75,140
-27,3.5,108
-27,8,252
-27,12.5,240
-27,14,194
-27,1.5,86
-27,8,124
-27,10.5,64
-27,8,46
-27,13,0
-28,12.5,62
-28,4.8,37
-28,2.2,44
-28,2.5,96
-28,4.6,104
-28,4.1,115
-28,2.3,135
-28,3.8,140
-28,2.9,197
-28,5.1,244
-29,2.3,287
-29,7.2,276
-29,13.5,284
-29,10.2,272
-29,2.5,72
-29,12.8,258
-29,6.9,236
-29,7.2,70
-29,8.5,112
-29,9,294
-30,13.5,256
-30,4,24
-30,8,257
-30,2,75
-30,5,268
-30,8,142
-30,6,200
-30,3,340
-30,5,16
-30,6,39
-31,4,127
-31,7,150
-31,1,30
-31,2.5,45
-31,3,62
-31,2.8,110
-31,4,246
-31,1.7,155
-31,3.6,176
-31,0.5,18
-32,3.3,335
-32,4.7,286
diff --git a/data/archery.jpg b/data/archery.jpg
deleted file mode 100644
index 9f99bbf..0000000
Binary files a/data/archery.jpg and /dev/null differ
diff --git a/data/compiled_data.csv b/data/compiled_data.csv
deleted file mode 100644
index 97cf63c..0000000
--- a/data/compiled_data.csv
+++ /dev/null
@@ -1,314 +0,0 @@
-user,radius (cm),angle (deg)
-0,1.49,3.2
-0,6.21,45.4
-0,11.56,340.1
-0,4.12,273.4
-0,7.48,205.7
-0,9.43,133.2
-0,8.11,159.5
-0,5.59,21.4
-0,4.91,82.3
-1,10.74,115.8
-1,3.7,295
-1,2.4,300
-1,4.2,134
-1,0.6,236
-1,0.5,18
-1,3.3,16
-1,7.6,224
-1,6.1,290
-1,2.3,224
-2,4.9,8
-2,7.5,74
-2,4.5,56
-2,5.25,102
-2,6,92
-2,2,134
-2,4.5,16
-2,4,66
-2,13.25,284
-2,3,84
-3,7,290
-3,1.5,18
-3,6,63
-3,5,115
-3,4.5,122
-3,5.5,137
-3,0.5,205
-3,7,76
-3,2.5,117
-3,4,101
-4,2.5,281
-4,3,340
-4,5.5,185
-4,8,67
-4,3.5,7
-4,2.5,255
-4,3,290
-4,3.5,295
-4,4.5,250
-4,8.5,275
-5,10.5,304
-5,1.2,120
-5,16.6,90
-5,14.3,152
-5,14.4,150
-5,4.6,210
-5,5.9,100
-5,3.7,86
-5,14.2,10
-5,7.3,65
-6,10.1,88
-6,1,30
-6,4.5,60
-6,6,101
-6,6.1,110
-6,1.4,160
-6,0.8,200
-6,4,250
-6,3.7,273
-6,2.3,320
-7,1.4,340
-7,11,260
-7,12,53
-7,12.5,88
-7,8.2,138
-7,3.6,130
-7,2,15
-7,6.2,175
-7,9.5,225
-7,12.5,302
-8,4,268
-8,10,98
-8,4.6,83
-8,2.7,102
-8,3.4,42
-8,2.8,24
-8,3.4,-6
-8,3.5,-42
-8,2.9,-46
-8,3.8,-47
-9,5.6,-84
-9,4,310
-9,4,140
-9,8,155
-9,9,75
-9,12,85
-9,13,65
-9,15,110
-9,11,200
-9,14,190
-10,8,290
-10,8,140
-10,2.2,15
-10,9.7,97
-10,6,122
-10,7.5,330
-10,5.9,100
-10,3.1,190
-10,9.8,150
-10,10.2,45
-11,4.8,22
-11,10,92
-11,5.1,92
-11,5.4,122
-11,3.1,164
-11,2.6,338
-11,3.4,234
-11,5.7,295
-11,7.9,263
-11,8.9,259
-12,12.4,242
-12,8.2,85
-12,5.3,92
-12,3.1,105
-12,2.6,11
-12,6.4,65
-12,1.1,112
-12,3.5,121
-12,4.8,92
-12,3.2,76
-13,2,90
-13,6.6,216
-13,2.4,272
-13,3,320
-13,0.5,16
-13,4.3,44
-13,2.6,60
-13,1.1,124
-13,2.4,358
-13,6.1,86
-14,3.4,174
-14,14,128
-14,5,220
-14,12.5,338
-14,15.5,290
-14,13.5,284
-14,15,236
-14,4,290
-14,3,25
-14,6.5,85
-15,16.5,105
-15,2,50
-15,2.7,164
-15,4,194
-15,5,330
-15,5.2,262
-15,6,90
-15,8.1,305
-15,8.5,48
-15,8.5,136
-16,10,240
-16,3,275
-16,7,61
-16,2,51
-16,6,255
-16,12,64
-16,4,77
-16,4,184
-16,5,111
-16,8,119
-17,11,15
-17,3.2,0
-17,4.1,12
-17,2,20
-17,2.2,38
-17,3.2,39
-17,1.5,50
-17,2.9,52
-17,9.4,88
-17,3.9,180
-18,10.2,241
-18,5.5,20
-18,2,200
-18,7,170
-18,4.5,70
-18,6.5,330
-18,4,290
-18,1,240
-18,1.5,90
-18,6,120
-19,4.5,280
-19,2.4,15
-19,6.7,45
-19,3.5,121
-19,6.9,126
-19,4.5,129
-19,3.8,134
-19,4.3,221
-19,5,257
-19,2.4,322
-20,1.8,337
-20,7.75,9
-20,7.6,82.5
-20,5.4,122
-20,5.8,154.5
-20,7.2,211
-20,4.5,220
-20,3.6,260
-20,5,278
-20,3.8,316
-21,6.5,339
-21,10,86
-21,6.5,102
-21,8,124
-21,13,78
-21,3.5,270
-21,4,180
-21,17,251
-21,9,93
-21,4.5,90
-22,6,270
-22,5,8.5
-23,2.5,317
-23,7,35
-23,9,166
-23,2,112
-23,14,330
-23,13,240
-23,5,105
-23,11,60
-23,4,52
-23,8,262
-24,13,155
-24,8.9,9
-24,5,82
-24,5.4,290
-24,5.8,20
-24,7.2,220
-24,4.5,180
-24,3.6,270
-24,5,278
-24,3.8,316
-25,6.5,339
-25,4,95
-25,12,90
-25,11.5,67
-25,12.5,35
-25,11,30
-25,6,357
-25,13,300
-25,18,308
-25,10,182
-26,13,235
-26,3,155
-26,1.5,50
-26,1.6,172
-26,0.75,80
-26,2.5,12.5
-26,1.5,145
-26,1.75,95
-26,2.25,170
-26,1.75,110
-27,0.75,140
-27,3.5,108
-27,8,252
-27,12.5,240
-27,14,194
-27,1.5,86
-27,8,124
-27,10.5,64
-27,8,46
-27,13,0
-28,12.5,62
-28,4.8,37
-28,2.2,44
-28,2.5,96
-28,4.6,104
-28,4.1,115
-28,2.3,135
-28,3.8,140
-28,2.9,197
-28,5.1,244
-29,2.3,287
-29,7.2,276
-29,13.5,284
-29,10.2,272
-29,2.5,72
-29,12.8,258
-29,6.9,236
-29,7.2,70
-29,8.5,112
-29,9,294
-30,13.5,256
-30,4,24
-30,8,257
-30,2,75
-30,5,268
-30,8,142
-30,6,200
-30,3,340
-30,5,16
-30,6,39
-31,4,127
-31,7,150
-31,1,30
-31,2.5,45
-31,3,62
-31,2.8,110
-31,4,246
-31,1.7,155
-31,3.6,176
-31,0.5,18
-32,3.3,335
-32,4.7,286
diff --git a/data/hits_im1.npy b/data/hits_im1.npy
new file mode 100644
index 0000000..f808cdd
Binary files /dev/null and b/data/hits_im1.npy differ
diff --git a/data/hits_im2.npy b/data/hits_im2.npy
new file mode 100644
index 0000000..445030c
Binary files /dev/null and b/data/hits_im2.npy differ
diff --git a/data/target_01.jpg b/data/target_01.jpg
new file mode 100644
index 0000000..29a83ca
Binary files /dev/null and b/data/target_01.jpg differ
diff --git a/data/target_02.jpg b/data/target_02.jpg
new file mode 100644
index 0000000..5ad0f3a
Binary files /dev/null and b/data/target_02.jpg differ
diff --git a/data/target_data.csv b/data/target_data.csv
new file mode 100644
index 0000000..77a57ba
--- /dev/null
+++ b/data/target_data.csv
@@ -0,0 +1,55 @@
+throw #, x position (m), y position (m),picture x position (pixel), picture y position (pixel),target x position (pixel), target y position (pixel), image #
+0,-0.4664032892922447,-0.3040002885922146,1260.8551136363637,990.5994318181815,2055.1692559776675,1508.3310474572158,1
+1,-0.20697765114141842,-0.4481256431204515,1702.6732954545455,745.144886363636,2055.1692559776675,1508.3310474572158,1
+2,-0.09167736751882886,-0.45773400008900067,1899.036931818182,728.7812499999995,2055.1692559776675,1508.3310474572158,1
+3,0.1533357351791739,-0.5105799634160209,2316.3096590909095,638.7812499999995,2055.1692559776675,1508.3310474572158,1
+4,0.3647195884872546,-0.5970551761329631,2676.3096590909095,491.50852272727207,2055.1692559776675,1508.3310474572158,1
+5,-0.1205024384244762,-0.22713343284382184,1849.946022727273,1121.508522727272,2055.1692559776675,1508.3310474572158,1
+6,-0.09167736751882886,-0.25595850374946916,1899.036931818182,1072.417613636363,2055.1692559776675,1508.3310474572158,1
+7,-0.09648154600310332,-0.17909164800107613,1890.855113636364,1203.326704545454,2055.1692559776675,1508.3310474572158,1
+8,-0.19256511568859477,-0.04457465044138831,1727.21875,1432.4176136363633,2055.1692559776675,1508.3310474572158,1
+9,-0.08206901055027967,-0.02535793650429005,1915.4005681818185,1465.144886363636,2055.1692559776675,1508.3310474572158,1
+10,0.16294409214772285,-0.3136086455607638,2332.6732954545455,974.235795454545,2055.1692559776675,1508.3310474572158,1
+11,0.24941930486466507,-0.3328253594978622,2479.946022727273,941.5085227272721,2055.1692559776675,1508.3310474572158,1
+12,0.4415864442356477,-0.3712587873720587,2807.2187500000005,876.0539772727266,2055.1692559776675,1508.3310474572158,1
+13,0.03803545155658435,-0.00614122256719179,2119.946022727273,1497.8721590909088,2055.1692559776675,1508.3310474572158,1
+14,0.07646887943078087,-0.05898718589421197,2185.4005681818185,1407.8721590909088,2055.1692559776675,1508.3310474572158,1
+15,0.1341190212420755,-0.07339972134703576,2283.5823863636365,1383.326704545454,2055.1692559776675,1508.3310474572158,1
+16,0.1341190212420755,-0.15987493406397774,2283.5823863636365,1236.053977272727,2055.1692559776675,1508.3310474572158,1
+17,0.16294409214772285,-0.020553758020015585,2332.6732954545455,1473.326704545454,2055.1692559776675,1508.3310474572158,1
+18,0.2782443757703124,-0.07339972134703576,2529.036931818182,1383.326704545454,2055.1692559776675,1508.3310474572158,1
+19,0.30226526819168525,-0.09742061376840862,2569.946022727273,1342.417613636363,2055.1692559776675,1508.3310474572158,1
+20,0.21098587699046856,0.09474652560257397,2414.4914772727275,1669.6903409090905,2055.1692559776675,1508.3310474572158,1
+21,0.3310903390973326,0.15720084589814334,2619.036931818182,1776.053977272727,2055.1692559776675,1508.3310474572158,1
+22,0.11490230730497739,0.2244593446779872,2250.855113636364,1890.5994318181815,2055.1692559776675,1508.3310474572158,1
+23,0.1341190212420755,0.33975962830057677,2283.5823863636365,2086.963068181818,2055.1692559776675,1508.3310474572158,1
+24,-0.15413168781439823,0.18602591680379066,1792.6732954545455,1825.144886363636,2055.1692559776675,1508.3310474572158,1
+25,-0.17334840175149638,0.15720084589814334,1759.946022727273,1776.053977272727,2055.1692559776675,1508.3310474572158,1
+26,-0.30786539931118434,0.14278831044531956,1530.8551136363635,1751.5085227272723,2055.1692559776675,1508.3310474572158,1
+27,-0.3126695777954588,0.37338887769049856,1522.6732954545455,2144.235795454545,2055.1692559776675,1508.3310474572158,1
+28,-0.49042418171361757,0.3974097701118714,1219.9460227272727,2185.144886363636,2055.1692559776675,1508.3310474572158,1
+29,-0.7114163919902475,0.33495544981630226,843.5823863636363,2078.78125,2055.1692559776675,1508.3310474572158,1
+30,-0.29131239559921246,-0.12719786197191166,1412.21875,1254.9744318181815,2019.26155624037,1520.031972265023,2
+31,-0.12640552037257052,-0.3038837997147423,1755.855113636364,886.7926136363631,2019.26155624037,1520.031972265023,2
+32,-0.1970798954697028,-0.15860869534841487,1608.5823863636365,1189.519886363636,2019.26155624037,1520.031972265023,2
+33,-0.23241708301826883,-0.07615525773509413,1534.946022727273,1361.3380681818176,2019.26155624037,1520.031972265023,2
+34,-0.11855281202844486,-0.0447444243585908,1772.21875,1426.7926136363633,2019.26155624037,1520.031972265023,2
+35,-0.07143656196368994,-0.10756609111159712,1870.4005681818185,1295.8835227272725,2019.26155624037,1520.031972265023,2
+36,-0.04395208275924976,-0.1900195287249181,1927.6732954545455,1124.0653409090905,2019.26155624037,1520.031972265023,2
+37,0.011016875649630815,-0.1468296328322262,2042.21875,1214.0653409090905,2019.26155624037,1520.031972265023,2
+38,0.03850135485407121,-0.315662862230931,2099.4914772727275,862.2471590909086,2019.26155624037,1520.031972265023,2
+39,0.09347031326295178,-0.1311242161439747,2214.036931818182,1246.792613636363,2019.26155624037,1520.031972265023,2
+40,0.16807104253214689,-0.17038775786460356,2369.4914772727275,1164.9744318181815,2019.26155624037,1520.031972265023,2
+41,0.22696635511309052,-0.24498848713379867,2492.2187500000005,1009.519886363636,2019.26155624037,1520.031972265023,2
+42,0.6038963556311289,-0.31958921640299376,3277.673295454546,854.0653409090905,2019.26155624037,1520.031972265023,2
+43,0.30549343855434846,-0.013333590982087582,2655.855113636364,1492.2471590909088,2019.26155624037,1520.031972265023,2
+44,0.1955555217365873,-0.06830254939096826,2426.764204545455,1377.701704545454,2019.26155624037,1520.031972265023,2
+45,0.11702843829532915,-0.03689171601446505,2263.127840909091,1443.1562499999995,2019.26155624037,1520.031972265023,2
+46,0.018869583993756673,0.08875161749154782,2058.5823863636365,1704.9744318181815,2019.26155624037,1520.031972265023,2
+47,0.16414468836008408,0.10053068000773649,2361.3096590909095,1729.519886363636,2019.26155624037,1520.031972265023,2
+48,0.2897880218660969,0.16727870093280575,2623.1278409090914,1868.610795454545,2019.26155624037,1520.031972265023,2
+49,0.525369272189871,0.06911984663123327,3114.0369318181824,1664.0653409090905,2019.26155624037,1520.031972265023,2
+50,-0.12640552037257052,0.3635964095359507,1755.855113636364,2277.701704545454,2019.26155624037,1520.031972265023,2
+51,-0.2441961455344575,0.40285995125657975,1510.4005681818185,2359.519886363636,2019.26155624037,1520.031972265023,2
+52,-0.2127853121579543,0.5638404723111586,1575.855113636364,2694.9744318181815,2019.26155624037,1520.031972265023,2
+53,-0.23241708301826883,0.2889956802667558,1534.946022727273,2122.247159090909,2019.26155624037,1520.031972265023,2
diff --git a/images/target.png b/images/target.png
new file mode 100644
index 0000000..583533f
Binary files /dev/null and b/images/target.png differ
diff --git a/images/target.svg b/images/target.svg
new file mode 100644
index 0000000..7b09eac
--- /dev/null
+++ b/images/target.svg
@@ -0,0 +1,336 @@
+
+
+
+
diff --git a/notebooks/.ipynb_checkpoints/01_Cheers_Stats_Beers-checkpoint.ipynb b/notebooks/.ipynb_checkpoints/01_Cheers_Stats_Beers-checkpoint.ipynb
index fa8752b..8d868a6 100644
--- a/notebooks/.ipynb_checkpoints/01_Cheers_Stats_Beers-checkpoint.ipynb
+++ b/notebooks/.ipynb_checkpoints/01_Cheers_Stats_Beers-checkpoint.ipynb
@@ -4,7 +4,7 @@
"cell_type": "markdown",
"metadata": {},
"source": [
- "###### Content under Creative Commons Attribution license CC-BY 4.0, code under BSD 3-Clause License © 2019 Ryan C. Cooper"
+ "###### Content modified under Creative Commons Attribution license CC-BY 4.0, code under BSD 3-Clause License © 2020 Ryan C. Cooper"
]
},
{
@@ -853,7 +853,11 @@
"\n",
"Why? This gets a little technical, but the reason is that if you have a _sample_ of the population, you don't know the _real_ value of the mean, and $\\bar{x}$ is actually an _estimate_ of the mean. That's why you'll often find the symbol $\\mu$ used to denote the population mean, and distinguish it with the sample mean, $\\bar{x}$. Using $\\bar{x}$ to compute the standard deviation introduces a small bias: $\\bar{x}$ is computed _from the sample values_, and the data are on average (slightly) closer to $\\bar{x}$ than the population is to $\\mu$. Dividing by $N-1$ instead of $N$ corrects this bias!\n",
"\n",
- "Prof. Sainani explains it by saying that we lost one degree of freedom when we estimated the mean using $\\bar{x}$. For example, say we have 100 people and I give you their mean age, and the actual age for 99 people from the sample: you'll be able to calculate the age of that 100th person. Once we calculated the mean, we only have 99 degrees of freedom left because that 100th person's age is fixed. "
+ "Prof. Sainani explains it by saying that we lost one degree of freedom when we estimated the mean using $\\bar{x}$. For example, say we have 100 people and I give you their mean age, and the actual age for 99 people from the sample: you'll be able to calculate the age of that 100th person. Once we calculated the mean, we only have 99 degrees of freedom left because that 100th person's age is fixed. \n",
+ "\n",
+ "Below is a graphical distinction between the _sample_ and the _population_ from [@allison_horst on twitter](https://twitter.com/allison_horst)\n",
+ "\n",
+ "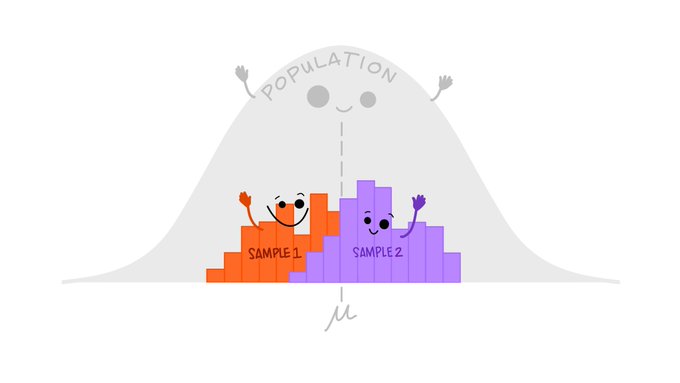\n"
]
},
{
diff --git a/notebooks/.ipynb_checkpoints/02_Seeing_Stats-checkpoint.ipynb b/notebooks/.ipynb_checkpoints/02_Seeing_Stats-checkpoint.ipynb
index 1193c0a..a25d3aa 100644
--- a/notebooks/.ipynb_checkpoints/02_Seeing_Stats-checkpoint.ipynb
+++ b/notebooks/.ipynb_checkpoints/02_Seeing_Stats-checkpoint.ipynb
@@ -4,7 +4,7 @@
"cell_type": "markdown",
"metadata": {},
"source": [
- "###### Modified under Creative Commons Attribution license CC-BY 4.0, code under BSD 3-Clause License © 2020 R.C. Cooper"
+ "###### Content modified under Creative Commons Attribution license CC-BY 4.0, code under BSD 3-Clause License © 2020 R.C. Cooper"
]
},
{
@@ -484,7 +484,7 @@
"##### Exercise:\n",
"\n",
"* Go to the documentation of [`np.var()`](https://docs.scipy.org/doc/numpy-1.13.0/reference/generated/numpy.var.html) and analyze if this function is computing the _sample variance_. \n",
- "**Hint**: Check what it says about the \"data degrees of freedom.\"\n",
+ "__Hint__: Check what it says about the \"data degrees of freedom.\"\n",
"\n",
"If you did the reading, you might have noticed that, by default, the argument `ddof` in `np.var()` is set to zero. If we use the default option, then we are not really calculating the sample variance. Recall from the previous lesson that the **sample variance** is:\n",
"\n",
diff --git a/notebooks/.ipynb_checkpoints/04_Stats_and_Montecarlo-checkpoint.ipynb b/notebooks/.ipynb_checkpoints/04_Stats_and_Montecarlo-checkpoint.ipynb
index 7e22206..e24a004 100644
--- a/notebooks/.ipynb_checkpoints/04_Stats_and_Montecarlo-checkpoint.ipynb
+++ b/notebooks/.ipynb_checkpoints/04_Stats_and_Montecarlo-checkpoint.ipynb
@@ -4,7 +4,7 @@
"cell_type": "markdown",
"metadata": {},
"source": [
- "###### Created under Creative Commons Attribution license CC-BY 4.0, code under BSD 3-Clause License © 2020 R.C. Cooper"
+ "###### Content created under Creative Commons Attribution license CC-BY 4.0, code under BSD 3-Clause License © 2020 R.C. Cooper"
]
},
{
@@ -99,7 +99,7 @@
"cell_type": "markdown",
"metadata": {},
"source": [
- "## Exercise\n",
+ "## Exercise and Discussion\n",
"\n",
"Try generating more random numbers and plotting histograms of the results i.e. increase `10` to larger values. \n",
"\n",
@@ -323,6 +323,13 @@
"Why does the Monte Carlo method work? Is there a benefit to random numbers as opposed to using an ordered set divided into equally-spaced intervals?"
]
},
+ {
+ "cell_type": "code",
+ "execution_count": null,
+ "metadata": {},
+ "outputs": [],
+ "source": []
+ },
{
"cell_type": "markdown",
"metadata": {},
diff --git a/notebooks/01_Cheers_Stats_Beers.ipynb b/notebooks/01_Cheers_Stats_Beers.ipynb
index fa8752b..8d868a6 100644
--- a/notebooks/01_Cheers_Stats_Beers.ipynb
+++ b/notebooks/01_Cheers_Stats_Beers.ipynb
@@ -4,7 +4,7 @@
"cell_type": "markdown",
"metadata": {},
"source": [
- "###### Content under Creative Commons Attribution license CC-BY 4.0, code under BSD 3-Clause License © 2019 Ryan C. Cooper"
+ "###### Content modified under Creative Commons Attribution license CC-BY 4.0, code under BSD 3-Clause License © 2020 Ryan C. Cooper"
]
},
{
@@ -853,7 +853,11 @@
"\n",
"Why? This gets a little technical, but the reason is that if you have a _sample_ of the population, you don't know the _real_ value of the mean, and $\\bar{x}$ is actually an _estimate_ of the mean. That's why you'll often find the symbol $\\mu$ used to denote the population mean, and distinguish it with the sample mean, $\\bar{x}$. Using $\\bar{x}$ to compute the standard deviation introduces a small bias: $\\bar{x}$ is computed _from the sample values_, and the data are on average (slightly) closer to $\\bar{x}$ than the population is to $\\mu$. Dividing by $N-1$ instead of $N$ corrects this bias!\n",
"\n",
- "Prof. Sainani explains it by saying that we lost one degree of freedom when we estimated the mean using $\\bar{x}$. For example, say we have 100 people and I give you their mean age, and the actual age for 99 people from the sample: you'll be able to calculate the age of that 100th person. Once we calculated the mean, we only have 99 degrees of freedom left because that 100th person's age is fixed. "
+ "Prof. Sainani explains it by saying that we lost one degree of freedom when we estimated the mean using $\\bar{x}$. For example, say we have 100 people and I give you their mean age, and the actual age for 99 people from the sample: you'll be able to calculate the age of that 100th person. Once we calculated the mean, we only have 99 degrees of freedom left because that 100th person's age is fixed. \n",
+ "\n",
+ "Below is a graphical distinction between the _sample_ and the _population_ from [@allison_horst on twitter](https://twitter.com/allison_horst)\n",
+ "\n",
+ "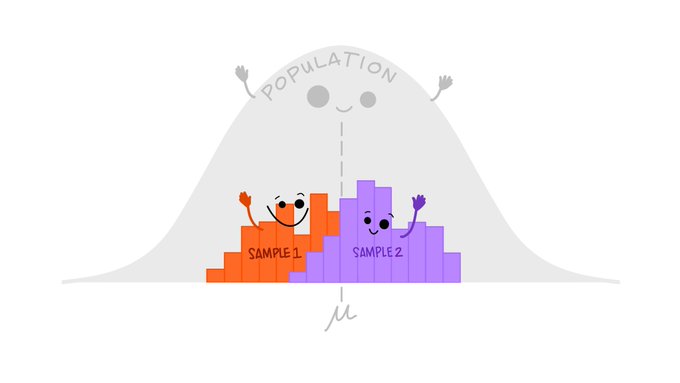\n"
]
},
{
diff --git a/notebooks/02_Seeing_Stats.ipynb b/notebooks/02_Seeing_Stats.ipynb
index 1193c0a..a25d3aa 100644
--- a/notebooks/02_Seeing_Stats.ipynb
+++ b/notebooks/02_Seeing_Stats.ipynb
@@ -4,7 +4,7 @@
"cell_type": "markdown",
"metadata": {},
"source": [
- "###### Modified under Creative Commons Attribution license CC-BY 4.0, code under BSD 3-Clause License © 2020 R.C. Cooper"
+ "###### Content modified under Creative Commons Attribution license CC-BY 4.0, code under BSD 3-Clause License © 2020 R.C. Cooper"
]
},
{
@@ -484,7 +484,7 @@
"##### Exercise:\n",
"\n",
"* Go to the documentation of [`np.var()`](https://docs.scipy.org/doc/numpy-1.13.0/reference/generated/numpy.var.html) and analyze if this function is computing the _sample variance_. \n",
- "**Hint**: Check what it says about the \"data degrees of freedom.\"\n",
+ "__Hint__: Check what it says about the \"data degrees of freedom.\"\n",
"\n",
"If you did the reading, you might have noticed that, by default, the argument `ddof` in `np.var()` is set to zero. If we use the default option, then we are not really calculating the sample variance. Recall from the previous lesson that the **sample variance** is:\n",
"\n",
diff --git a/notebooks/03_Linear_Regression_with_Real_Data.ipynb b/notebooks/03_Linear_Regression_with_Real_Data.ipynb
index 21438c1..4f9013c 100644
--- a/notebooks/03_Linear_Regression_with_Real_Data.ipynb
+++ b/notebooks/03_Linear_Regression_with_Real_Data.ipynb
@@ -4,7 +4,7 @@
"cell_type": "markdown",
"metadata": {},
"source": [
- "###### Modified under Creative Commons Attribution license CC-BY 4.0, code under BSD 3-Clause License © 2020 R.C. Cooper"
+ "###### Content modified under Creative Commons Attribution license CC-BY 4.0, code under BSD 3-Clause License © 2020 R.C. Cooper"
]
},
{
diff --git a/notebooks/04_Stats_and_Montecarlo.ipynb b/notebooks/04_Stats_and_Montecarlo.ipynb
index 7e22206..e24a004 100644
--- a/notebooks/04_Stats_and_Montecarlo.ipynb
+++ b/notebooks/04_Stats_and_Montecarlo.ipynb
@@ -4,7 +4,7 @@
"cell_type": "markdown",
"metadata": {},
"source": [
- "###### Created under Creative Commons Attribution license CC-BY 4.0, code under BSD 3-Clause License © 2020 R.C. Cooper"
+ "###### Content created under Creative Commons Attribution license CC-BY 4.0, code under BSD 3-Clause License © 2020 R.C. Cooper"
]
},
{
@@ -99,7 +99,7 @@
"cell_type": "markdown",
"metadata": {},
"source": [
- "## Exercise\n",
+ "## Exercise and Discussion\n",
"\n",
"Try generating more random numbers and plotting histograms of the results i.e. increase `10` to larger values. \n",
"\n",
@@ -323,6 +323,13 @@
"Why does the Monte Carlo method work? Is there a benefit to random numbers as opposed to using an ordered set divided into equally-spaced intervals?"
]
},
+ {
+ "cell_type": "code",
+ "execution_count": null,
+ "metadata": {},
+ "outputs": [],
+ "source": []
+ },
{
"cell_type": "markdown",
"metadata": {},
diff --git a/project/.ipynb_checkpoints/02_Analyze-data_project-checkpoint.ipynb b/project/.ipynb_checkpoints/02_Analyze-data_project-checkpoint.ipynb
index ce6abc8..9b149ff 100644
--- a/project/.ipynb_checkpoints/02_Analyze-data_project-checkpoint.ipynb
+++ b/project/.ipynb_checkpoints/02_Analyze-data_project-checkpoint.ipynb
@@ -4,9 +4,9 @@
"cell_type": "markdown",
"metadata": {},
"source": [
- "# Computational Mechanics Project #02 - Create specifications for a spitballing robot\n",
+ "# Computational Mechanics Project #02 - Create specifications for a projectile robot\n",
"\n",
- "On the first day of class, we threw $2\"\\times~2\"$ dampened paper (spitballs) at a target on the whiteboard. Now, we are going to analyze the accuracy of the class with some cool Python tools and design a robot that has the same accuracy and precision as the class. \n",
+ "On the first day of class, we threw $2\"\\times~2\"$ dampened paper (spitballs) at a target on the whiteboard. Now, we are going to analyze the accuracy of the class with some cool Python tools and design a robot that has the same accuracy and precision as the class, but we will have the robot move farther away from the target and use a simpler projectile i.e. a tennis ball so we don't need to worry about knuckle-ball physics. \n",
"\n",
"The goal of this project is to determine the precision of necessary components for a robot that can reproduce the class throwing distibution. We have generated pseudo random numbers using `numpy.random`, but the class target practice is an example of truly random distributions. If we repeated the exercise, there is a vanishingly small probability that we would hit the same points on the target, and there are no deterministic models that could take into account all of the factors that affected each hit on the board. \n",
"\n",
@@ -69,7 +69,7 @@
"source": [
"# Project Deliverables\n",
"\n",
- "1. Statistical analysis of class accuracy and precision (x- and z-locations)\n",
+ "1. Statistical analysis of class accuracy and precision (x- and z-locations) data is in the csv file [../data/target_data.csv](../data/target_data.csv) _Note: if you want to see how I turned the images into data check out the jupyter notebook [process_target_practice](./process_target_practice.ipynb)\n",
"\n",
"2. A Monte Carlo model to generate impact heights based upon uncertainty in $\\theta_0$ and $v_0$. \n",
"\n",
diff --git a/project/.ipynb_checkpoints/process_target_practice-checkpoint.ipynb b/project/.ipynb_checkpoints/process_target_practice-checkpoint.ipynb
index 55dacd5..ce8d8be 100644
--- a/project/.ipynb_checkpoints/process_target_practice-checkpoint.ipynb
+++ b/project/.ipynb_checkpoints/process_target_practice-checkpoint.ipynb
@@ -1,20 +1,1749 @@
{
"cells": [
+ {
+ "cell_type": "markdown",
+ "metadata": {},
+ "source": [
+ "# Creating quantitative data from an image\n",
+ "\n",
+ "In this _preview_ notebook, we will generate the location of board hits by clicking on an image displayed by matplotlib. We will repeat this process in the next Module_03-IVPs. \n",
+ "\n",
+ "The first step is to import the libraries we need, the new functions here are \n",
+ "\n",
+ "- `imread`: a function that creates a numpy array that can be plotted with plt.imshow\n",
+ "\n",
+ "- `%matplotlib notebook`: is a \"magic\" command that allows for an interactive matplotlib figure. There's one extra plotting step of creating a figure before plotting with `plt.figure()`"
+ ]
+ },
+ {
+ "cell_type": "code",
+ "execution_count": 6,
+ "metadata": {},
+ "outputs": [],
+ "source": [
+ "import matplotlib.pyplot as plt\n",
+ "from matplotlib.image import imread\n",
+ "import numpy as np\n",
+ "%matplotlib notebook"
+ ]
+ },
+ {
+ "cell_type": "markdown",
+ "metadata": {},
+ "source": [
+ "# Read in images target_01 and target_02\n",
+ "\n",
+ "Now we read in target_01.jpg and target_02.jpg into variables `im1` and `im2`. These are the images of the whiteboard. The black lines are perpendicular to each other and 36\" (0.9144 m) long. We'll display `im1` to start."
+ ]
+ },
+ {
+ "cell_type": "code",
+ "execution_count": 7,
+ "metadata": {},
+ "outputs": [
+ {
+ "data": {
+ "application/javascript": [
+ "/* Put everything inside the global mpl namespace */\n",
+ "window.mpl = {};\n",
+ "\n",
+ "\n",
+ "mpl.get_websocket_type = function() {\n",
+ " if (typeof(WebSocket) !== 'undefined') {\n",
+ " return WebSocket;\n",
+ " } else if (typeof(MozWebSocket) !== 'undefined') {\n",
+ " return MozWebSocket;\n",
+ " } else {\n",
+ " alert('Your browser does not have WebSocket support. ' +\n",
+ " 'Please try Chrome, Safari or Firefox ≥ 6. ' +\n",
+ " 'Firefox 4 and 5 are also supported but you ' +\n",
+ " 'have to enable WebSockets in about:config.');\n",
+ " };\n",
+ "}\n",
+ "\n",
+ "mpl.figure = function(figure_id, websocket, ondownload, parent_element) {\n",
+ " this.id = figure_id;\n",
+ "\n",
+ " this.ws = websocket;\n",
+ "\n",
+ " this.supports_binary = (this.ws.binaryType != undefined);\n",
+ "\n",
+ " if (!this.supports_binary) {\n",
+ " var warnings = document.getElementById(\"mpl-warnings\");\n",
+ " if (warnings) {\n",
+ " warnings.style.display = 'block';\n",
+ " warnings.textContent = (\n",
+ " \"This browser does not support binary websocket messages. \" +\n",
+ " \"Performance may be slow.\");\n",
+ " }\n",
+ " }\n",
+ "\n",
+ " this.imageObj = new Image();\n",
+ "\n",
+ " this.context = undefined;\n",
+ " this.message = undefined;\n",
+ " this.canvas = undefined;\n",
+ " this.rubberband_canvas = undefined;\n",
+ " this.rubberband_context = undefined;\n",
+ " this.format_dropdown = undefined;\n",
+ "\n",
+ " this.image_mode = 'full';\n",
+ "\n",
+ " this.root = $('
');\n",
+ " this._root_extra_style(this.root)\n",
+ " this.root.attr('style', 'display: inline-block');\n",
+ "\n",
+ " $(parent_element).append(this.root);\n",
+ "\n",
+ " this._init_header(this);\n",
+ " this._init_canvas(this);\n",
+ " this._init_toolbar(this);\n",
+ "\n",
+ " var fig = this;\n",
+ "\n",
+ " this.waiting = false;\n",
+ "\n",
+ " this.ws.onopen = function () {\n",
+ " fig.send_message(\"supports_binary\", {value: fig.supports_binary});\n",
+ " fig.send_message(\"send_image_mode\", {});\n",
+ " if (mpl.ratio != 1) {\n",
+ " fig.send_message(\"set_dpi_ratio\", {'dpi_ratio': mpl.ratio});\n",
+ " }\n",
+ " fig.send_message(\"refresh\", {});\n",
+ " }\n",
+ "\n",
+ " this.imageObj.onload = function() {\n",
+ " if (fig.image_mode == 'full') {\n",
+ " // Full images could contain transparency (where diff images\n",
+ " // almost always do), so we need to clear the canvas so that\n",
+ " // there is no ghosting.\n",
+ " fig.context.clearRect(0, 0, fig.canvas.width, fig.canvas.height);\n",
+ " }\n",
+ " fig.context.drawImage(fig.imageObj, 0, 0);\n",
+ " };\n",
+ "\n",
+ " this.imageObj.onunload = function() {\n",
+ " fig.ws.close();\n",
+ " }\n",
+ "\n",
+ " this.ws.onmessage = this._make_on_message_function(this);\n",
+ "\n",
+ " this.ondownload = ondownload;\n",
+ "}\n",
+ "\n",
+ "mpl.figure.prototype._init_header = function() {\n",
+ " var titlebar = $(\n",
+ " '');\n",
+ " var titletext = $(\n",
+ " '');\n",
+ " titlebar.append(titletext)\n",
+ " this.root.append(titlebar);\n",
+ " this.header = titletext[0];\n",
+ "}\n",
+ "\n",
+ "\n",
+ "\n",
+ "mpl.figure.prototype._canvas_extra_style = function(canvas_div) {\n",
+ "\n",
+ "}\n",
+ "\n",
+ "\n",
+ "mpl.figure.prototype._root_extra_style = function(canvas_div) {\n",
+ "\n",
+ "}\n",
+ "\n",
+ "mpl.figure.prototype._init_canvas = function() {\n",
+ " var fig = this;\n",
+ "\n",
+ " var canvas_div = $('');\n",
+ "\n",
+ " canvas_div.attr('style', 'position: relative; clear: both; outline: 0');\n",
+ "\n",
+ " function canvas_keyboard_event(event) {\n",
+ " return fig.key_event(event, event['data']);\n",
+ " }\n",
+ "\n",
+ " canvas_div.keydown('key_press', canvas_keyboard_event);\n",
+ " canvas_div.keyup('key_release', canvas_keyboard_event);\n",
+ " this.canvas_div = canvas_div\n",
+ " this._canvas_extra_style(canvas_div)\n",
+ " this.root.append(canvas_div);\n",
+ "\n",
+ " var canvas = $('');\n",
+ " canvas.addClass('mpl-canvas');\n",
+ " canvas.attr('style', \"left: 0; top: 0; z-index: 0; outline: 0\")\n",
+ "\n",
+ " this.canvas = canvas[0];\n",
+ " this.context = canvas[0].getContext(\"2d\");\n",
+ "\n",
+ " var backingStore = this.context.backingStorePixelRatio ||\n",
+ "\tthis.context.webkitBackingStorePixelRatio ||\n",
+ "\tthis.context.mozBackingStorePixelRatio ||\n",
+ "\tthis.context.msBackingStorePixelRatio ||\n",
+ "\tthis.context.oBackingStorePixelRatio ||\n",
+ "\tthis.context.backingStorePixelRatio || 1;\n",
+ "\n",
+ " mpl.ratio = (window.devicePixelRatio || 1) / backingStore;\n",
+ "\n",
+ " var rubberband = $('');\n",
+ " rubberband.attr('style', \"position: absolute; left: 0; top: 0; z-index: 1;\")\n",
+ "\n",
+ " var pass_mouse_events = true;\n",
+ "\n",
+ " canvas_div.resizable({\n",
+ " start: function(event, ui) {\n",
+ " pass_mouse_events = false;\n",
+ " },\n",
+ " resize: function(event, ui) {\n",
+ " fig.request_resize(ui.size.width, ui.size.height);\n",
+ " },\n",
+ " stop: function(event, ui) {\n",
+ " pass_mouse_events = true;\n",
+ " fig.request_resize(ui.size.width, ui.size.height);\n",
+ " },\n",
+ " });\n",
+ "\n",
+ " function mouse_event_fn(event) {\n",
+ " if (pass_mouse_events)\n",
+ " return fig.mouse_event(event, event['data']);\n",
+ " }\n",
+ "\n",
+ " rubberband.mousedown('button_press', mouse_event_fn);\n",
+ " rubberband.mouseup('button_release', mouse_event_fn);\n",
+ " // Throttle sequential mouse events to 1 every 20ms.\n",
+ " rubberband.mousemove('motion_notify', mouse_event_fn);\n",
+ "\n",
+ " rubberband.mouseenter('figure_enter', mouse_event_fn);\n",
+ " rubberband.mouseleave('figure_leave', mouse_event_fn);\n",
+ "\n",
+ " canvas_div.on(\"wheel\", function (event) {\n",
+ " event = event.originalEvent;\n",
+ " event['data'] = 'scroll'\n",
+ " if (event.deltaY < 0) {\n",
+ " event.step = 1;\n",
+ " } else {\n",
+ " event.step = -1;\n",
+ " }\n",
+ " mouse_event_fn(event);\n",
+ " });\n",
+ "\n",
+ " canvas_div.append(canvas);\n",
+ " canvas_div.append(rubberband);\n",
+ "\n",
+ " this.rubberband = rubberband;\n",
+ " this.rubberband_canvas = rubberband[0];\n",
+ " this.rubberband_context = rubberband[0].getContext(\"2d\");\n",
+ " this.rubberband_context.strokeStyle = \"#000000\";\n",
+ "\n",
+ " this._resize_canvas = function(width, height) {\n",
+ " // Keep the size of the canvas, canvas container, and rubber band\n",
+ " // canvas in synch.\n",
+ " canvas_div.css('width', width)\n",
+ " canvas_div.css('height', height)\n",
+ "\n",
+ " canvas.attr('width', width * mpl.ratio);\n",
+ " canvas.attr('height', height * mpl.ratio);\n",
+ " canvas.attr('style', 'width: ' + width + 'px; height: ' + height + 'px;');\n",
+ "\n",
+ " rubberband.attr('width', width);\n",
+ " rubberband.attr('height', height);\n",
+ " }\n",
+ "\n",
+ " // Set the figure to an initial 600x600px, this will subsequently be updated\n",
+ " // upon first draw.\n",
+ " this._resize_canvas(600, 600);\n",
+ "\n",
+ " // Disable right mouse context menu.\n",
+ " $(this.rubberband_canvas).bind(\"contextmenu\",function(e){\n",
+ " return false;\n",
+ " });\n",
+ "\n",
+ " function set_focus () {\n",
+ " canvas.focus();\n",
+ " canvas_div.focus();\n",
+ " }\n",
+ "\n",
+ " window.setTimeout(set_focus, 100);\n",
+ "}\n",
+ "\n",
+ "mpl.figure.prototype._init_toolbar = function() {\n",
+ " var fig = this;\n",
+ "\n",
+ " var nav_element = $('');\n",
+ " nav_element.attr('style', 'width: 100%');\n",
+ " this.root.append(nav_element);\n",
+ "\n",
+ " // Define a callback function for later on.\n",
+ " function toolbar_event(event) {\n",
+ " return fig.toolbar_button_onclick(event['data']);\n",
+ " }\n",
+ " function toolbar_mouse_event(event) {\n",
+ " return fig.toolbar_button_onmouseover(event['data']);\n",
+ " }\n",
+ "\n",
+ " for(var toolbar_ind in mpl.toolbar_items) {\n",
+ " var name = mpl.toolbar_items[toolbar_ind][0];\n",
+ " var tooltip = mpl.toolbar_items[toolbar_ind][1];\n",
+ " var image = mpl.toolbar_items[toolbar_ind][2];\n",
+ " var method_name = mpl.toolbar_items[toolbar_ind][3];\n",
+ "\n",
+ " if (!name) {\n",
+ " // put a spacer in here.\n",
+ " continue;\n",
+ " }\n",
+ " var button = $('');\n",
+ " button.addClass('ui-button ui-widget ui-state-default ui-corner-all ' +\n",
+ " 'ui-button-icon-only');\n",
+ " button.attr('role', 'button');\n",
+ " button.attr('aria-disabled', 'false');\n",
+ " button.click(method_name, toolbar_event);\n",
+ " button.mouseover(tooltip, toolbar_mouse_event);\n",
+ "\n",
+ " var icon_img = $('');\n",
+ " icon_img.addClass('ui-button-icon-primary ui-icon');\n",
+ " icon_img.addClass(image);\n",
+ " icon_img.addClass('ui-corner-all');\n",
+ "\n",
+ " var tooltip_span = $('');\n",
+ " tooltip_span.addClass('ui-button-text');\n",
+ " tooltip_span.html(tooltip);\n",
+ "\n",
+ " button.append(icon_img);\n",
+ " button.append(tooltip_span);\n",
+ "\n",
+ " nav_element.append(button);\n",
+ " }\n",
+ "\n",
+ " var fmt_picker_span = $('');\n",
+ "\n",
+ " var fmt_picker = $('');\n",
+ " fmt_picker.addClass('mpl-toolbar-option ui-widget ui-widget-content');\n",
+ " fmt_picker_span.append(fmt_picker);\n",
+ " nav_element.append(fmt_picker_span);\n",
+ " this.format_dropdown = fmt_picker[0];\n",
+ "\n",
+ " for (var ind in mpl.extensions) {\n",
+ " var fmt = mpl.extensions[ind];\n",
+ " var option = $(\n",
+ " '', {selected: fmt === mpl.default_extension}).html(fmt);\n",
+ " fmt_picker.append(option);\n",
+ " }\n",
+ "\n",
+ " // Add hover states to the ui-buttons\n",
+ " $( \".ui-button\" ).hover(\n",
+ " function() { $(this).addClass(\"ui-state-hover\");},\n",
+ " function() { $(this).removeClass(\"ui-state-hover\");}\n",
+ " );\n",
+ "\n",
+ " var status_bar = $('');\n",
+ " nav_element.append(status_bar);\n",
+ " this.message = status_bar[0];\n",
+ "}\n",
+ "\n",
+ "mpl.figure.prototype.request_resize = function(x_pixels, y_pixels) {\n",
+ " // Request matplotlib to resize the figure. Matplotlib will then trigger a resize in the client,\n",
+ " // which will in turn request a refresh of the image.\n",
+ " this.send_message('resize', {'width': x_pixels, 'height': y_pixels});\n",
+ "}\n",
+ "\n",
+ "mpl.figure.prototype.send_message = function(type, properties) {\n",
+ " properties['type'] = type;\n",
+ " properties['figure_id'] = this.id;\n",
+ " this.ws.send(JSON.stringify(properties));\n",
+ "}\n",
+ "\n",
+ "mpl.figure.prototype.send_draw_message = function() {\n",
+ " if (!this.waiting) {\n",
+ " this.waiting = true;\n",
+ " this.ws.send(JSON.stringify({type: \"draw\", figure_id: this.id}));\n",
+ " }\n",
+ "}\n",
+ "\n",
+ "\n",
+ "mpl.figure.prototype.handle_save = function(fig, msg) {\n",
+ " var format_dropdown = fig.format_dropdown;\n",
+ " var format = format_dropdown.options[format_dropdown.selectedIndex].value;\n",
+ " fig.ondownload(fig, format);\n",
+ "}\n",
+ "\n",
+ "\n",
+ "mpl.figure.prototype.handle_resize = function(fig, msg) {\n",
+ " var size = msg['size'];\n",
+ " if (size[0] != fig.canvas.width || size[1] != fig.canvas.height) {\n",
+ " fig._resize_canvas(size[0], size[1]);\n",
+ " fig.send_message(\"refresh\", {});\n",
+ " };\n",
+ "}\n",
+ "\n",
+ "mpl.figure.prototype.handle_rubberband = function(fig, msg) {\n",
+ " var x0 = msg['x0'] / mpl.ratio;\n",
+ " var y0 = (fig.canvas.height - msg['y0']) / mpl.ratio;\n",
+ " var x1 = msg['x1'] / mpl.ratio;\n",
+ " var y1 = (fig.canvas.height - msg['y1']) / mpl.ratio;\n",
+ " x0 = Math.floor(x0) + 0.5;\n",
+ " y0 = Math.floor(y0) + 0.5;\n",
+ " x1 = Math.floor(x1) + 0.5;\n",
+ " y1 = Math.floor(y1) + 0.5;\n",
+ " var min_x = Math.min(x0, x1);\n",
+ " var min_y = Math.min(y0, y1);\n",
+ " var width = Math.abs(x1 - x0);\n",
+ " var height = Math.abs(y1 - y0);\n",
+ "\n",
+ " fig.rubberband_context.clearRect(\n",
+ " 0, 0, fig.canvas.width / mpl.ratio, fig.canvas.height / mpl.ratio);\n",
+ "\n",
+ " fig.rubberband_context.strokeRect(min_x, min_y, width, height);\n",
+ "}\n",
+ "\n",
+ "mpl.figure.prototype.handle_figure_label = function(fig, msg) {\n",
+ " // Updates the figure title.\n",
+ " fig.header.textContent = msg['label'];\n",
+ "}\n",
+ "\n",
+ "mpl.figure.prototype.handle_cursor = function(fig, msg) {\n",
+ " var cursor = msg['cursor'];\n",
+ " switch(cursor)\n",
+ " {\n",
+ " case 0:\n",
+ " cursor = 'pointer';\n",
+ " break;\n",
+ " case 1:\n",
+ " cursor = 'default';\n",
+ " break;\n",
+ " case 2:\n",
+ " cursor = 'crosshair';\n",
+ " break;\n",
+ " case 3:\n",
+ " cursor = 'move';\n",
+ " break;\n",
+ " }\n",
+ " fig.rubberband_canvas.style.cursor = cursor;\n",
+ "}\n",
+ "\n",
+ "mpl.figure.prototype.handle_message = function(fig, msg) {\n",
+ " fig.message.textContent = msg['message'];\n",
+ "}\n",
+ "\n",
+ "mpl.figure.prototype.handle_draw = function(fig, msg) {\n",
+ " // Request the server to send over a new figure.\n",
+ " fig.send_draw_message();\n",
+ "}\n",
+ "\n",
+ "mpl.figure.prototype.handle_image_mode = function(fig, msg) {\n",
+ " fig.image_mode = msg['mode'];\n",
+ "}\n",
+ "\n",
+ "mpl.figure.prototype.updated_canvas_event = function() {\n",
+ " // Called whenever the canvas gets updated.\n",
+ " this.send_message(\"ack\", {});\n",
+ "}\n",
+ "\n",
+ "// A function to construct a web socket function for onmessage handling.\n",
+ "// Called in the figure constructor.\n",
+ "mpl.figure.prototype._make_on_message_function = function(fig) {\n",
+ " return function socket_on_message(evt) {\n",
+ " if (evt.data instanceof Blob) {\n",
+ " /* FIXME: We get \"Resource interpreted as Image but\n",
+ " * transferred with MIME type text/plain:\" errors on\n",
+ " * Chrome. But how to set the MIME type? It doesn't seem\n",
+ " * to be part of the websocket stream */\n",
+ " evt.data.type = \"image/png\";\n",
+ "\n",
+ " /* Free the memory for the previous frames */\n",
+ " if (fig.imageObj.src) {\n",
+ " (window.URL || window.webkitURL).revokeObjectURL(\n",
+ " fig.imageObj.src);\n",
+ " }\n",
+ "\n",
+ " fig.imageObj.src = (window.URL || window.webkitURL).createObjectURL(\n",
+ " evt.data);\n",
+ " fig.updated_canvas_event();\n",
+ " fig.waiting = false;\n",
+ " return;\n",
+ " }\n",
+ " else if (typeof evt.data === 'string' && evt.data.slice(0, 21) == \"data:image/png;base64\") {\n",
+ " fig.imageObj.src = evt.data;\n",
+ " fig.updated_canvas_event();\n",
+ " fig.waiting = false;\n",
+ " return;\n",
+ " }\n",
+ "\n",
+ " var msg = JSON.parse(evt.data);\n",
+ " var msg_type = msg['type'];\n",
+ "\n",
+ " // Call the \"handle_{type}\" callback, which takes\n",
+ " // the figure and JSON message as its only arguments.\n",
+ " try {\n",
+ " var callback = fig[\"handle_\" + msg_type];\n",
+ " } catch (e) {\n",
+ " console.log(\"No handler for the '\" + msg_type + \"' message type: \", msg);\n",
+ " return;\n",
+ " }\n",
+ "\n",
+ " if (callback) {\n",
+ " try {\n",
+ " // console.log(\"Handling '\" + msg_type + \"' message: \", msg);\n",
+ " callback(fig, msg);\n",
+ " } catch (e) {\n",
+ " console.log(\"Exception inside the 'handler_\" + msg_type + \"' callback:\", e, e.stack, msg);\n",
+ " }\n",
+ " }\n",
+ " };\n",
+ "}\n",
+ "\n",
+ "// from http://stackoverflow.com/questions/1114465/getting-mouse-location-in-canvas\n",
+ "mpl.findpos = function(e) {\n",
+ " //this section is from http://www.quirksmode.org/js/events_properties.html\n",
+ " var targ;\n",
+ " if (!e)\n",
+ " e = window.event;\n",
+ " if (e.target)\n",
+ " targ = e.target;\n",
+ " else if (e.srcElement)\n",
+ " targ = e.srcElement;\n",
+ " if (targ.nodeType == 3) // defeat Safari bug\n",
+ " targ = targ.parentNode;\n",
+ "\n",
+ " // jQuery normalizes the pageX and pageY\n",
+ " // pageX,Y are the mouse positions relative to the document\n",
+ " // offset() returns the position of the element relative to the document\n",
+ " var x = e.pageX - $(targ).offset().left;\n",
+ " var y = e.pageY - $(targ).offset().top;\n",
+ "\n",
+ " return {\"x\": x, \"y\": y};\n",
+ "};\n",
+ "\n",
+ "/*\n",
+ " * return a copy of an object with only non-object keys\n",
+ " * we need this to avoid circular references\n",
+ " * http://stackoverflow.com/a/24161582/3208463\n",
+ " */\n",
+ "function simpleKeys (original) {\n",
+ " return Object.keys(original).reduce(function (obj, key) {\n",
+ " if (typeof original[key] !== 'object')\n",
+ " obj[key] = original[key]\n",
+ " return obj;\n",
+ " }, {});\n",
+ "}\n",
+ "\n",
+ "mpl.figure.prototype.mouse_event = function(event, name) {\n",
+ " var canvas_pos = mpl.findpos(event)\n",
+ "\n",
+ " if (name === 'button_press')\n",
+ " {\n",
+ " this.canvas.focus();\n",
+ " this.canvas_div.focus();\n",
+ " }\n",
+ "\n",
+ " var x = canvas_pos.x * mpl.ratio;\n",
+ " var y = canvas_pos.y * mpl.ratio;\n",
+ "\n",
+ " this.send_message(name, {x: x, y: y, button: event.button,\n",
+ " step: event.step,\n",
+ " guiEvent: simpleKeys(event)});\n",
+ "\n",
+ " /* This prevents the web browser from automatically changing to\n",
+ " * the text insertion cursor when the button is pressed. We want\n",
+ " * to control all of the cursor setting manually through the\n",
+ " * 'cursor' event from matplotlib */\n",
+ " event.preventDefault();\n",
+ " return false;\n",
+ "}\n",
+ "\n",
+ "mpl.figure.prototype._key_event_extra = function(event, name) {\n",
+ " // Handle any extra behaviour associated with a key event\n",
+ "}\n",
+ "\n",
+ "mpl.figure.prototype.key_event = function(event, name) {\n",
+ "\n",
+ " // Prevent repeat events\n",
+ " if (name == 'key_press')\n",
+ " {\n",
+ " if (event.which === this._key)\n",
+ " return;\n",
+ " else\n",
+ " this._key = event.which;\n",
+ " }\n",
+ " if (name == 'key_release')\n",
+ " this._key = null;\n",
+ "\n",
+ " var value = '';\n",
+ " if (event.ctrlKey && event.which != 17)\n",
+ " value += \"ctrl+\";\n",
+ " if (event.altKey && event.which != 18)\n",
+ " value += \"alt+\";\n",
+ " if (event.shiftKey && event.which != 16)\n",
+ " value += \"shift+\";\n",
+ "\n",
+ " value += 'k';\n",
+ " value += event.which.toString();\n",
+ "\n",
+ " this._key_event_extra(event, name);\n",
+ "\n",
+ " this.send_message(name, {key: value,\n",
+ " guiEvent: simpleKeys(event)});\n",
+ " return false;\n",
+ "}\n",
+ "\n",
+ "mpl.figure.prototype.toolbar_button_onclick = function(name) {\n",
+ " if (name == 'download') {\n",
+ " this.handle_save(this, null);\n",
+ " } else {\n",
+ " this.send_message(\"toolbar_button\", {name: name});\n",
+ " }\n",
+ "};\n",
+ "\n",
+ "mpl.figure.prototype.toolbar_button_onmouseover = function(tooltip) {\n",
+ " this.message.textContent = tooltip;\n",
+ "};\n",
+ "mpl.toolbar_items = [[\"Home\", \"Reset original view\", \"fa fa-home icon-home\", \"home\"], [\"Back\", \"Back to previous view\", \"fa fa-arrow-left icon-arrow-left\", \"back\"], [\"Forward\", \"Forward to next view\", \"fa fa-arrow-right icon-arrow-right\", \"forward\"], [\"\", \"\", \"\", \"\"], [\"Pan\", \"Pan axes with left mouse, zoom with right\", \"fa fa-arrows icon-move\", \"pan\"], [\"Zoom\", \"Zoom to rectangle\", \"fa fa-square-o icon-check-empty\", \"zoom\"], [\"\", \"\", \"\", \"\"], [\"Download\", \"Download plot\", \"fa fa-floppy-o icon-save\", \"download\"]];\n",
+ "\n",
+ "mpl.extensions = [\"eps\", \"jpeg\", \"pdf\", \"png\", \"ps\", \"raw\", \"svg\", \"tif\"];\n",
+ "\n",
+ "mpl.default_extension = \"png\";var comm_websocket_adapter = function(comm) {\n",
+ " // Create a \"websocket\"-like object which calls the given IPython comm\n",
+ " // object with the appropriate methods. Currently this is a non binary\n",
+ " // socket, so there is still some room for performance tuning.\n",
+ " var ws = {};\n",
+ "\n",
+ " ws.close = function() {\n",
+ " comm.close()\n",
+ " };\n",
+ " ws.send = function(m) {\n",
+ " //console.log('sending', m);\n",
+ " comm.send(m);\n",
+ " };\n",
+ " // Register the callback with on_msg.\n",
+ " comm.on_msg(function(msg) {\n",
+ " //console.log('receiving', msg['content']['data'], msg);\n",
+ " // Pass the mpl event to the overridden (by mpl) onmessage function.\n",
+ " ws.onmessage(msg['content']['data'])\n",
+ " });\n",
+ " return ws;\n",
+ "}\n",
+ "\n",
+ "mpl.mpl_figure_comm = function(comm, msg) {\n",
+ " // This is the function which gets called when the mpl process\n",
+ " // starts-up an IPython Comm through the \"matplotlib\" channel.\n",
+ "\n",
+ " var id = msg.content.data.id;\n",
+ " // Get hold of the div created by the display call when the Comm\n",
+ " // socket was opened in Python.\n",
+ " var element = $(\"#\" + id);\n",
+ " var ws_proxy = comm_websocket_adapter(comm)\n",
+ "\n",
+ " function ondownload(figure, format) {\n",
+ " window.open(figure.imageObj.src);\n",
+ " }\n",
+ "\n",
+ " var fig = new mpl.figure(id, ws_proxy,\n",
+ " ondownload,\n",
+ " element.get(0));\n",
+ "\n",
+ " // Call onopen now - mpl needs it, as it is assuming we've passed it a real\n",
+ " // web socket which is closed, not our websocket->open comm proxy.\n",
+ " ws_proxy.onopen();\n",
+ "\n",
+ " fig.parent_element = element.get(0);\n",
+ " fig.cell_info = mpl.find_output_cell(\"\");\n",
+ " if (!fig.cell_info) {\n",
+ " console.error(\"Failed to find cell for figure\", id, fig);\n",
+ " return;\n",
+ " }\n",
+ "\n",
+ " var output_index = fig.cell_info[2]\n",
+ " var cell = fig.cell_info[0];\n",
+ "\n",
+ "};\n",
+ "\n",
+ "mpl.figure.prototype.handle_close = function(fig, msg) {\n",
+ " var width = fig.canvas.width/mpl.ratio\n",
+ " fig.root.unbind('remove')\n",
+ "\n",
+ " // Update the output cell to use the data from the current canvas.\n",
+ " fig.push_to_output();\n",
+ " var dataURL = fig.canvas.toDataURL();\n",
+ " // Re-enable the keyboard manager in IPython - without this line, in FF,\n",
+ " // the notebook keyboard shortcuts fail.\n",
+ " IPython.keyboard_manager.enable()\n",
+ " $(fig.parent_element).html('
');\n",
+ " fig.close_ws(fig, msg);\n",
+ "}\n",
+ "\n",
+ "mpl.figure.prototype.close_ws = function(fig, msg){\n",
+ " fig.send_message('closing', msg);\n",
+ " // fig.ws.close()\n",
+ "}\n",
+ "\n",
+ "mpl.figure.prototype.push_to_output = function(remove_interactive) {\n",
+ " // Turn the data on the canvas into data in the output cell.\n",
+ " var width = this.canvas.width/mpl.ratio\n",
+ " var dataURL = this.canvas.toDataURL();\n",
+ " this.cell_info[1]['text/html'] = '
';\n",
+ "}\n",
+ "\n",
+ "mpl.figure.prototype.updated_canvas_event = function() {\n",
+ " // Tell IPython that the notebook contents must change.\n",
+ " IPython.notebook.set_dirty(true);\n",
+ " this.send_message(\"ack\", {});\n",
+ " var fig = this;\n",
+ " // Wait a second, then push the new image to the DOM so\n",
+ " // that it is saved nicely (might be nice to debounce this).\n",
+ " setTimeout(function () { fig.push_to_output() }, 1000);\n",
+ "}\n",
+ "\n",
+ "mpl.figure.prototype._init_toolbar = function() {\n",
+ " var fig = this;\n",
+ "\n",
+ " var nav_element = $('');\n",
+ " nav_element.attr('style', 'width: 100%');\n",
+ " this.root.append(nav_element);\n",
+ "\n",
+ " // Define a callback function for later on.\n",
+ " function toolbar_event(event) {\n",
+ " return fig.toolbar_button_onclick(event['data']);\n",
+ " }\n",
+ " function toolbar_mouse_event(event) {\n",
+ " return fig.toolbar_button_onmouseover(event['data']);\n",
+ " }\n",
+ "\n",
+ " for(var toolbar_ind in mpl.toolbar_items){\n",
+ " var name = mpl.toolbar_items[toolbar_ind][0];\n",
+ " var tooltip = mpl.toolbar_items[toolbar_ind][1];\n",
+ " var image = mpl.toolbar_items[toolbar_ind][2];\n",
+ " var method_name = mpl.toolbar_items[toolbar_ind][3];\n",
+ "\n",
+ " if (!name) { continue; };\n",
+ "\n",
+ " var button = $('');\n",
+ " button.click(method_name, toolbar_event);\n",
+ " button.mouseover(tooltip, toolbar_mouse_event);\n",
+ " nav_element.append(button);\n",
+ " }\n",
+ "\n",
+ " // Add the status bar.\n",
+ " var status_bar = $('');\n",
+ " nav_element.append(status_bar);\n",
+ " this.message = status_bar[0];\n",
+ "\n",
+ " // Add the close button to the window.\n",
+ " var buttongrp = $('');\n",
+ " var button = $('');\n",
+ " button.click(function (evt) { fig.handle_close(fig, {}); } );\n",
+ " button.mouseover('Stop Interaction', toolbar_mouse_event);\n",
+ " buttongrp.append(button);\n",
+ " var titlebar = this.root.find($('.ui-dialog-titlebar'));\n",
+ " titlebar.prepend(buttongrp);\n",
+ "}\n",
+ "\n",
+ "mpl.figure.prototype._root_extra_style = function(el){\n",
+ " var fig = this\n",
+ " el.on(\"remove\", function(){\n",
+ "\tfig.close_ws(fig, {});\n",
+ " });\n",
+ "}\n",
+ "\n",
+ "mpl.figure.prototype._canvas_extra_style = function(el){\n",
+ " // this is important to make the div 'focusable\n",
+ " el.attr('tabindex', 0)\n",
+ " // reach out to IPython and tell the keyboard manager to turn it's self\n",
+ " // off when our div gets focus\n",
+ "\n",
+ " // location in version 3\n",
+ " if (IPython.notebook.keyboard_manager) {\n",
+ " IPython.notebook.keyboard_manager.register_events(el);\n",
+ " }\n",
+ " else {\n",
+ " // location in version 2\n",
+ " IPython.keyboard_manager.register_events(el);\n",
+ " }\n",
+ "\n",
+ "}\n",
+ "\n",
+ "mpl.figure.prototype._key_event_extra = function(event, name) {\n",
+ " var manager = IPython.notebook.keyboard_manager;\n",
+ " if (!manager)\n",
+ " manager = IPython.keyboard_manager;\n",
+ "\n",
+ " // Check for shift+enter\n",
+ " if (event.shiftKey && event.which == 13) {\n",
+ " this.canvas_div.blur();\n",
+ " event.shiftKey = false;\n",
+ " // Send a \"J\" for go to next cell\n",
+ " event.which = 74;\n",
+ " event.keyCode = 74;\n",
+ " manager.command_mode();\n",
+ " manager.handle_keydown(event);\n",
+ " }\n",
+ "}\n",
+ "\n",
+ "mpl.figure.prototype.handle_save = function(fig, msg) {\n",
+ " fig.ondownload(fig, null);\n",
+ "}\n",
+ "\n",
+ "\n",
+ "mpl.find_output_cell = function(html_output) {\n",
+ " // Return the cell and output element which can be found *uniquely* in the notebook.\n",
+ " // Note - this is a bit hacky, but it is done because the \"notebook_saving.Notebook\"\n",
+ " // IPython event is triggered only after the cells have been serialised, which for\n",
+ " // our purposes (turning an active figure into a static one), is too late.\n",
+ " var cells = IPython.notebook.get_cells();\n",
+ " var ncells = cells.length;\n",
+ " for (var i=0; i= 3 moved mimebundle to data attribute of output\n",
+ " data = data.data;\n",
+ " }\n",
+ " if (data['text/html'] == html_output) {\n",
+ " return [cell, data, j];\n",
+ " }\n",
+ " }\n",
+ " }\n",
+ " }\n",
+ "}\n",
+ "\n",
+ "// Register the function which deals with the matplotlib target/channel.\n",
+ "// The kernel may be null if the page has been refreshed.\n",
+ "if (IPython.notebook.kernel != null) {\n",
+ " IPython.notebook.kernel.comm_manager.register_target('matplotlib', mpl.mpl_figure_comm);\n",
+ "}\n"
+ ],
+ "text/plain": [
+ ""
+ ]
+ },
+ "metadata": {},
+ "output_type": "display_data"
+ },
+ {
+ "data": {
+ "text/html": [
+ "
"
+ ],
+ "text/plain": [
+ ""
+ ]
+ },
+ "metadata": {},
+ "output_type": "display_data"
+ },
+ {
+ "data": {
+ "text/plain": [
+ ""
+ ]
+ },
+ "execution_count": 7,
+ "metadata": {},
+ "output_type": "execute_result"
+ }
+ ],
+ "source": [
+ "im1 = imread('../data/target_01.jpg')\n",
+ "im2 = imread('../data/target_02.jpg')\n",
+ "plt.figure()\n",
+ "plt.imshow(im1)\n"
+ ]
+ },
+ {
+ "cell_type": "markdown",
+ "metadata": {},
+ "source": [
+ "### Capture mouse clicks on the frame\n",
+ "\n",
+ "Okay! Here is where things get really interesting. Matplotlib has the ability to create [event connections](https://matplotlib.org/devdocs/users/event_handling.html?highlight=mpl_connect), that is, connect the figure canvas to user-interface events on it, like mouse clicks. \n",
+ "\n",
+ "To use this ability, you write a function with the events you want to capture, and then connect this function to the Matplotlib \"event manager\" using [`mpl_connect()`](https://matplotlib.org/devdocs/api/backend_bases_api.html#matplotlib.backend_bases.FigureCanvasBase.mpl_connect). In this case, we connect the `'button_press_event'` to the function named `onclick()`, which captures the $(x,y)$ coordinates of the mouse click on the figure. Magic!"
+ ]
+ },
+ {
+ "cell_type": "code",
+ "execution_count": 14,
+ "metadata": {},
+ "outputs": [],
+ "source": [
+ "def onclick(event):\n",
+ " '''Capture the x,y coordinates of a mouse click on the image'''\n",
+ " ix, iy = event.xdata, event.ydata\n",
+ " coords.append([ix, iy]) "
+ ]
+ },
+ {
+ "cell_type": "code",
+ "execution_count": 15,
+ "metadata": {},
+ "outputs": [
+ {
+ "data": {
+ "application/javascript": [
+ "/* Put everything inside the global mpl namespace */\n",
+ "window.mpl = {};\n",
+ "\n",
+ "\n",
+ "mpl.get_websocket_type = function() {\n",
+ " if (typeof(WebSocket) !== 'undefined') {\n",
+ " return WebSocket;\n",
+ " } else if (typeof(MozWebSocket) !== 'undefined') {\n",
+ " return MozWebSocket;\n",
+ " } else {\n",
+ " alert('Your browser does not have WebSocket support. ' +\n",
+ " 'Please try Chrome, Safari or Firefox ≥ 6. ' +\n",
+ " 'Firefox 4 and 5 are also supported but you ' +\n",
+ " 'have to enable WebSockets in about:config.');\n",
+ " };\n",
+ "}\n",
+ "\n",
+ "mpl.figure = function(figure_id, websocket, ondownload, parent_element) {\n",
+ " this.id = figure_id;\n",
+ "\n",
+ " this.ws = websocket;\n",
+ "\n",
+ " this.supports_binary = (this.ws.binaryType != undefined);\n",
+ "\n",
+ " if (!this.supports_binary) {\n",
+ " var warnings = document.getElementById(\"mpl-warnings\");\n",
+ " if (warnings) {\n",
+ " warnings.style.display = 'block';\n",
+ " warnings.textContent = (\n",
+ " \"This browser does not support binary websocket messages. \" +\n",
+ " \"Performance may be slow.\");\n",
+ " }\n",
+ " }\n",
+ "\n",
+ " this.imageObj = new Image();\n",
+ "\n",
+ " this.context = undefined;\n",
+ " this.message = undefined;\n",
+ " this.canvas = undefined;\n",
+ " this.rubberband_canvas = undefined;\n",
+ " this.rubberband_context = undefined;\n",
+ " this.format_dropdown = undefined;\n",
+ "\n",
+ " this.image_mode = 'full';\n",
+ "\n",
+ " this.root = $('');\n",
+ " this._root_extra_style(this.root)\n",
+ " this.root.attr('style', 'display: inline-block');\n",
+ "\n",
+ " $(parent_element).append(this.root);\n",
+ "\n",
+ " this._init_header(this);\n",
+ " this._init_canvas(this);\n",
+ " this._init_toolbar(this);\n",
+ "\n",
+ " var fig = this;\n",
+ "\n",
+ " this.waiting = false;\n",
+ "\n",
+ " this.ws.onopen = function () {\n",
+ " fig.send_message(\"supports_binary\", {value: fig.supports_binary});\n",
+ " fig.send_message(\"send_image_mode\", {});\n",
+ " if (mpl.ratio != 1) {\n",
+ " fig.send_message(\"set_dpi_ratio\", {'dpi_ratio': mpl.ratio});\n",
+ " }\n",
+ " fig.send_message(\"refresh\", {});\n",
+ " }\n",
+ "\n",
+ " this.imageObj.onload = function() {\n",
+ " if (fig.image_mode == 'full') {\n",
+ " // Full images could contain transparency (where diff images\n",
+ " // almost always do), so we need to clear the canvas so that\n",
+ " // there is no ghosting.\n",
+ " fig.context.clearRect(0, 0, fig.canvas.width, fig.canvas.height);\n",
+ " }\n",
+ " fig.context.drawImage(fig.imageObj, 0, 0);\n",
+ " };\n",
+ "\n",
+ " this.imageObj.onunload = function() {\n",
+ " fig.ws.close();\n",
+ " }\n",
+ "\n",
+ " this.ws.onmessage = this._make_on_message_function(this);\n",
+ "\n",
+ " this.ondownload = ondownload;\n",
+ "}\n",
+ "\n",
+ "mpl.figure.prototype._init_header = function() {\n",
+ " var titlebar = $(\n",
+ " '');\n",
+ " var titletext = $(\n",
+ " '');\n",
+ " titlebar.append(titletext)\n",
+ " this.root.append(titlebar);\n",
+ " this.header = titletext[0];\n",
+ "}\n",
+ "\n",
+ "\n",
+ "\n",
+ "mpl.figure.prototype._canvas_extra_style = function(canvas_div) {\n",
+ "\n",
+ "}\n",
+ "\n",
+ "\n",
+ "mpl.figure.prototype._root_extra_style = function(canvas_div) {\n",
+ "\n",
+ "}\n",
+ "\n",
+ "mpl.figure.prototype._init_canvas = function() {\n",
+ " var fig = this;\n",
+ "\n",
+ " var canvas_div = $('');\n",
+ "\n",
+ " canvas_div.attr('style', 'position: relative; clear: both; outline: 0');\n",
+ "\n",
+ " function canvas_keyboard_event(event) {\n",
+ " return fig.key_event(event, event['data']);\n",
+ " }\n",
+ "\n",
+ " canvas_div.keydown('key_press', canvas_keyboard_event);\n",
+ " canvas_div.keyup('key_release', canvas_keyboard_event);\n",
+ " this.canvas_div = canvas_div\n",
+ " this._canvas_extra_style(canvas_div)\n",
+ " this.root.append(canvas_div);\n",
+ "\n",
+ " var canvas = $('');\n",
+ " canvas.addClass('mpl-canvas');\n",
+ " canvas.attr('style', \"left: 0; top: 0; z-index: 0; outline: 0\")\n",
+ "\n",
+ " this.canvas = canvas[0];\n",
+ " this.context = canvas[0].getContext(\"2d\");\n",
+ "\n",
+ " var backingStore = this.context.backingStorePixelRatio ||\n",
+ "\tthis.context.webkitBackingStorePixelRatio ||\n",
+ "\tthis.context.mozBackingStorePixelRatio ||\n",
+ "\tthis.context.msBackingStorePixelRatio ||\n",
+ "\tthis.context.oBackingStorePixelRatio ||\n",
+ "\tthis.context.backingStorePixelRatio || 1;\n",
+ "\n",
+ " mpl.ratio = (window.devicePixelRatio || 1) / backingStore;\n",
+ "\n",
+ " var rubberband = $('');\n",
+ " rubberband.attr('style', \"position: absolute; left: 0; top: 0; z-index: 1;\")\n",
+ "\n",
+ " var pass_mouse_events = true;\n",
+ "\n",
+ " canvas_div.resizable({\n",
+ " start: function(event, ui) {\n",
+ " pass_mouse_events = false;\n",
+ " },\n",
+ " resize: function(event, ui) {\n",
+ " fig.request_resize(ui.size.width, ui.size.height);\n",
+ " },\n",
+ " stop: function(event, ui) {\n",
+ " pass_mouse_events = true;\n",
+ " fig.request_resize(ui.size.width, ui.size.height);\n",
+ " },\n",
+ " });\n",
+ "\n",
+ " function mouse_event_fn(event) {\n",
+ " if (pass_mouse_events)\n",
+ " return fig.mouse_event(event, event['data']);\n",
+ " }\n",
+ "\n",
+ " rubberband.mousedown('button_press', mouse_event_fn);\n",
+ " rubberband.mouseup('button_release', mouse_event_fn);\n",
+ " // Throttle sequential mouse events to 1 every 20ms.\n",
+ " rubberband.mousemove('motion_notify', mouse_event_fn);\n",
+ "\n",
+ " rubberband.mouseenter('figure_enter', mouse_event_fn);\n",
+ " rubberband.mouseleave('figure_leave', mouse_event_fn);\n",
+ "\n",
+ " canvas_div.on(\"wheel\", function (event) {\n",
+ " event = event.originalEvent;\n",
+ " event['data'] = 'scroll'\n",
+ " if (event.deltaY < 0) {\n",
+ " event.step = 1;\n",
+ " } else {\n",
+ " event.step = -1;\n",
+ " }\n",
+ " mouse_event_fn(event);\n",
+ " });\n",
+ "\n",
+ " canvas_div.append(canvas);\n",
+ " canvas_div.append(rubberband);\n",
+ "\n",
+ " this.rubberband = rubberband;\n",
+ " this.rubberband_canvas = rubberband[0];\n",
+ " this.rubberband_context = rubberband[0].getContext(\"2d\");\n",
+ " this.rubberband_context.strokeStyle = \"#000000\";\n",
+ "\n",
+ " this._resize_canvas = function(width, height) {\n",
+ " // Keep the size of the canvas, canvas container, and rubber band\n",
+ " // canvas in synch.\n",
+ " canvas_div.css('width', width)\n",
+ " canvas_div.css('height', height)\n",
+ "\n",
+ " canvas.attr('width', width * mpl.ratio);\n",
+ " canvas.attr('height', height * mpl.ratio);\n",
+ " canvas.attr('style', 'width: ' + width + 'px; height: ' + height + 'px;');\n",
+ "\n",
+ " rubberband.attr('width', width);\n",
+ " rubberband.attr('height', height);\n",
+ " }\n",
+ "\n",
+ " // Set the figure to an initial 600x600px, this will subsequently be updated\n",
+ " // upon first draw.\n",
+ " this._resize_canvas(600, 600);\n",
+ "\n",
+ " // Disable right mouse context menu.\n",
+ " $(this.rubberband_canvas).bind(\"contextmenu\",function(e){\n",
+ " return false;\n",
+ " });\n",
+ "\n",
+ " function set_focus () {\n",
+ " canvas.focus();\n",
+ " canvas_div.focus();\n",
+ " }\n",
+ "\n",
+ " window.setTimeout(set_focus, 100);\n",
+ "}\n",
+ "\n",
+ "mpl.figure.prototype._init_toolbar = function() {\n",
+ " var fig = this;\n",
+ "\n",
+ " var nav_element = $('');\n",
+ " nav_element.attr('style', 'width: 100%');\n",
+ " this.root.append(nav_element);\n",
+ "\n",
+ " // Define a callback function for later on.\n",
+ " function toolbar_event(event) {\n",
+ " return fig.toolbar_button_onclick(event['data']);\n",
+ " }\n",
+ " function toolbar_mouse_event(event) {\n",
+ " return fig.toolbar_button_onmouseover(event['data']);\n",
+ " }\n",
+ "\n",
+ " for(var toolbar_ind in mpl.toolbar_items) {\n",
+ " var name = mpl.toolbar_items[toolbar_ind][0];\n",
+ " var tooltip = mpl.toolbar_items[toolbar_ind][1];\n",
+ " var image = mpl.toolbar_items[toolbar_ind][2];\n",
+ " var method_name = mpl.toolbar_items[toolbar_ind][3];\n",
+ "\n",
+ " if (!name) {\n",
+ " // put a spacer in here.\n",
+ " continue;\n",
+ " }\n",
+ " var button = $('');\n",
+ " button.addClass('ui-button ui-widget ui-state-default ui-corner-all ' +\n",
+ " 'ui-button-icon-only');\n",
+ " button.attr('role', 'button');\n",
+ " button.attr('aria-disabled', 'false');\n",
+ " button.click(method_name, toolbar_event);\n",
+ " button.mouseover(tooltip, toolbar_mouse_event);\n",
+ "\n",
+ " var icon_img = $('');\n",
+ " icon_img.addClass('ui-button-icon-primary ui-icon');\n",
+ " icon_img.addClass(image);\n",
+ " icon_img.addClass('ui-corner-all');\n",
+ "\n",
+ " var tooltip_span = $('');\n",
+ " tooltip_span.addClass('ui-button-text');\n",
+ " tooltip_span.html(tooltip);\n",
+ "\n",
+ " button.append(icon_img);\n",
+ " button.append(tooltip_span);\n",
+ "\n",
+ " nav_element.append(button);\n",
+ " }\n",
+ "\n",
+ " var fmt_picker_span = $('');\n",
+ "\n",
+ " var fmt_picker = $('');\n",
+ " fmt_picker.addClass('mpl-toolbar-option ui-widget ui-widget-content');\n",
+ " fmt_picker_span.append(fmt_picker);\n",
+ " nav_element.append(fmt_picker_span);\n",
+ " this.format_dropdown = fmt_picker[0];\n",
+ "\n",
+ " for (var ind in mpl.extensions) {\n",
+ " var fmt = mpl.extensions[ind];\n",
+ " var option = $(\n",
+ " '', {selected: fmt === mpl.default_extension}).html(fmt);\n",
+ " fmt_picker.append(option);\n",
+ " }\n",
+ "\n",
+ " // Add hover states to the ui-buttons\n",
+ " $( \".ui-button\" ).hover(\n",
+ " function() { $(this).addClass(\"ui-state-hover\");},\n",
+ " function() { $(this).removeClass(\"ui-state-hover\");}\n",
+ " );\n",
+ "\n",
+ " var status_bar = $('');\n",
+ " nav_element.append(status_bar);\n",
+ " this.message = status_bar[0];\n",
+ "}\n",
+ "\n",
+ "mpl.figure.prototype.request_resize = function(x_pixels, y_pixels) {\n",
+ " // Request matplotlib to resize the figure. Matplotlib will then trigger a resize in the client,\n",
+ " // which will in turn request a refresh of the image.\n",
+ " this.send_message('resize', {'width': x_pixels, 'height': y_pixels});\n",
+ "}\n",
+ "\n",
+ "mpl.figure.prototype.send_message = function(type, properties) {\n",
+ " properties['type'] = type;\n",
+ " properties['figure_id'] = this.id;\n",
+ " this.ws.send(JSON.stringify(properties));\n",
+ "}\n",
+ "\n",
+ "mpl.figure.prototype.send_draw_message = function() {\n",
+ " if (!this.waiting) {\n",
+ " this.waiting = true;\n",
+ " this.ws.send(JSON.stringify({type: \"draw\", figure_id: this.id}));\n",
+ " }\n",
+ "}\n",
+ "\n",
+ "\n",
+ "mpl.figure.prototype.handle_save = function(fig, msg) {\n",
+ " var format_dropdown = fig.format_dropdown;\n",
+ " var format = format_dropdown.options[format_dropdown.selectedIndex].value;\n",
+ " fig.ondownload(fig, format);\n",
+ "}\n",
+ "\n",
+ "\n",
+ "mpl.figure.prototype.handle_resize = function(fig, msg) {\n",
+ " var size = msg['size'];\n",
+ " if (size[0] != fig.canvas.width || size[1] != fig.canvas.height) {\n",
+ " fig._resize_canvas(size[0], size[1]);\n",
+ " fig.send_message(\"refresh\", {});\n",
+ " };\n",
+ "}\n",
+ "\n",
+ "mpl.figure.prototype.handle_rubberband = function(fig, msg) {\n",
+ " var x0 = msg['x0'] / mpl.ratio;\n",
+ " var y0 = (fig.canvas.height - msg['y0']) / mpl.ratio;\n",
+ " var x1 = msg['x1'] / mpl.ratio;\n",
+ " var y1 = (fig.canvas.height - msg['y1']) / mpl.ratio;\n",
+ " x0 = Math.floor(x0) + 0.5;\n",
+ " y0 = Math.floor(y0) + 0.5;\n",
+ " x1 = Math.floor(x1) + 0.5;\n",
+ " y1 = Math.floor(y1) + 0.5;\n",
+ " var min_x = Math.min(x0, x1);\n",
+ " var min_y = Math.min(y0, y1);\n",
+ " var width = Math.abs(x1 - x0);\n",
+ " var height = Math.abs(y1 - y0);\n",
+ "\n",
+ " fig.rubberband_context.clearRect(\n",
+ " 0, 0, fig.canvas.width / mpl.ratio, fig.canvas.height / mpl.ratio);\n",
+ "\n",
+ " fig.rubberband_context.strokeRect(min_x, min_y, width, height);\n",
+ "}\n",
+ "\n",
+ "mpl.figure.prototype.handle_figure_label = function(fig, msg) {\n",
+ " // Updates the figure title.\n",
+ " fig.header.textContent = msg['label'];\n",
+ "}\n",
+ "\n",
+ "mpl.figure.prototype.handle_cursor = function(fig, msg) {\n",
+ " var cursor = msg['cursor'];\n",
+ " switch(cursor)\n",
+ " {\n",
+ " case 0:\n",
+ " cursor = 'pointer';\n",
+ " break;\n",
+ " case 1:\n",
+ " cursor = 'default';\n",
+ " break;\n",
+ " case 2:\n",
+ " cursor = 'crosshair';\n",
+ " break;\n",
+ " case 3:\n",
+ " cursor = 'move';\n",
+ " break;\n",
+ " }\n",
+ " fig.rubberband_canvas.style.cursor = cursor;\n",
+ "}\n",
+ "\n",
+ "mpl.figure.prototype.handle_message = function(fig, msg) {\n",
+ " fig.message.textContent = msg['message'];\n",
+ "}\n",
+ "\n",
+ "mpl.figure.prototype.handle_draw = function(fig, msg) {\n",
+ " // Request the server to send over a new figure.\n",
+ " fig.send_draw_message();\n",
+ "}\n",
+ "\n",
+ "mpl.figure.prototype.handle_image_mode = function(fig, msg) {\n",
+ " fig.image_mode = msg['mode'];\n",
+ "}\n",
+ "\n",
+ "mpl.figure.prototype.updated_canvas_event = function() {\n",
+ " // Called whenever the canvas gets updated.\n",
+ " this.send_message(\"ack\", {});\n",
+ "}\n",
+ "\n",
+ "// A function to construct a web socket function for onmessage handling.\n",
+ "// Called in the figure constructor.\n",
+ "mpl.figure.prototype._make_on_message_function = function(fig) {\n",
+ " return function socket_on_message(evt) {\n",
+ " if (evt.data instanceof Blob) {\n",
+ " /* FIXME: We get \"Resource interpreted as Image but\n",
+ " * transferred with MIME type text/plain:\" errors on\n",
+ " * Chrome. But how to set the MIME type? It doesn't seem\n",
+ " * to be part of the websocket stream */\n",
+ " evt.data.type = \"image/png\";\n",
+ "\n",
+ " /* Free the memory for the previous frames */\n",
+ " if (fig.imageObj.src) {\n",
+ " (window.URL || window.webkitURL).revokeObjectURL(\n",
+ " fig.imageObj.src);\n",
+ " }\n",
+ "\n",
+ " fig.imageObj.src = (window.URL || window.webkitURL).createObjectURL(\n",
+ " evt.data);\n",
+ " fig.updated_canvas_event();\n",
+ " fig.waiting = false;\n",
+ " return;\n",
+ " }\n",
+ " else if (typeof evt.data === 'string' && evt.data.slice(0, 21) == \"data:image/png;base64\") {\n",
+ " fig.imageObj.src = evt.data;\n",
+ " fig.updated_canvas_event();\n",
+ " fig.waiting = false;\n",
+ " return;\n",
+ " }\n",
+ "\n",
+ " var msg = JSON.parse(evt.data);\n",
+ " var msg_type = msg['type'];\n",
+ "\n",
+ " // Call the \"handle_{type}\" callback, which takes\n",
+ " // the figure and JSON message as its only arguments.\n",
+ " try {\n",
+ " var callback = fig[\"handle_\" + msg_type];\n",
+ " } catch (e) {\n",
+ " console.log(\"No handler for the '\" + msg_type + \"' message type: \", msg);\n",
+ " return;\n",
+ " }\n",
+ "\n",
+ " if (callback) {\n",
+ " try {\n",
+ " // console.log(\"Handling '\" + msg_type + \"' message: \", msg);\n",
+ " callback(fig, msg);\n",
+ " } catch (e) {\n",
+ " console.log(\"Exception inside the 'handler_\" + msg_type + \"' callback:\", e, e.stack, msg);\n",
+ " }\n",
+ " }\n",
+ " };\n",
+ "}\n",
+ "\n",
+ "// from http://stackoverflow.com/questions/1114465/getting-mouse-location-in-canvas\n",
+ "mpl.findpos = function(e) {\n",
+ " //this section is from http://www.quirksmode.org/js/events_properties.html\n",
+ " var targ;\n",
+ " if (!e)\n",
+ " e = window.event;\n",
+ " if (e.target)\n",
+ " targ = e.target;\n",
+ " else if (e.srcElement)\n",
+ " targ = e.srcElement;\n",
+ " if (targ.nodeType == 3) // defeat Safari bug\n",
+ " targ = targ.parentNode;\n",
+ "\n",
+ " // jQuery normalizes the pageX and pageY\n",
+ " // pageX,Y are the mouse positions relative to the document\n",
+ " // offset() returns the position of the element relative to the document\n",
+ " var x = e.pageX - $(targ).offset().left;\n",
+ " var y = e.pageY - $(targ).offset().top;\n",
+ "\n",
+ " return {\"x\": x, \"y\": y};\n",
+ "};\n",
+ "\n",
+ "/*\n",
+ " * return a copy of an object with only non-object keys\n",
+ " * we need this to avoid circular references\n",
+ " * http://stackoverflow.com/a/24161582/3208463\n",
+ " */\n",
+ "function simpleKeys (original) {\n",
+ " return Object.keys(original).reduce(function (obj, key) {\n",
+ " if (typeof original[key] !== 'object')\n",
+ " obj[key] = original[key]\n",
+ " return obj;\n",
+ " }, {});\n",
+ "}\n",
+ "\n",
+ "mpl.figure.prototype.mouse_event = function(event, name) {\n",
+ " var canvas_pos = mpl.findpos(event)\n",
+ "\n",
+ " if (name === 'button_press')\n",
+ " {\n",
+ " this.canvas.focus();\n",
+ " this.canvas_div.focus();\n",
+ " }\n",
+ "\n",
+ " var x = canvas_pos.x * mpl.ratio;\n",
+ " var y = canvas_pos.y * mpl.ratio;\n",
+ "\n",
+ " this.send_message(name, {x: x, y: y, button: event.button,\n",
+ " step: event.step,\n",
+ " guiEvent: simpleKeys(event)});\n",
+ "\n",
+ " /* This prevents the web browser from automatically changing to\n",
+ " * the text insertion cursor when the button is pressed. We want\n",
+ " * to control all of the cursor setting manually through the\n",
+ " * 'cursor' event from matplotlib */\n",
+ " event.preventDefault();\n",
+ " return false;\n",
+ "}\n",
+ "\n",
+ "mpl.figure.prototype._key_event_extra = function(event, name) {\n",
+ " // Handle any extra behaviour associated with a key event\n",
+ "}\n",
+ "\n",
+ "mpl.figure.prototype.key_event = function(event, name) {\n",
+ "\n",
+ " // Prevent repeat events\n",
+ " if (name == 'key_press')\n",
+ " {\n",
+ " if (event.which === this._key)\n",
+ " return;\n",
+ " else\n",
+ " this._key = event.which;\n",
+ " }\n",
+ " if (name == 'key_release')\n",
+ " this._key = null;\n",
+ "\n",
+ " var value = '';\n",
+ " if (event.ctrlKey && event.which != 17)\n",
+ " value += \"ctrl+\";\n",
+ " if (event.altKey && event.which != 18)\n",
+ " value += \"alt+\";\n",
+ " if (event.shiftKey && event.which != 16)\n",
+ " value += \"shift+\";\n",
+ "\n",
+ " value += 'k';\n",
+ " value += event.which.toString();\n",
+ "\n",
+ " this._key_event_extra(event, name);\n",
+ "\n",
+ " this.send_message(name, {key: value,\n",
+ " guiEvent: simpleKeys(event)});\n",
+ " return false;\n",
+ "}\n",
+ "\n",
+ "mpl.figure.prototype.toolbar_button_onclick = function(name) {\n",
+ " if (name == 'download') {\n",
+ " this.handle_save(this, null);\n",
+ " } else {\n",
+ " this.send_message(\"toolbar_button\", {name: name});\n",
+ " }\n",
+ "};\n",
+ "\n",
+ "mpl.figure.prototype.toolbar_button_onmouseover = function(tooltip) {\n",
+ " this.message.textContent = tooltip;\n",
+ "};\n",
+ "mpl.toolbar_items = [[\"Home\", \"Reset original view\", \"fa fa-home icon-home\", \"home\"], [\"Back\", \"Back to previous view\", \"fa fa-arrow-left icon-arrow-left\", \"back\"], [\"Forward\", \"Forward to next view\", \"fa fa-arrow-right icon-arrow-right\", \"forward\"], [\"\", \"\", \"\", \"\"], [\"Pan\", \"Pan axes with left mouse, zoom with right\", \"fa fa-arrows icon-move\", \"pan\"], [\"Zoom\", \"Zoom to rectangle\", \"fa fa-square-o icon-check-empty\", \"zoom\"], [\"\", \"\", \"\", \"\"], [\"Download\", \"Download plot\", \"fa fa-floppy-o icon-save\", \"download\"]];\n",
+ "\n",
+ "mpl.extensions = [\"eps\", \"jpeg\", \"pdf\", \"png\", \"ps\", \"raw\", \"svg\", \"tif\"];\n",
+ "\n",
+ "mpl.default_extension = \"png\";var comm_websocket_adapter = function(comm) {\n",
+ " // Create a \"websocket\"-like object which calls the given IPython comm\n",
+ " // object with the appropriate methods. Currently this is a non binary\n",
+ " // socket, so there is still some room for performance tuning.\n",
+ " var ws = {};\n",
+ "\n",
+ " ws.close = function() {\n",
+ " comm.close()\n",
+ " };\n",
+ " ws.send = function(m) {\n",
+ " //console.log('sending', m);\n",
+ " comm.send(m);\n",
+ " };\n",
+ " // Register the callback with on_msg.\n",
+ " comm.on_msg(function(msg) {\n",
+ " //console.log('receiving', msg['content']['data'], msg);\n",
+ " // Pass the mpl event to the overridden (by mpl) onmessage function.\n",
+ " ws.onmessage(msg['content']['data'])\n",
+ " });\n",
+ " return ws;\n",
+ "}\n",
+ "\n",
+ "mpl.mpl_figure_comm = function(comm, msg) {\n",
+ " // This is the function which gets called when the mpl process\n",
+ " // starts-up an IPython Comm through the \"matplotlib\" channel.\n",
+ "\n",
+ " var id = msg.content.data.id;\n",
+ " // Get hold of the div created by the display call when the Comm\n",
+ " // socket was opened in Python.\n",
+ " var element = $(\"#\" + id);\n",
+ " var ws_proxy = comm_websocket_adapter(comm)\n",
+ "\n",
+ " function ondownload(figure, format) {\n",
+ " window.open(figure.imageObj.src);\n",
+ " }\n",
+ "\n",
+ " var fig = new mpl.figure(id, ws_proxy,\n",
+ " ondownload,\n",
+ " element.get(0));\n",
+ "\n",
+ " // Call onopen now - mpl needs it, as it is assuming we've passed it a real\n",
+ " // web socket which is closed, not our websocket->open comm proxy.\n",
+ " ws_proxy.onopen();\n",
+ "\n",
+ " fig.parent_element = element.get(0);\n",
+ " fig.cell_info = mpl.find_output_cell(\"\");\n",
+ " if (!fig.cell_info) {\n",
+ " console.error(\"Failed to find cell for figure\", id, fig);\n",
+ " return;\n",
+ " }\n",
+ "\n",
+ " var output_index = fig.cell_info[2]\n",
+ " var cell = fig.cell_info[0];\n",
+ "\n",
+ "};\n",
+ "\n",
+ "mpl.figure.prototype.handle_close = function(fig, msg) {\n",
+ " var width = fig.canvas.width/mpl.ratio\n",
+ " fig.root.unbind('remove')\n",
+ "\n",
+ " // Update the output cell to use the data from the current canvas.\n",
+ " fig.push_to_output();\n",
+ " var dataURL = fig.canvas.toDataURL();\n",
+ " // Re-enable the keyboard manager in IPython - without this line, in FF,\n",
+ " // the notebook keyboard shortcuts fail.\n",
+ " IPython.keyboard_manager.enable()\n",
+ " $(fig.parent_element).html('
');\n",
+ " fig.close_ws(fig, msg);\n",
+ "}\n",
+ "\n",
+ "mpl.figure.prototype.close_ws = function(fig, msg){\n",
+ " fig.send_message('closing', msg);\n",
+ " // fig.ws.close()\n",
+ "}\n",
+ "\n",
+ "mpl.figure.prototype.push_to_output = function(remove_interactive) {\n",
+ " // Turn the data on the canvas into data in the output cell.\n",
+ " var width = this.canvas.width/mpl.ratio\n",
+ " var dataURL = this.canvas.toDataURL();\n",
+ " this.cell_info[1]['text/html'] = '
';\n",
+ "}\n",
+ "\n",
+ "mpl.figure.prototype.updated_canvas_event = function() {\n",
+ " // Tell IPython that the notebook contents must change.\n",
+ " IPython.notebook.set_dirty(true);\n",
+ " this.send_message(\"ack\", {});\n",
+ " var fig = this;\n",
+ " // Wait a second, then push the new image to the DOM so\n",
+ " // that it is saved nicely (might be nice to debounce this).\n",
+ " setTimeout(function () { fig.push_to_output() }, 1000);\n",
+ "}\n",
+ "\n",
+ "mpl.figure.prototype._init_toolbar = function() {\n",
+ " var fig = this;\n",
+ "\n",
+ " var nav_element = $('');\n",
+ " nav_element.attr('style', 'width: 100%');\n",
+ " this.root.append(nav_element);\n",
+ "\n",
+ " // Define a callback function for later on.\n",
+ " function toolbar_event(event) {\n",
+ " return fig.toolbar_button_onclick(event['data']);\n",
+ " }\n",
+ " function toolbar_mouse_event(event) {\n",
+ " return fig.toolbar_button_onmouseover(event['data']);\n",
+ " }\n",
+ "\n",
+ " for(var toolbar_ind in mpl.toolbar_items){\n",
+ " var name = mpl.toolbar_items[toolbar_ind][0];\n",
+ " var tooltip = mpl.toolbar_items[toolbar_ind][1];\n",
+ " var image = mpl.toolbar_items[toolbar_ind][2];\n",
+ " var method_name = mpl.toolbar_items[toolbar_ind][3];\n",
+ "\n",
+ " if (!name) { continue; };\n",
+ "\n",
+ " var button = $('');\n",
+ " button.click(method_name, toolbar_event);\n",
+ " button.mouseover(tooltip, toolbar_mouse_event);\n",
+ " nav_element.append(button);\n",
+ " }\n",
+ "\n",
+ " // Add the status bar.\n",
+ " var status_bar = $('');\n",
+ " nav_element.append(status_bar);\n",
+ " this.message = status_bar[0];\n",
+ "\n",
+ " // Add the close button to the window.\n",
+ " var buttongrp = $('');\n",
+ " var button = $('');\n",
+ " button.click(function (evt) { fig.handle_close(fig, {}); } );\n",
+ " button.mouseover('Stop Interaction', toolbar_mouse_event);\n",
+ " buttongrp.append(button);\n",
+ " var titlebar = this.root.find($('.ui-dialog-titlebar'));\n",
+ " titlebar.prepend(buttongrp);\n",
+ "}\n",
+ "\n",
+ "mpl.figure.prototype._root_extra_style = function(el){\n",
+ " var fig = this\n",
+ " el.on(\"remove\", function(){\n",
+ "\tfig.close_ws(fig, {});\n",
+ " });\n",
+ "}\n",
+ "\n",
+ "mpl.figure.prototype._canvas_extra_style = function(el){\n",
+ " // this is important to make the div 'focusable\n",
+ " el.attr('tabindex', 0)\n",
+ " // reach out to IPython and tell the keyboard manager to turn it's self\n",
+ " // off when our div gets focus\n",
+ "\n",
+ " // location in version 3\n",
+ " if (IPython.notebook.keyboard_manager) {\n",
+ " IPython.notebook.keyboard_manager.register_events(el);\n",
+ " }\n",
+ " else {\n",
+ " // location in version 2\n",
+ " IPython.keyboard_manager.register_events(el);\n",
+ " }\n",
+ "\n",
+ "}\n",
+ "\n",
+ "mpl.figure.prototype._key_event_extra = function(event, name) {\n",
+ " var manager = IPython.notebook.keyboard_manager;\n",
+ " if (!manager)\n",
+ " manager = IPython.keyboard_manager;\n",
+ "\n",
+ " // Check for shift+enter\n",
+ " if (event.shiftKey && event.which == 13) {\n",
+ " this.canvas_div.blur();\n",
+ " event.shiftKey = false;\n",
+ " // Send a \"J\" for go to next cell\n",
+ " event.which = 74;\n",
+ " event.keyCode = 74;\n",
+ " manager.command_mode();\n",
+ " manager.handle_keydown(event);\n",
+ " }\n",
+ "}\n",
+ "\n",
+ "mpl.figure.prototype.handle_save = function(fig, msg) {\n",
+ " fig.ondownload(fig, null);\n",
+ "}\n",
+ "\n",
+ "\n",
+ "mpl.find_output_cell = function(html_output) {\n",
+ " // Return the cell and output element which can be found *uniquely* in the notebook.\n",
+ " // Note - this is a bit hacky, but it is done because the \"notebook_saving.Notebook\"\n",
+ " // IPython event is triggered only after the cells have been serialised, which for\n",
+ " // our purposes (turning an active figure into a static one), is too late.\n",
+ " var cells = IPython.notebook.get_cells();\n",
+ " var ncells = cells.length;\n",
+ " for (var i=0; i= 3 moved mimebundle to data attribute of output\n",
+ " data = data.data;\n",
+ " }\n",
+ " if (data['text/html'] == html_output) {\n",
+ " return [cell, data, j];\n",
+ " }\n",
+ " }\n",
+ " }\n",
+ " }\n",
+ "}\n",
+ "\n",
+ "// Register the function which deals with the matplotlib target/channel.\n",
+ "// The kernel may be null if the page has been refreshed.\n",
+ "if (IPython.notebook.kernel != null) {\n",
+ " IPython.notebook.kernel.comm_manager.register_target('matplotlib', mpl.mpl_figure_comm);\n",
+ "}\n"
+ ],
+ "text/plain": [
+ ""
+ ]
+ },
+ "metadata": {},
+ "output_type": "display_data"
+ },
+ {
+ "data": {
+ "text/html": [
+ "
"
+ ],
+ "text/plain": [
+ ""
+ ]
+ },
+ "metadata": {},
+ "output_type": "display_data"
+ }
+ ],
+ "source": [
+ "fig = plt.figure(3)\n",
+ "plt.imshow(im1, interpolation='nearest')\n",
+ "\n",
+ "coords = []\n",
+ "connectId = fig.canvas.mpl_connect('button_press_event', onclick)"
+ ]
+ },
+ {
+ "cell_type": "markdown",
+ "metadata": {},
+ "source": [
+ "Notice that in the previous code cell, we created an empty list named `coords`, and inside the `onclick()` function, we are appending to it the $(x,y)$ coordinates of each mouse click on the figure. After executing the cell above, you have a connection to the figure, via the user interface: try clicking with your mouse on the endpoints of the white lines of the metered panel (click on the edge of the panel to get approximately equal $x$ coordinates), then print the contents of the `coords` list below."
+ ]
+ },
+ {
+ "cell_type": "code",
+ "execution_count": 16,
+ "metadata": {},
+ "outputs": [
+ {
+ "data": {
+ "text/plain": [
+ "[]"
+ ]
+ },
+ "execution_count": 16,
+ "metadata": {},
+ "output_type": "execute_result"
+ }
+ ],
+ "source": [
+ "coords"
+ ]
+ },
+ {
+ "cell_type": "markdown",
+ "metadata": {},
+ "source": [
+ "The $x$ coordinates are pretty close, but there is some variation due to our shaky hand (or bad eyesight), and perhaps because the metered panel is not perfectly vertical. We can cast the `coords` list to a NumPy array, then grab all the first elements of the coordinate pairs, then get the standard deviation as an indication of our error in the mouse-click captures."
+ ]
+ },
+ {
+ "cell_type": "markdown",
+ "metadata": {},
+ "source": [
+ "## Save your work to a file\n",
+ "\n",
+ "In the next two code cells, we assign the clicks that were in the list `coords` to a numpy array `hits1`. Then, we save this array to the file `hits_im1.npy`. This is a numpy-readable file that we can load back into our workspace later as `np.load('../data/hits_im1.npy')`."
+ ]
+ },
+ {
+ "cell_type": "code",
+ "execution_count": 17,
+ "metadata": {},
+ "outputs": [],
+ "source": [
+ "hits1 = np.array(coords)"
+ ]
+ },
{
"cell_type": "code",
- "execution_count": 2,
+ "execution_count": 18,
"metadata": {},
"outputs": [],
"source": [
- "import matplotlib.pyplot as plt\n",
- "from matplotlib.image import imread\n",
- "import numpy as np\n",
- "%matplotlib notebook"
+ "np.save('../data/hits_im1.npy',hits1)"
]
},
{
"cell_type": "code",
- "execution_count": 3,
+ "execution_count": 47,
"metadata": {},
"outputs": [
{
@@ -800,7 +2529,7 @@
{
"data": {
"text/html": [
- "
"
+ "
"
],
"text/plain": [
""
@@ -812,21 +2541,44 @@
{
"data": {
"text/plain": [
- "[]"
+ "[]"
]
},
- "execution_count": 3,
+ "execution_count": 47,
"metadata": {},
"output_type": "execute_result"
}
],
"source": [
- "plt.plot(np.random.rand(10))"
+ "plt.figure()\n",
+ "plt.imshow(im1)\n",
+ "\n",
+ "plt.plot(hits1[:,0],hits1[:,1],'x')\n",
+ "#plt.plot(rings[:,0],rings[:,1],'s')"
+ ]
+ },
+ {
+ "cell_type": "markdown",
+ "metadata": {},
+ "source": [
+ "# Rinse and repeat\n",
+ "\n",
+ "Now we will repeat the process for `hits2` collecting the hit locations in target_02.jpg."
+ ]
+ },
+ {
+ "cell_type": "markdown",
+ "metadata": {},
+ "source": [
+ "### Capture mouse clicks on the frame\n",
+ "\n",
+ "Once again we create [event connections](https://matplotlib.org/devdocs/users/event_handling.html?highlight=mpl_connect), that is, connect the figure canvas to user-interface events on it, like mouse clicks. \n",
+ "We connect the `'button_press_event'` to the function named `onclick()`, which captures the $(x,y)$ coordinates of the mouse click on the figure. Magic!"
]
},
{
"cell_type": "code",
- "execution_count": 6,
+ "execution_count": 20,
"metadata": {},
"outputs": [
{
@@ -1612,7 +3364,7 @@
{
"data": {
"text/html": [
- "
"
+ "
"
],
"text/plain": [
""
@@ -1620,50 +3372,53 @@
},
"metadata": {},
"output_type": "display_data"
- },
- {
- "data": {
- "text/plain": [
- ""
- ]
- },
- "execution_count": 6,
- "metadata": {},
- "output_type": "execute_result"
}
],
"source": [
- "im = imread('../data/archery.jpg')\n",
- "plt.figure()\n",
- "plt.imshow(im)\n"
+ "fig = plt.figure()\n",
+ "plt.imshow(im2, interpolation='nearest')\n",
+ "\n",
+ "coords = []\n",
+ "connectId = fig.canvas.mpl_connect('button_press_event', onclick)"
]
},
{
"cell_type": "markdown",
"metadata": {},
"source": [
- "### Capture mouse clicks on the frame\n",
- "\n",
- "Okay! Here is where things get really interesting. Matplotlib has the ability to create [event connections](https://matplotlib.org/devdocs/users/event_handling.html?highlight=mpl_connect), that is, connect the figure canvas to user-interface events on it, like mouse clicks. \n",
+ "The $x$ coordinates are pretty close, but there is some variation due to our shaky hand (or bad eyesight), and perhaps because the metered panel is not perfectly vertical. We can cast the `coords` list to a NumPy array, then grab all the first elements of the coordinate pairs, then get the standard deviation as an indication of our error in the mouse-click captures."
+ ]
+ },
+ {
+ "cell_type": "code",
+ "execution_count": 21,
+ "metadata": {},
+ "outputs": [],
+ "source": [
+ "hits2 = np.array(coords)"
+ ]
+ },
+ {
+ "cell_type": "markdown",
+ "metadata": {},
+ "source": [
+ "## Save your work to a file\n",
"\n",
- "To use this ability, you write a function with the events you want to capture, and then connect this function to the Matplotlib \"event manager\" using [`mpl_connect()`](https://matplotlib.org/devdocs/api/backend_bases_api.html#matplotlib.backend_bases.FigureCanvasBase.mpl_connect). In this case, we connect the `'button_press_event'` to the function named `onclick()`, which captures the $(x,y)$ coordinates of the mouse click on the figure. Magic!"
+ "In the next two code cells, we assign the clicks that were in the list `coords` to a numpy array `hits2`. Then, we save this array to the file `hits_im2.npy`. This is a numpy-readable file that we can load back into our workspace later as `np.load('../data/hits_im2.npy')`."
]
},
{
"cell_type": "code",
- "execution_count": 10,
+ "execution_count": 44,
"metadata": {},
"outputs": [],
"source": [
- "def onclick(event):\n",
- " '''Capture the x,y coordinates of a mouse click on the image'''\n",
- " ix, iy = event.xdata, event.ydata\n",
- " coords.append([ix, iy]) "
+ "np.save('../data/hits_im2.npy',hits2)"
]
},
{
"cell_type": "code",
- "execution_count": 63,
+ "execution_count": 45,
"metadata": {},
"outputs": [
{
@@ -2449,7 +4204,7 @@
{
"data": {
"text/html": [
- "
"
+ "
"
],
"text/plain": [
""
@@ -2457,81 +4212,41 @@
},
"metadata": {},
"output_type": "display_data"
- }
- ],
- "source": [
- "fig = plt.figure(3)\n",
- "plt.imshow(im, interpolation='nearest')\n",
- "\n",
- "coords = []\n",
- "connectId = fig.canvas.mpl_connect('button_press_event', onclick)"
- ]
- },
- {
- "cell_type": "markdown",
- "metadata": {},
- "source": [
- "Notice that in the previous code cell, we created an empty list named `coords`, and inside the `onclick()` function, we are appending to it the $(x,y)$ coordinates of each mouse click on the figure. After executing the cell above, you have a connection to the figure, via the user interface: try clicking with your mouse on the endpoints of the white lines of the metered panel (click on the edge of the panel to get approximately equal $x$ coordinates), then print the contents of the `coords` list below."
- ]
- },
- {
- "cell_type": "code",
- "execution_count": 66,
- "metadata": {},
- "outputs": [
+ },
{
"data": {
"text/plain": [
- "[[229.51449092741933, 245.74974798387098],\n",
- " [229.51449092741933, 324.3787802419355],\n",
- " [325.44191028225805, 292.9271673387097],\n",
- " [364.7564264112903, 300.7900705645161],\n",
- " [380.4822328629032, 308.65297379032256],\n",
- " [367.90158770161287, 329.09652217741933],\n",
- " [339.59513608870964, 346.39490927419354],\n",
- " [339.59513608870964, 371.5561995967742],\n",
- " [364.7564264112903, 363.69329637096774],\n",
- " [309.7161038306451, 393.57232862903226],\n",
- " [308.14352318548384, 443.89490927419354],\n",
- " [408.7886844758064, 421.8787802419355],\n",
- " [473.26449092741933, 376.27394153225805],\n",
- " [465.40158770161287, 280.34652217741933],\n",
- " [496.8532006048387, 267.765877016129],\n",
- " [452.8209425403225, 242.6045866935484],\n",
- " [430.80481350806446, 220.5884576612903],\n",
- " [627.3773941532259, 187.56426411290323],\n",
- " [350.60320060483866, 176.5561995967742],\n",
- " [364.7564264112903, 206.43523185483872]]"
+ "[]"
]
},
- "execution_count": 66,
+ "execution_count": 45,
"metadata": {},
"output_type": "execute_result"
}
],
"source": [
- "coords"
+ "plt.figure()\n",
+ "plt.imshow(im2)\n",
+ "\n",
+ "plt.plot(hits2[:,0],hits2[:,1],'x')"
]
},
{
"cell_type": "markdown",
"metadata": {},
"source": [
- "The $x$ coordinates are pretty close, but there is some variation due to our shaky hand (or bad eyesight), and perhaps because the metered panel is not perfectly vertical. We can cast the `coords` list to a NumPy array, then grab all the first elements of the coordinate pairs, then get the standard deviation as an indication of our error in the mouse-click captures."
- ]
- },
- {
- "cell_type": "code",
- "execution_count": null,
- "metadata": {},
- "outputs": [],
- "source": [
- "hits = np.array(coords)"
+ "## Set the scale to convert pixels -> meters\n",
+ "\n",
+ "In the next two `imshow` commands, we will choose the corners of the target shown below as location 1, 2, 3, and 4 and the center we will determine from the average locations of locations 1-4 in $\\times$ . \n",
+ "\n",
+ "\n",
+ "\n",
+ "We use the same `onclick` function to create the `corners1` array for target_01.jpg and `corners2` for targete_02.jpg. "
]
},
{
"cell_type": "code",
- "execution_count": 81,
+ "execution_count": 25,
"metadata": {},
"outputs": [
{
@@ -3317,7 +5032,7 @@
{
"data": {
"text/html": [
- "
"
+ "
"
],
"text/plain": [
""
@@ -3325,49 +5040,28 @@
},
"metadata": {},
"output_type": "display_data"
- },
- {
- "data": {
- "text/plain": [
- "[]"
- ]
- },
- "execution_count": 81,
- "metadata": {},
- "output_type": "execute_result"
}
],
"source": [
- "plt.figure()\n",
- "plt.imshow(im)\n",
+ "fig = plt.figure()\n",
+ "plt.imshow(im1, interpolation='nearest')\n",
"\n",
- "plt.plot(hits[:,0],hits[:,1],'x')\n",
- "plt.plot(rings[:,0],rings[:,1],'s')"
+ "coords = []\n",
+ "connectId = fig.canvas.mpl_connect('button_press_event', onclick)"
]
},
{
"cell_type": "code",
- "execution_count": 73,
+ "execution_count": 26,
"metadata": {},
- "outputs": [
- {
- "data": {
- "text/plain": [
- "array([ 0, 8, 16, 24])"
- ]
- },
- "execution_count": 73,
- "metadata": {},
- "output_type": "execute_result"
- }
- ],
+ "outputs": [],
"source": [
- "rings"
+ "corners1 =np.array(coords)"
]
},
{
"cell_type": "code",
- "execution_count": 68,
+ "execution_count": 27,
"metadata": {},
"outputs": [
{
@@ -4153,7 +5847,7 @@
{
"data": {
"text/html": [
- "
"
+ "
"
],
"text/plain": [
""
@@ -4165,7 +5859,7 @@
],
"source": [
"fig = plt.figure()\n",
- "plt.imshow(im, interpolation='nearest')\n",
+ "plt.imshow(im2, interpolation='nearest')\n",
"\n",
"coords = []\n",
"connectId = fig.canvas.mpl_connect('button_press_event', onclick)"
@@ -4173,97 +5867,25 @@
},
{
"cell_type": "code",
- "execution_count": 78,
- "metadata": {},
- "outputs": [
- {
- "data": {
- "text/plain": [
- "array([[352.17578125, 283.00025202],\n",
- " [468.54674899, 240.5405746 ],\n",
- " [597.4983619 , 223.2421875 ],\n",
- " [728.02255544, 207.51638105]])"
- ]
- },
- "execution_count": 78,
- "metadata": {},
- "output_type": "execute_result"
- }
- ],
- "source": [
- "rings = np.array(coords)\n",
- "rings"
- ]
- },
- {
- "cell_type": "code",
- "execution_count": 79,
+ "execution_count": 28,
"metadata": {},
- "outputs": [
- {
- "data": {
- "text/plain": [
- "0.06351808135606307"
- ]
- },
- "execution_count": 79,
- "metadata": {},
- "output_type": "execute_result"
- }
- ],
+ "outputs": [],
"source": [
- "bull=rings[0,:]\n",
- "distances=np.sqrt((rings[:,0]-bull[0])**2+(rings[:,1]-bull[1])**2)\n",
- "ring_cm = np.array([0,8,16,24]) # ring distance from Bullseye cm\n",
- "scale =np.mean(ring_cm[1:]/distances[1:]) # scale for distances to cm/px\n",
- "scale"
+ "corners2 =np.array(coords)"
]
},
{
- "cell_type": "code",
- "execution_count": 82,
+ "cell_type": "markdown",
"metadata": {},
- "outputs": [
- {
- "data": {
- "text/plain": [
- "array([[ 7.79120982, 2.36608055],\n",
- " [ 7.79120982, -2.62828472],\n",
- " [ 1.69808419, -0.63053862],\n",
- " [ -0.79909844, -1.12997514],\n",
- " [ -1.7979715 , -1.62941167],\n",
- " [ -0.99887305, -2.92794664],\n",
- " [ 0.79909844, -4.026707 ],\n",
- " [ 0.79909844, -5.62490388],\n",
- " [ -0.79909844, -5.12546736],\n",
- " [ 2.69695724, -7.02332616],\n",
- " [ 2.79684455, -10.21971993],\n",
- " [ -3.59594299, -8.82129765],\n",
- " [ -7.69132251, -5.9245658 ],\n",
- " [ -7.19188599, 0.16855983],\n",
- " [ -9.18963209, 0.96765827],\n",
- " [ -6.39278754, 2.56585516],\n",
- " [ -4.99436527, 3.96427743],\n",
- " [-17.48027844, 6.06191084],\n",
- " [ 0.09988731, 6.76112198],\n",
- " [ -0.79909844, 4.86326318]])"
- ]
- },
- "execution_count": 82,
- "metadata": {},
- "output_type": "execute_result"
- }
- ],
"source": [
- "hit_scale=hits\n",
- "hit_scale[:,0]=(bull[0]-hits[:,0])*scale\n",
- "hit_scale[:,1]=(bull[1]-hits[:,1])*scale\n",
- "hits"
+ "## Set the absolute position and scale results\n",
+ "\n",
+ "With the corner locations save in the `corners1` and `corners2`, we locate the target center as the average x-position and average y-position of all four chosen points. Below, we plot the corners and centers for both images, you can change the `imshow` command to check target_01.jpg and target_02.jpg. "
]
},
{
"cell_type": "code",
- "execution_count": 83,
+ "execution_count": 33,
"metadata": {},
"outputs": [
{
@@ -5049,7 +6671,7 @@
{
"data": {
"text/html": [
- "
"
+ "
"
],
"text/plain": [
""
@@ -5061,23 +6683,97 @@
{
"data": {
"text/plain": [
- "[]"
+ "Text(0, 0.5, 'x-axis')"
]
},
- "execution_count": 83,
+ "execution_count": 33,
"metadata": {},
"output_type": "execute_result"
}
],
"source": [
"plt.figure()\n",
- "plt.plot(hit_scale[:,0],hit_scale[:,1],'o')\n",
- "plt.plot(np.mean(hit_scale[:,0]),np.mean(hit_scale[:,1]),'s')"
+ "\n",
+ "center1_x = np.mean(corners1[:,0])\n",
+ "center1_y = np.mean(corners1[:,1])\n",
+ "\n",
+ "center2_x = np.mean(corners2[:,0])\n",
+ "center2_y = np.mean(corners2[:,1])\n",
+ "\n",
+ "plt.imshow(im1)\n",
+ "\n",
+ "plt.plot(corners1[:,0],corners1[:,1],'o')\n",
+ "plt.plot(corners2[:,0],corners2[:,1],'s')\n",
+ "\n",
+ "plt.plot(center1_x,center1_y,'+')\n",
+ "plt.plot(center2_x,center2_y,'x')\n",
+ "plt.plot(1500,2500,'s')\n",
+ "plt.xlabel('y-axis')\n",
+ "plt.ylabel('x-axis')"
+ ]
+ },
+ {
+ "cell_type": "markdown",
+ "metadata": {},
+ "source": [
+ "You can see above that each image will have a different scale to convert from position in pixels to position in meters. This is the case for all quantitative image data, every time a camera is repositioned you will have a new scale to convert pixels to meters. \n",
+ "\n",
+ "Below, we use the 0.9144-m distance between corner locations to set oour `im1_scale` and `im2_scale` for target_01.jpg and target_02.jpg, respectively. "
+ ]
+ },
+ {
+ "cell_type": "code",
+ "execution_count": 39,
+ "metadata": {},
+ "outputs": [
+ {
+ "name": "stdout",
+ "output_type": "stream",
+ "text": [
+ "target_01.jpg has conversion 0.00059 meter/pixel\n",
+ "target_02.jpg has conversion 0.00048 meter/pixel\n"
+ ]
+ }
+ ],
+ "source": [
+ "d1=np.sqrt((corners1[0,0]-corners1[1,0])**2+(corners1[0,1]-corners1[1,1])**2)\n",
+ "d2=np.sqrt((corners1[2,0]-corners1[3,0])**2+(corners1[2,1]-corners1[3,1])**2)\n",
+ "\n",
+ "\n",
+ "im1_scale = 0.9144/np.mean([d1,d2]) # in 0.9144 meters/ pixel distance\n",
+ "print('target_01.jpg has conversion {:.2} meter/pixel'.format(im1_scale))\n",
+ "d1=np.sqrt((corners2[0,0]-corners2[1,0])**2+(corners2[0,1]-corners2[1,1])**2)\n",
+ "d2=np.sqrt((corners2[2,0]-corners2[3,0])**2+(corners2[2,1]-corners2[3,1])**2)\n",
+ "\n",
+ "\n",
+ "im2_scale = 0.9144/np.mean([d1,d2]) # in 0.9144 meters/ pixel distance\n",
+ "print('target_02.jpg has conversion {:.2} meter/pixel'.format(im2_scale))"
+ ]
+ },
+ {
+ "cell_type": "markdown",
+ "metadata": {},
+ "source": [
+ "# Final Data preparation\n",
+ "\n",
+ "So far, we have\n",
+ "\n",
+ "1. Chosen hit points on two images\n",
+ "\n",
+ "2. Chosen calibration points on two images\n",
+ "\n",
+ "2. Determined a local reference in each image i.e. point (0,0)\n",
+ "\n",
+ "3. Determined the scale for each image i.e. convert pixels -> meters\n",
+ "\n",
+ "The final data preparation is to convert the chosen hit points on the two images into (x,y) locations in meters. We will save some other information in case we need to check our work later _(part of creating reproducible research)_.\n",
+ "\n",
+ "The first step is to plot our scaled and centered data from targets 01 and 02. We can also plot the mean location of our hits from the class. "
]
},
{
"cell_type": "code",
- "execution_count": 98,
+ "execution_count": 42,
"metadata": {},
"outputs": [
{
@@ -5863,7 +7559,7 @@
{
"data": {
"text/html": [
- "
"
+ "
"
],
"text/plain": [
""
@@ -5875,89 +7571,83 @@
{
"data": {
"text/plain": [
- "(array([1., 1., 0., 2., 0., 1., 0., 1., 1., 1.]),\n",
- " array([0.44381555, 1.12382265, 1.80382975, 2.48383685, 3.16384395,\n",
- " 3.84385105, 4.52385815, 5.20386525, 5.88387235, 6.56387946,\n",
- " 7.24388656]),\n",
- " )"
+ ""
]
},
- "execution_count": 98,
+ "execution_count": 42,
"metadata": {},
"output_type": "execute_result"
}
],
"source": [
- "angle=np.arctan2(hit_scale[:,1],hit_scale[:,0])\n",
- "radius=np.sqrt(hit_scale[:,1]**2+hit_scale[:,0]**2)\n",
+ "hits1=np.load('./hits_im1.npy')\n",
+ "hits2=np.load('./hits_im2.npy')\n",
+ "\n",
+ "center_hits1=hits1\n",
+ "center_hits1[:,0]= hits1[:,0]-center1_x\n",
+ "center_hits1[:,1]= hits1[:,1]-center1_y\n",
+ "\n",
+ "center_hits2=hits2\n",
+ "center_hits2[:,0]= hits2[:,0]-center2_x\n",
+ "center_hits2[:,1]= hits2[:,1]-center2_y\n",
+ "\n",
+ "scaled_hits1 = center_hits1*im1_scale\n",
+ "scaled_hits2 = center_hits2*im2_scale\n",
+ "\n",
"plt.figure()\n",
- "#plt.hist(angle)\n",
- "plt.hist(radius)\n"
+ "plt.plot(scaled_hits1[:,0],scaled_hits1[:,1],'x')\n",
+ "plt.plot(scaled_hits2[:,0],scaled_hits2[:,1],'x')\n",
+ "\n",
+ "plt.plot(np.mean(scaled_hits1[:,0]),np.mean(scaled_hits1[:,1]),'o',label='mean hit target_01')\n",
+ "plt.plot(np.mean(scaled_hits2[:,0]),np.mean(scaled_hits2[:,1]),'o',label='mean hit target_02')\n",
+ "plt.legend()\n"
]
},
{
"cell_type": "markdown",
"metadata": {},
"source": [
- "Looking ahead, what we'll do is repeat the process of capturing mouse clicks on the image, but clicking on the ball positions. Then, we will want to have the vertical positions converted to physical length (in meters), from the pixel numbers on the image.\n",
+ "The locations look good so far, now we export the data to a csv file so we can access it later. \n",
"\n",
- "You can get the scaling from pixels to meters via the distance between two white lines on the metered panel, which we know is $0.25\\rm{m}$. \n",
+ "The csv file will save the following information:\n",
"\n",
- "Let's get the average vertical distance between two while lines, which we can calculate as:\n",
- "\n",
- "\\begin{equation}\n",
- "\\overline{\\Delta y} = \\sum_{i=0}^N \\frac{y_{i+1}-y_i}{N-1}\n",
- "\\end{equation}"
+ "|throw #| x position (m)| y position (m)|picture x position (pixel)| picture y position (pixel)|target x position (pixel)| target y position (pixel)| image #|\n",
+ "|-------|--------------|---------------|-------|----------|---------|----------|--------|\n",
+ "|arbitrary hit #|centered x position|centered y position|absolute x pixel location|absoluter y pixel location|target center x pixel location|target center y pixel location |either 1 or 2 for target_01.jpg or target_02.jpg|\n",
+ "\n"
]
},
{
"cell_type": "code",
- "execution_count": 17,
+ "execution_count": 43,
"metadata": {},
- "outputs": [
- {
- "data": {
- "text/plain": [
- "123.56215213358072"
- ]
- },
- "execution_count": 17,
- "metadata": {},
- "output_type": "execute_result"
- }
- ],
+ "outputs": [],
"source": [
- "gap_lines = y_lines[1:] - y_lines[0:-1]\n",
- "gap_lines.mean()"
+ "hits1=np.load('../data/hits_im1.npy')\n",
+ "hits2=np.load('../data/hits_im2.npy')\n",
+ "file = open('../data/target_data.csv','w')\n",
+ "file.write('throw #, x position (m), y position (m),')\n",
+ "file.write('picture x position (pixel), picture y position (pixel),')\n",
+ "file.write('target x position (pixel), target y position (pixel), image #\\n')\n",
+ "for i in range(0,len(scaled_hits1)):\n",
+ " file.write('{},{},{},'.format(i,scaled_hits1[i,0],scaled_hits1[i,1]))\n",
+ " file.write('{},{},'.format(hits1[i,0],hits1[i,1]))\n",
+ " file.write('{},{},{}\\n'.format(center1_x,center1_y,1))\n",
+ "for i in range(0,len(scaled_hits2)):\n",
+ " file.write('{},{},{},'.format(len(scaled_hits1)+i,scaled_hits2[i,0],scaled_hits2[i,1]))\n",
+ " file.write('{},{},'.format(hits2[i,0],hits2[i,1]))\n",
+ " file.write('{},{},{}\\n'.format(center2_x,center2_y,2))\n",
+ "file.close()"
]
},
{
- "cell_type": "code",
- "execution_count": null,
- "metadata": {},
- "outputs": [],
- "source": []
- },
- {
- "cell_type": "code",
- "execution_count": null,
- "metadata": {},
- "outputs": [],
- "source": []
- },
- {
- "cell_type": "code",
- "execution_count": null,
- "metadata": {},
- "outputs": [],
- "source": []
- },
- {
- "cell_type": "code",
- "execution_count": null,
+ "cell_type": "markdown",
"metadata": {},
- "outputs": [],
- "source": []
+ "source": [
+ "# Congratulations\n",
+ "\n",
+ "Now you can do some statistics on accuracy and precision of the class by loading the csv into a `pandas` dataframe. "
+ ]
}
],
"metadata": {
diff --git a/project/.ipynb_checkpoints/target_data-checkpoint.csv b/project/.ipynb_checkpoints/target_data-checkpoint.csv
new file mode 100644
index 0000000..0ac62c8
--- /dev/null
+++ b/project/.ipynb_checkpoints/target_data-checkpoint.csv
@@ -0,0 +1,55 @@
+throw #, x position (m), y position (m),picture x position (pixel), picture y position (pixel),target x position (pixel), target y position (pixel), image #
+0,-0.45718396926227894,-0.2956137183449811,1260.8551136363637,990.5994318181815,2042.2187500000005,1495.826704545454,1
+1,-0.19867156779460304,-0.4392317191603567,1702.6732954545455,745.144886363636,2042.2187500000005,1495.826704545454,1
+2,-0.08377716714230256,-0.44880625254804846,1899.036931818182,728.7812499999995,2042.2187500000005,1495.826704545454,1
+3,0.16037343424383593,-0.5014661861803528,2316.3096590909095,638.7812499999995,2042.2187500000005,1495.826704545454,1
+4,0.37101316877305335,-0.5876369866695782,2676.3096590909095,491.50852272727207,2042.2187500000005,1495.826704545454,1
+5,-0.1125007673053776,-0.21901745124344776,1849.946022727273,1121.508522727272,2042.2187500000005,1495.826704545454,1
+6,-0.08377716714230256,-0.2477410514065228,1899.036931818182,1072.417613636363,2042.2187500000005,1495.826704545454,1
+7,-0.0885644338361483,-0.17114478430498917,1890.855113636364,1203.326704545454,2042.2187500000005,1495.826704545454,1
+8,-0.1843097677130655,-0.03710131687730526,1727.21875,1432.4176136363633,2042.2187500000005,1495.826704545454,1
+9,-0.07420263375461078,-0.017952250101921842,1915.4005681818185,1465.144886363636,2042.2187500000005,1495.826704545454,1
+10,0.16994796763152745,-0.30518825173267294,2332.6732954545455,974.235795454545,2042.2187500000005,1495.826704545454,1
+11,0.2561187681207529,-0.32433731850805647,2479.946022727273,941.5085227272721,2042.2187500000005,1495.826704545454,1
+12,0.447609435874587,-0.3626354520588233,2807.2187500000005,876.0539772727266,2042.2187500000005,1495.826704545454,1
+13,0.04547903359153546,0.0011968166734615715,2119.946022727273,1497.8721590909088,2042.2187500000005,1495.826704545454,1
+14,0.08377716714230228,-0.051463116958842786,2185.4005681818185,1407.8721590909088,2042.2187500000005,1495.826704545454,1
+15,0.1412243674684524,-0.06582491704038045,2283.5823863636365,1383.326704545454,2042.2187500000005,1495.826704545454,1
+16,0.1412243674684524,-0.1519957175296056,2283.5823863636365,1236.053977272727,2042.2187500000005,1495.826704545454,1
+17,0.16994796763152745,-0.013164983408076088,2332.6732954545455,1473.326704545454,2042.2187500000005,1495.826704545454,1
+18,0.2848423682838279,-0.06582491704038045,2529.036931818182,1383.326704545454,2042.2187500000005,1495.826704545454,1
+19,0.3087787017530572,-0.08976125050960974,2569.946022727273,1342.417613636363,2042.2187500000005,1495.826704545454,1
+20,0.21782063456998604,0.1017294172442244,2414.4914772727275,1669.6903409090905,2042.2187500000005,1495.826704545454,1
+21,0.3375023019161323,0.1639638842642205,2619.036931818182,1776.053977272727,2042.2187500000005,1495.826704545454,1
+22,0.12207530069306911,0.2309856179780624,2250.855113636364,1890.5994318181815,2042.2187500000005,1495.826704545454,1
+23,0.1412243674684524,0.3458800186303629,2283.5823863636365,2086.963068181818,2042.2187500000005,1495.826704545454,1
+24,-0.14601163416229868,0.19268748442729558,1792.6732954545455,1825.144886363636,2042.2187500000005,1495.826704545454,1
+25,-0.16516070093768195,0.1639638842642205,1759.946022727273,1776.053977272727,2042.2187500000005,1495.826704545454,1
+26,-0.299204168365366,0.14960208418268287,1530.8551136363635,1751.5085227272723,2042.2187500000005,1495.826704545454,1
+27,-0.3039914350592117,0.37939088548728367,1522.6732954545455,2144.235795454545,2042.2187500000005,1495.826704545454,1
+28,-0.48112030273150824,0.403327218956513,1219.9460227272727,2185.144886363636,2042.2187500000005,1495.826704545454,1
+29,-0.7013345706484174,0.3410927519365171,843.5823863636363,2078.78125,2042.2187500000005,1495.826704545454,1
+30,-0.28905801786881596,-0.12698486845025021412.21875,1254.97443181818152017.9005681818185,1521.0539772727275,2
+31,-0.12505962274024188,-0.30269743465943671755.855113636364,886.79261363636312017.9005681818185,1521.0539772727275,2
+32,-0.19534464922391653,-0.158222657998551608.5823863636365,1189.5198863636362017.9005681818185,1521.0539772727275,2
+33,-0.23048716246575374,-0.076223460434263191534.946022727273,1361.33806818181762017.9005681818185,1521.0539772727275,2
+34,-0.11725017535316709,-0.044985670885963261772.21875,1426.79261363636332017.9005681818185,1521.0539772727275,2
+35,-0.07039349103071725,-0.107461249982562791870.4005681818185,1295.88352272727252017.9005681818185,1521.0539772727275,2
+36,-0.04306042517595505,-0.189460447546849851927.6732954545455,1124.06534090909052017.9005681818185,1521.0539772727275,2
+37,0.01160570653356958,-0.14650848691793762042.21875,1214.06534090909052017.9005681818185,1521.0539772727275,2
+38,0.038938772388332,-0.31441160574004912099.4914772727275,862.24715909090862017.9005681818185,1521.0539772727275,2
+39,0.09360490409785663,-0.130889592143787812214.036931818182,1246.7926136363632017.9005681818185,1521.0539772727275,2
+40,0.16779465427506868,-0.169936829079162442369.4914772727275,1164.97443181818152017.9005681818185,1521.0539772727275,2
+41,0.2263655096781309,-0.244126579256374462492.2187500000005,1009.5198863636362017.9005681818185,1521.0539772727275,2
+42,0.6012189842577286,-0.31831632943358653277.673295454546,854.06534090909052017.9005681818185,1521.0539772727275,2
+43,0.3044599835488804,-0.0137478813376634392655.855113636364,1492.24715909090882017.9005681818185,1521.0539772727275,2
+44,0.1951277201298311,-0.068414013047188182426.764204545455,1377.7017045454542017.9005681818185,1521.0539772727275,2
+45,0.11703324625908144,-0.0371762234988883562263.127840909091,1443.15624999999952017.9005681818185,1521.0539772727275,2
+46,0.01941515392064459,0.087774934694310912058.5823863636365,1704.97443181818152017.9005681818185,1521.0539772727275,2
+47,0.16388993058153128,0.099489105774923332361.3096590909095,1729.5198863636362017.9005681818185,1521.0539772727275,2
+48,0.2888410887747305,0.165869408565060362623.1278409090914,1868.6107954545452017.9005681818185,1521.0539772727275,2
+49,0.523124510386979,0.06825131622662353114.0369318181824,1664.06534090909052017.9005681818185,1521.0539772727275,2
+50,-0.12505962274024188,0.36110559324193411755.855113636364,2277.7017045454542017.9005681818185,1521.0539772727275,2
+51,-0.24220133354636614,0.40015283017730891510.4005681818185,2359.5198863636362017.9005681818185,1521.0539772727275,2
+52,-0.21096354399806633,0.56024650161234531575.855113636364,2694.97443181818152017.9005681818185,1521.0539772727275,2
+53,-0.23048716246575374,0.286915843064722231534.946022727273,2122.2471590909092017.9005681818185,1521.0539772727275,2
diff --git a/project/02_Analyze-data_project.ipynb b/project/02_Analyze-data_project.ipynb
index ce6abc8..9b149ff 100644
--- a/project/02_Analyze-data_project.ipynb
+++ b/project/02_Analyze-data_project.ipynb
@@ -4,9 +4,9 @@
"cell_type": "markdown",
"metadata": {},
"source": [
- "# Computational Mechanics Project #02 - Create specifications for a spitballing robot\n",
+ "# Computational Mechanics Project #02 - Create specifications for a projectile robot\n",
"\n",
- "On the first day of class, we threw $2\"\\times~2\"$ dampened paper (spitballs) at a target on the whiteboard. Now, we are going to analyze the accuracy of the class with some cool Python tools and design a robot that has the same accuracy and precision as the class. \n",
+ "On the first day of class, we threw $2\"\\times~2\"$ dampened paper (spitballs) at a target on the whiteboard. Now, we are going to analyze the accuracy of the class with some cool Python tools and design a robot that has the same accuracy and precision as the class, but we will have the robot move farther away from the target and use a simpler projectile i.e. a tennis ball so we don't need to worry about knuckle-ball physics. \n",
"\n",
"The goal of this project is to determine the precision of necessary components for a robot that can reproduce the class throwing distibution. We have generated pseudo random numbers using `numpy.random`, but the class target practice is an example of truly random distributions. If we repeated the exercise, there is a vanishingly small probability that we would hit the same points on the target, and there are no deterministic models that could take into account all of the factors that affected each hit on the board. \n",
"\n",
@@ -69,7 +69,7 @@
"source": [
"# Project Deliverables\n",
"\n",
- "1. Statistical analysis of class accuracy and precision (x- and z-locations)\n",
+ "1. Statistical analysis of class accuracy and precision (x- and z-locations) data is in the csv file [../data/target_data.csv](../data/target_data.csv) _Note: if you want to see how I turned the images into data check out the jupyter notebook [process_target_practice](./process_target_practice.ipynb)\n",
"\n",
"2. A Monte Carlo model to generate impact heights based upon uncertainty in $\\theta_0$ and $v_0$. \n",
"\n",
diff --git a/project/Cooper_data/hits_im1.npy b/project/Cooper_data/hits_im1.npy
new file mode 100644
index 0000000..f808cdd
Binary files /dev/null and b/project/Cooper_data/hits_im1.npy differ
diff --git a/project/Cooper_data/hits_im2.npy b/project/Cooper_data/hits_im2.npy
new file mode 100644
index 0000000..445030c
Binary files /dev/null and b/project/Cooper_data/hits_im2.npy differ
diff --git a/project/Cooper_data/target_data.csv b/project/Cooper_data/target_data.csv
new file mode 100644
index 0000000..77a57ba
--- /dev/null
+++ b/project/Cooper_data/target_data.csv
@@ -0,0 +1,55 @@
+throw #, x position (m), y position (m),picture x position (pixel), picture y position (pixel),target x position (pixel), target y position (pixel), image #
+0,-0.4664032892922447,-0.3040002885922146,1260.8551136363637,990.5994318181815,2055.1692559776675,1508.3310474572158,1
+1,-0.20697765114141842,-0.4481256431204515,1702.6732954545455,745.144886363636,2055.1692559776675,1508.3310474572158,1
+2,-0.09167736751882886,-0.45773400008900067,1899.036931818182,728.7812499999995,2055.1692559776675,1508.3310474572158,1
+3,0.1533357351791739,-0.5105799634160209,2316.3096590909095,638.7812499999995,2055.1692559776675,1508.3310474572158,1
+4,0.3647195884872546,-0.5970551761329631,2676.3096590909095,491.50852272727207,2055.1692559776675,1508.3310474572158,1
+5,-0.1205024384244762,-0.22713343284382184,1849.946022727273,1121.508522727272,2055.1692559776675,1508.3310474572158,1
+6,-0.09167736751882886,-0.25595850374946916,1899.036931818182,1072.417613636363,2055.1692559776675,1508.3310474572158,1
+7,-0.09648154600310332,-0.17909164800107613,1890.855113636364,1203.326704545454,2055.1692559776675,1508.3310474572158,1
+8,-0.19256511568859477,-0.04457465044138831,1727.21875,1432.4176136363633,2055.1692559776675,1508.3310474572158,1
+9,-0.08206901055027967,-0.02535793650429005,1915.4005681818185,1465.144886363636,2055.1692559776675,1508.3310474572158,1
+10,0.16294409214772285,-0.3136086455607638,2332.6732954545455,974.235795454545,2055.1692559776675,1508.3310474572158,1
+11,0.24941930486466507,-0.3328253594978622,2479.946022727273,941.5085227272721,2055.1692559776675,1508.3310474572158,1
+12,0.4415864442356477,-0.3712587873720587,2807.2187500000005,876.0539772727266,2055.1692559776675,1508.3310474572158,1
+13,0.03803545155658435,-0.00614122256719179,2119.946022727273,1497.8721590909088,2055.1692559776675,1508.3310474572158,1
+14,0.07646887943078087,-0.05898718589421197,2185.4005681818185,1407.8721590909088,2055.1692559776675,1508.3310474572158,1
+15,0.1341190212420755,-0.07339972134703576,2283.5823863636365,1383.326704545454,2055.1692559776675,1508.3310474572158,1
+16,0.1341190212420755,-0.15987493406397774,2283.5823863636365,1236.053977272727,2055.1692559776675,1508.3310474572158,1
+17,0.16294409214772285,-0.020553758020015585,2332.6732954545455,1473.326704545454,2055.1692559776675,1508.3310474572158,1
+18,0.2782443757703124,-0.07339972134703576,2529.036931818182,1383.326704545454,2055.1692559776675,1508.3310474572158,1
+19,0.30226526819168525,-0.09742061376840862,2569.946022727273,1342.417613636363,2055.1692559776675,1508.3310474572158,1
+20,0.21098587699046856,0.09474652560257397,2414.4914772727275,1669.6903409090905,2055.1692559776675,1508.3310474572158,1
+21,0.3310903390973326,0.15720084589814334,2619.036931818182,1776.053977272727,2055.1692559776675,1508.3310474572158,1
+22,0.11490230730497739,0.2244593446779872,2250.855113636364,1890.5994318181815,2055.1692559776675,1508.3310474572158,1
+23,0.1341190212420755,0.33975962830057677,2283.5823863636365,2086.963068181818,2055.1692559776675,1508.3310474572158,1
+24,-0.15413168781439823,0.18602591680379066,1792.6732954545455,1825.144886363636,2055.1692559776675,1508.3310474572158,1
+25,-0.17334840175149638,0.15720084589814334,1759.946022727273,1776.053977272727,2055.1692559776675,1508.3310474572158,1
+26,-0.30786539931118434,0.14278831044531956,1530.8551136363635,1751.5085227272723,2055.1692559776675,1508.3310474572158,1
+27,-0.3126695777954588,0.37338887769049856,1522.6732954545455,2144.235795454545,2055.1692559776675,1508.3310474572158,1
+28,-0.49042418171361757,0.3974097701118714,1219.9460227272727,2185.144886363636,2055.1692559776675,1508.3310474572158,1
+29,-0.7114163919902475,0.33495544981630226,843.5823863636363,2078.78125,2055.1692559776675,1508.3310474572158,1
+30,-0.29131239559921246,-0.12719786197191166,1412.21875,1254.9744318181815,2019.26155624037,1520.031972265023,2
+31,-0.12640552037257052,-0.3038837997147423,1755.855113636364,886.7926136363631,2019.26155624037,1520.031972265023,2
+32,-0.1970798954697028,-0.15860869534841487,1608.5823863636365,1189.519886363636,2019.26155624037,1520.031972265023,2
+33,-0.23241708301826883,-0.07615525773509413,1534.946022727273,1361.3380681818176,2019.26155624037,1520.031972265023,2
+34,-0.11855281202844486,-0.0447444243585908,1772.21875,1426.7926136363633,2019.26155624037,1520.031972265023,2
+35,-0.07143656196368994,-0.10756609111159712,1870.4005681818185,1295.8835227272725,2019.26155624037,1520.031972265023,2
+36,-0.04395208275924976,-0.1900195287249181,1927.6732954545455,1124.0653409090905,2019.26155624037,1520.031972265023,2
+37,0.011016875649630815,-0.1468296328322262,2042.21875,1214.0653409090905,2019.26155624037,1520.031972265023,2
+38,0.03850135485407121,-0.315662862230931,2099.4914772727275,862.2471590909086,2019.26155624037,1520.031972265023,2
+39,0.09347031326295178,-0.1311242161439747,2214.036931818182,1246.792613636363,2019.26155624037,1520.031972265023,2
+40,0.16807104253214689,-0.17038775786460356,2369.4914772727275,1164.9744318181815,2019.26155624037,1520.031972265023,2
+41,0.22696635511309052,-0.24498848713379867,2492.2187500000005,1009.519886363636,2019.26155624037,1520.031972265023,2
+42,0.6038963556311289,-0.31958921640299376,3277.673295454546,854.0653409090905,2019.26155624037,1520.031972265023,2
+43,0.30549343855434846,-0.013333590982087582,2655.855113636364,1492.2471590909088,2019.26155624037,1520.031972265023,2
+44,0.1955555217365873,-0.06830254939096826,2426.764204545455,1377.701704545454,2019.26155624037,1520.031972265023,2
+45,0.11702843829532915,-0.03689171601446505,2263.127840909091,1443.1562499999995,2019.26155624037,1520.031972265023,2
+46,0.018869583993756673,0.08875161749154782,2058.5823863636365,1704.9744318181815,2019.26155624037,1520.031972265023,2
+47,0.16414468836008408,0.10053068000773649,2361.3096590909095,1729.519886363636,2019.26155624037,1520.031972265023,2
+48,0.2897880218660969,0.16727870093280575,2623.1278409090914,1868.610795454545,2019.26155624037,1520.031972265023,2
+49,0.525369272189871,0.06911984663123327,3114.0369318181824,1664.0653409090905,2019.26155624037,1520.031972265023,2
+50,-0.12640552037257052,0.3635964095359507,1755.855113636364,2277.701704545454,2019.26155624037,1520.031972265023,2
+51,-0.2441961455344575,0.40285995125657975,1510.4005681818185,2359.519886363636,2019.26155624037,1520.031972265023,2
+52,-0.2127853121579543,0.5638404723111586,1575.855113636364,2694.9744318181815,2019.26155624037,1520.031972265023,2
+53,-0.23241708301826883,0.2889956802667558,1534.946022727273,2122.247159090909,2019.26155624037,1520.031972265023,2
diff --git a/project/process_target_practice.ipynb b/project/process_target_practice.ipynb
index 55dacd5..ce8d8be 100644
--- a/project/process_target_practice.ipynb
+++ b/project/process_target_practice.ipynb
@@ -1,20 +1,1749 @@
{
"cells": [
+ {
+ "cell_type": "markdown",
+ "metadata": {},
+ "source": [
+ "# Creating quantitative data from an image\n",
+ "\n",
+ "In this _preview_ notebook, we will generate the location of board hits by clicking on an image displayed by matplotlib. We will repeat this process in the next Module_03-IVPs. \n",
+ "\n",
+ "The first step is to import the libraries we need, the new functions here are \n",
+ "\n",
+ "- `imread`: a function that creates a numpy array that can be plotted with plt.imshow\n",
+ "\n",
+ "- `%matplotlib notebook`: is a \"magic\" command that allows for an interactive matplotlib figure. There's one extra plotting step of creating a figure before plotting with `plt.figure()`"
+ ]
+ },
+ {
+ "cell_type": "code",
+ "execution_count": 6,
+ "metadata": {},
+ "outputs": [],
+ "source": [
+ "import matplotlib.pyplot as plt\n",
+ "from matplotlib.image import imread\n",
+ "import numpy as np\n",
+ "%matplotlib notebook"
+ ]
+ },
+ {
+ "cell_type": "markdown",
+ "metadata": {},
+ "source": [
+ "# Read in images target_01 and target_02\n",
+ "\n",
+ "Now we read in target_01.jpg and target_02.jpg into variables `im1` and `im2`. These are the images of the whiteboard. The black lines are perpendicular to each other and 36\" (0.9144 m) long. We'll display `im1` to start."
+ ]
+ },
+ {
+ "cell_type": "code",
+ "execution_count": 7,
+ "metadata": {},
+ "outputs": [
+ {
+ "data": {
+ "application/javascript": [
+ "/* Put everything inside the global mpl namespace */\n",
+ "window.mpl = {};\n",
+ "\n",
+ "\n",
+ "mpl.get_websocket_type = function() {\n",
+ " if (typeof(WebSocket) !== 'undefined') {\n",
+ " return WebSocket;\n",
+ " } else if (typeof(MozWebSocket) !== 'undefined') {\n",
+ " return MozWebSocket;\n",
+ " } else {\n",
+ " alert('Your browser does not have WebSocket support. ' +\n",
+ " 'Please try Chrome, Safari or Firefox ≥ 6. ' +\n",
+ " 'Firefox 4 and 5 are also supported but you ' +\n",
+ " 'have to enable WebSockets in about:config.');\n",
+ " };\n",
+ "}\n",
+ "\n",
+ "mpl.figure = function(figure_id, websocket, ondownload, parent_element) {\n",
+ " this.id = figure_id;\n",
+ "\n",
+ " this.ws = websocket;\n",
+ "\n",
+ " this.supports_binary = (this.ws.binaryType != undefined);\n",
+ "\n",
+ " if (!this.supports_binary) {\n",
+ " var warnings = document.getElementById(\"mpl-warnings\");\n",
+ " if (warnings) {\n",
+ " warnings.style.display = 'block';\n",
+ " warnings.textContent = (\n",
+ " \"This browser does not support binary websocket messages. \" +\n",
+ " \"Performance may be slow.\");\n",
+ " }\n",
+ " }\n",
+ "\n",
+ " this.imageObj = new Image();\n",
+ "\n",
+ " this.context = undefined;\n",
+ " this.message = undefined;\n",
+ " this.canvas = undefined;\n",
+ " this.rubberband_canvas = undefined;\n",
+ " this.rubberband_context = undefined;\n",
+ " this.format_dropdown = undefined;\n",
+ "\n",
+ " this.image_mode = 'full';\n",
+ "\n",
+ " this.root = $('');\n",
+ " this._root_extra_style(this.root)\n",
+ " this.root.attr('style', 'display: inline-block');\n",
+ "\n",
+ " $(parent_element).append(this.root);\n",
+ "\n",
+ " this._init_header(this);\n",
+ " this._init_canvas(this);\n",
+ " this._init_toolbar(this);\n",
+ "\n",
+ " var fig = this;\n",
+ "\n",
+ " this.waiting = false;\n",
+ "\n",
+ " this.ws.onopen = function () {\n",
+ " fig.send_message(\"supports_binary\", {value: fig.supports_binary});\n",
+ " fig.send_message(\"send_image_mode\", {});\n",
+ " if (mpl.ratio != 1) {\n",
+ " fig.send_message(\"set_dpi_ratio\", {'dpi_ratio': mpl.ratio});\n",
+ " }\n",
+ " fig.send_message(\"refresh\", {});\n",
+ " }\n",
+ "\n",
+ " this.imageObj.onload = function() {\n",
+ " if (fig.image_mode == 'full') {\n",
+ " // Full images could contain transparency (where diff images\n",
+ " // almost always do), so we need to clear the canvas so that\n",
+ " // there is no ghosting.\n",
+ " fig.context.clearRect(0, 0, fig.canvas.width, fig.canvas.height);\n",
+ " }\n",
+ " fig.context.drawImage(fig.imageObj, 0, 0);\n",
+ " };\n",
+ "\n",
+ " this.imageObj.onunload = function() {\n",
+ " fig.ws.close();\n",
+ " }\n",
+ "\n",
+ " this.ws.onmessage = this._make_on_message_function(this);\n",
+ "\n",
+ " this.ondownload = ondownload;\n",
+ "}\n",
+ "\n",
+ "mpl.figure.prototype._init_header = function() {\n",
+ " var titlebar = $(\n",
+ " '');\n",
+ " var titletext = $(\n",
+ " '');\n",
+ " titlebar.append(titletext)\n",
+ " this.root.append(titlebar);\n",
+ " this.header = titletext[0];\n",
+ "}\n",
+ "\n",
+ "\n",
+ "\n",
+ "mpl.figure.prototype._canvas_extra_style = function(canvas_div) {\n",
+ "\n",
+ "}\n",
+ "\n",
+ "\n",
+ "mpl.figure.prototype._root_extra_style = function(canvas_div) {\n",
+ "\n",
+ "}\n",
+ "\n",
+ "mpl.figure.prototype._init_canvas = function() {\n",
+ " var fig = this;\n",
+ "\n",
+ " var canvas_div = $('');\n",
+ "\n",
+ " canvas_div.attr('style', 'position: relative; clear: both; outline: 0');\n",
+ "\n",
+ " function canvas_keyboard_event(event) {\n",
+ " return fig.key_event(event, event['data']);\n",
+ " }\n",
+ "\n",
+ " canvas_div.keydown('key_press', canvas_keyboard_event);\n",
+ " canvas_div.keyup('key_release', canvas_keyboard_event);\n",
+ " this.canvas_div = canvas_div\n",
+ " this._canvas_extra_style(canvas_div)\n",
+ " this.root.append(canvas_div);\n",
+ "\n",
+ " var canvas = $('');\n",
+ " canvas.addClass('mpl-canvas');\n",
+ " canvas.attr('style', \"left: 0; top: 0; z-index: 0; outline: 0\")\n",
+ "\n",
+ " this.canvas = canvas[0];\n",
+ " this.context = canvas[0].getContext(\"2d\");\n",
+ "\n",
+ " var backingStore = this.context.backingStorePixelRatio ||\n",
+ "\tthis.context.webkitBackingStorePixelRatio ||\n",
+ "\tthis.context.mozBackingStorePixelRatio ||\n",
+ "\tthis.context.msBackingStorePixelRatio ||\n",
+ "\tthis.context.oBackingStorePixelRatio ||\n",
+ "\tthis.context.backingStorePixelRatio || 1;\n",
+ "\n",
+ " mpl.ratio = (window.devicePixelRatio || 1) / backingStore;\n",
+ "\n",
+ " var rubberband = $('');\n",
+ " rubberband.attr('style', \"position: absolute; left: 0; top: 0; z-index: 1;\")\n",
+ "\n",
+ " var pass_mouse_events = true;\n",
+ "\n",
+ " canvas_div.resizable({\n",
+ " start: function(event, ui) {\n",
+ " pass_mouse_events = false;\n",
+ " },\n",
+ " resize: function(event, ui) {\n",
+ " fig.request_resize(ui.size.width, ui.size.height);\n",
+ " },\n",
+ " stop: function(event, ui) {\n",
+ " pass_mouse_events = true;\n",
+ " fig.request_resize(ui.size.width, ui.size.height);\n",
+ " },\n",
+ " });\n",
+ "\n",
+ " function mouse_event_fn(event) {\n",
+ " if (pass_mouse_events)\n",
+ " return fig.mouse_event(event, event['data']);\n",
+ " }\n",
+ "\n",
+ " rubberband.mousedown('button_press', mouse_event_fn);\n",
+ " rubberband.mouseup('button_release', mouse_event_fn);\n",
+ " // Throttle sequential mouse events to 1 every 20ms.\n",
+ " rubberband.mousemove('motion_notify', mouse_event_fn);\n",
+ "\n",
+ " rubberband.mouseenter('figure_enter', mouse_event_fn);\n",
+ " rubberband.mouseleave('figure_leave', mouse_event_fn);\n",
+ "\n",
+ " canvas_div.on(\"wheel\", function (event) {\n",
+ " event = event.originalEvent;\n",
+ " event['data'] = 'scroll'\n",
+ " if (event.deltaY < 0) {\n",
+ " event.step = 1;\n",
+ " } else {\n",
+ " event.step = -1;\n",
+ " }\n",
+ " mouse_event_fn(event);\n",
+ " });\n",
+ "\n",
+ " canvas_div.append(canvas);\n",
+ " canvas_div.append(rubberband);\n",
+ "\n",
+ " this.rubberband = rubberband;\n",
+ " this.rubberband_canvas = rubberband[0];\n",
+ " this.rubberband_context = rubberband[0].getContext(\"2d\");\n",
+ " this.rubberband_context.strokeStyle = \"#000000\";\n",
+ "\n",
+ " this._resize_canvas = function(width, height) {\n",
+ " // Keep the size of the canvas, canvas container, and rubber band\n",
+ " // canvas in synch.\n",
+ " canvas_div.css('width', width)\n",
+ " canvas_div.css('height', height)\n",
+ "\n",
+ " canvas.attr('width', width * mpl.ratio);\n",
+ " canvas.attr('height', height * mpl.ratio);\n",
+ " canvas.attr('style', 'width: ' + width + 'px; height: ' + height + 'px;');\n",
+ "\n",
+ " rubberband.attr('width', width);\n",
+ " rubberband.attr('height', height);\n",
+ " }\n",
+ "\n",
+ " // Set the figure to an initial 600x600px, this will subsequently be updated\n",
+ " // upon first draw.\n",
+ " this._resize_canvas(600, 600);\n",
+ "\n",
+ " // Disable right mouse context menu.\n",
+ " $(this.rubberband_canvas).bind(\"contextmenu\",function(e){\n",
+ " return false;\n",
+ " });\n",
+ "\n",
+ " function set_focus () {\n",
+ " canvas.focus();\n",
+ " canvas_div.focus();\n",
+ " }\n",
+ "\n",
+ " window.setTimeout(set_focus, 100);\n",
+ "}\n",
+ "\n",
+ "mpl.figure.prototype._init_toolbar = function() {\n",
+ " var fig = this;\n",
+ "\n",
+ " var nav_element = $('');\n",
+ " nav_element.attr('style', 'width: 100%');\n",
+ " this.root.append(nav_element);\n",
+ "\n",
+ " // Define a callback function for later on.\n",
+ " function toolbar_event(event) {\n",
+ " return fig.toolbar_button_onclick(event['data']);\n",
+ " }\n",
+ " function toolbar_mouse_event(event) {\n",
+ " return fig.toolbar_button_onmouseover(event['data']);\n",
+ " }\n",
+ "\n",
+ " for(var toolbar_ind in mpl.toolbar_items) {\n",
+ " var name = mpl.toolbar_items[toolbar_ind][0];\n",
+ " var tooltip = mpl.toolbar_items[toolbar_ind][1];\n",
+ " var image = mpl.toolbar_items[toolbar_ind][2];\n",
+ " var method_name = mpl.toolbar_items[toolbar_ind][3];\n",
+ "\n",
+ " if (!name) {\n",
+ " // put a spacer in here.\n",
+ " continue;\n",
+ " }\n",
+ " var button = $('');\n",
+ " button.addClass('ui-button ui-widget ui-state-default ui-corner-all ' +\n",
+ " 'ui-button-icon-only');\n",
+ " button.attr('role', 'button');\n",
+ " button.attr('aria-disabled', 'false');\n",
+ " button.click(method_name, toolbar_event);\n",
+ " button.mouseover(tooltip, toolbar_mouse_event);\n",
+ "\n",
+ " var icon_img = $('');\n",
+ " icon_img.addClass('ui-button-icon-primary ui-icon');\n",
+ " icon_img.addClass(image);\n",
+ " icon_img.addClass('ui-corner-all');\n",
+ "\n",
+ " var tooltip_span = $('');\n",
+ " tooltip_span.addClass('ui-button-text');\n",
+ " tooltip_span.html(tooltip);\n",
+ "\n",
+ " button.append(icon_img);\n",
+ " button.append(tooltip_span);\n",
+ "\n",
+ " nav_element.append(button);\n",
+ " }\n",
+ "\n",
+ " var fmt_picker_span = $('');\n",
+ "\n",
+ " var fmt_picker = $('');\n",
+ " fmt_picker.addClass('mpl-toolbar-option ui-widget ui-widget-content');\n",
+ " fmt_picker_span.append(fmt_picker);\n",
+ " nav_element.append(fmt_picker_span);\n",
+ " this.format_dropdown = fmt_picker[0];\n",
+ "\n",
+ " for (var ind in mpl.extensions) {\n",
+ " var fmt = mpl.extensions[ind];\n",
+ " var option = $(\n",
+ " '', {selected: fmt === mpl.default_extension}).html(fmt);\n",
+ " fmt_picker.append(option);\n",
+ " }\n",
+ "\n",
+ " // Add hover states to the ui-buttons\n",
+ " $( \".ui-button\" ).hover(\n",
+ " function() { $(this).addClass(\"ui-state-hover\");},\n",
+ " function() { $(this).removeClass(\"ui-state-hover\");}\n",
+ " );\n",
+ "\n",
+ " var status_bar = $('');\n",
+ " nav_element.append(status_bar);\n",
+ " this.message = status_bar[0];\n",
+ "}\n",
+ "\n",
+ "mpl.figure.prototype.request_resize = function(x_pixels, y_pixels) {\n",
+ " // Request matplotlib to resize the figure. Matplotlib will then trigger a resize in the client,\n",
+ " // which will in turn request a refresh of the image.\n",
+ " this.send_message('resize', {'width': x_pixels, 'height': y_pixels});\n",
+ "}\n",
+ "\n",
+ "mpl.figure.prototype.send_message = function(type, properties) {\n",
+ " properties['type'] = type;\n",
+ " properties['figure_id'] = this.id;\n",
+ " this.ws.send(JSON.stringify(properties));\n",
+ "}\n",
+ "\n",
+ "mpl.figure.prototype.send_draw_message = function() {\n",
+ " if (!this.waiting) {\n",
+ " this.waiting = true;\n",
+ " this.ws.send(JSON.stringify({type: \"draw\", figure_id: this.id}));\n",
+ " }\n",
+ "}\n",
+ "\n",
+ "\n",
+ "mpl.figure.prototype.handle_save = function(fig, msg) {\n",
+ " var format_dropdown = fig.format_dropdown;\n",
+ " var format = format_dropdown.options[format_dropdown.selectedIndex].value;\n",
+ " fig.ondownload(fig, format);\n",
+ "}\n",
+ "\n",
+ "\n",
+ "mpl.figure.prototype.handle_resize = function(fig, msg) {\n",
+ " var size = msg['size'];\n",
+ " if (size[0] != fig.canvas.width || size[1] != fig.canvas.height) {\n",
+ " fig._resize_canvas(size[0], size[1]);\n",
+ " fig.send_message(\"refresh\", {});\n",
+ " };\n",
+ "}\n",
+ "\n",
+ "mpl.figure.prototype.handle_rubberband = function(fig, msg) {\n",
+ " var x0 = msg['x0'] / mpl.ratio;\n",
+ " var y0 = (fig.canvas.height - msg['y0']) / mpl.ratio;\n",
+ " var x1 = msg['x1'] / mpl.ratio;\n",
+ " var y1 = (fig.canvas.height - msg['y1']) / mpl.ratio;\n",
+ " x0 = Math.floor(x0) + 0.5;\n",
+ " y0 = Math.floor(y0) + 0.5;\n",
+ " x1 = Math.floor(x1) + 0.5;\n",
+ " y1 = Math.floor(y1) + 0.5;\n",
+ " var min_x = Math.min(x0, x1);\n",
+ " var min_y = Math.min(y0, y1);\n",
+ " var width = Math.abs(x1 - x0);\n",
+ " var height = Math.abs(y1 - y0);\n",
+ "\n",
+ " fig.rubberband_context.clearRect(\n",
+ " 0, 0, fig.canvas.width / mpl.ratio, fig.canvas.height / mpl.ratio);\n",
+ "\n",
+ " fig.rubberband_context.strokeRect(min_x, min_y, width, height);\n",
+ "}\n",
+ "\n",
+ "mpl.figure.prototype.handle_figure_label = function(fig, msg) {\n",
+ " // Updates the figure title.\n",
+ " fig.header.textContent = msg['label'];\n",
+ "}\n",
+ "\n",
+ "mpl.figure.prototype.handle_cursor = function(fig, msg) {\n",
+ " var cursor = msg['cursor'];\n",
+ " switch(cursor)\n",
+ " {\n",
+ " case 0:\n",
+ " cursor = 'pointer';\n",
+ " break;\n",
+ " case 1:\n",
+ " cursor = 'default';\n",
+ " break;\n",
+ " case 2:\n",
+ " cursor = 'crosshair';\n",
+ " break;\n",
+ " case 3:\n",
+ " cursor = 'move';\n",
+ " break;\n",
+ " }\n",
+ " fig.rubberband_canvas.style.cursor = cursor;\n",
+ "}\n",
+ "\n",
+ "mpl.figure.prototype.handle_message = function(fig, msg) {\n",
+ " fig.message.textContent = msg['message'];\n",
+ "}\n",
+ "\n",
+ "mpl.figure.prototype.handle_draw = function(fig, msg) {\n",
+ " // Request the server to send over a new figure.\n",
+ " fig.send_draw_message();\n",
+ "}\n",
+ "\n",
+ "mpl.figure.prototype.handle_image_mode = function(fig, msg) {\n",
+ " fig.image_mode = msg['mode'];\n",
+ "}\n",
+ "\n",
+ "mpl.figure.prototype.updated_canvas_event = function() {\n",
+ " // Called whenever the canvas gets updated.\n",
+ " this.send_message(\"ack\", {});\n",
+ "}\n",
+ "\n",
+ "// A function to construct a web socket function for onmessage handling.\n",
+ "// Called in the figure constructor.\n",
+ "mpl.figure.prototype._make_on_message_function = function(fig) {\n",
+ " return function socket_on_message(evt) {\n",
+ " if (evt.data instanceof Blob) {\n",
+ " /* FIXME: We get \"Resource interpreted as Image but\n",
+ " * transferred with MIME type text/plain:\" errors on\n",
+ " * Chrome. But how to set the MIME type? It doesn't seem\n",
+ " * to be part of the websocket stream */\n",
+ " evt.data.type = \"image/png\";\n",
+ "\n",
+ " /* Free the memory for the previous frames */\n",
+ " if (fig.imageObj.src) {\n",
+ " (window.URL || window.webkitURL).revokeObjectURL(\n",
+ " fig.imageObj.src);\n",
+ " }\n",
+ "\n",
+ " fig.imageObj.src = (window.URL || window.webkitURL).createObjectURL(\n",
+ " evt.data);\n",
+ " fig.updated_canvas_event();\n",
+ " fig.waiting = false;\n",
+ " return;\n",
+ " }\n",
+ " else if (typeof evt.data === 'string' && evt.data.slice(0, 21) == \"data:image/png;base64\") {\n",
+ " fig.imageObj.src = evt.data;\n",
+ " fig.updated_canvas_event();\n",
+ " fig.waiting = false;\n",
+ " return;\n",
+ " }\n",
+ "\n",
+ " var msg = JSON.parse(evt.data);\n",
+ " var msg_type = msg['type'];\n",
+ "\n",
+ " // Call the \"handle_{type}\" callback, which takes\n",
+ " // the figure and JSON message as its only arguments.\n",
+ " try {\n",
+ " var callback = fig[\"handle_\" + msg_type];\n",
+ " } catch (e) {\n",
+ " console.log(\"No handler for the '\" + msg_type + \"' message type: \", msg);\n",
+ " return;\n",
+ " }\n",
+ "\n",
+ " if (callback) {\n",
+ " try {\n",
+ " // console.log(\"Handling '\" + msg_type + \"' message: \", msg);\n",
+ " callback(fig, msg);\n",
+ " } catch (e) {\n",
+ " console.log(\"Exception inside the 'handler_\" + msg_type + \"' callback:\", e, e.stack, msg);\n",
+ " }\n",
+ " }\n",
+ " };\n",
+ "}\n",
+ "\n",
+ "// from http://stackoverflow.com/questions/1114465/getting-mouse-location-in-canvas\n",
+ "mpl.findpos = function(e) {\n",
+ " //this section is from http://www.quirksmode.org/js/events_properties.html\n",
+ " var targ;\n",
+ " if (!e)\n",
+ " e = window.event;\n",
+ " if (e.target)\n",
+ " targ = e.target;\n",
+ " else if (e.srcElement)\n",
+ " targ = e.srcElement;\n",
+ " if (targ.nodeType == 3) // defeat Safari bug\n",
+ " targ = targ.parentNode;\n",
+ "\n",
+ " // jQuery normalizes the pageX and pageY\n",
+ " // pageX,Y are the mouse positions relative to the document\n",
+ " // offset() returns the position of the element relative to the document\n",
+ " var x = e.pageX - $(targ).offset().left;\n",
+ " var y = e.pageY - $(targ).offset().top;\n",
+ "\n",
+ " return {\"x\": x, \"y\": y};\n",
+ "};\n",
+ "\n",
+ "/*\n",
+ " * return a copy of an object with only non-object keys\n",
+ " * we need this to avoid circular references\n",
+ " * http://stackoverflow.com/a/24161582/3208463\n",
+ " */\n",
+ "function simpleKeys (original) {\n",
+ " return Object.keys(original).reduce(function (obj, key) {\n",
+ " if (typeof original[key] !== 'object')\n",
+ " obj[key] = original[key]\n",
+ " return obj;\n",
+ " }, {});\n",
+ "}\n",
+ "\n",
+ "mpl.figure.prototype.mouse_event = function(event, name) {\n",
+ " var canvas_pos = mpl.findpos(event)\n",
+ "\n",
+ " if (name === 'button_press')\n",
+ " {\n",
+ " this.canvas.focus();\n",
+ " this.canvas_div.focus();\n",
+ " }\n",
+ "\n",
+ " var x = canvas_pos.x * mpl.ratio;\n",
+ " var y = canvas_pos.y * mpl.ratio;\n",
+ "\n",
+ " this.send_message(name, {x: x, y: y, button: event.button,\n",
+ " step: event.step,\n",
+ " guiEvent: simpleKeys(event)});\n",
+ "\n",
+ " /* This prevents the web browser from automatically changing to\n",
+ " * the text insertion cursor when the button is pressed. We want\n",
+ " * to control all of the cursor setting manually through the\n",
+ " * 'cursor' event from matplotlib */\n",
+ " event.preventDefault();\n",
+ " return false;\n",
+ "}\n",
+ "\n",
+ "mpl.figure.prototype._key_event_extra = function(event, name) {\n",
+ " // Handle any extra behaviour associated with a key event\n",
+ "}\n",
+ "\n",
+ "mpl.figure.prototype.key_event = function(event, name) {\n",
+ "\n",
+ " // Prevent repeat events\n",
+ " if (name == 'key_press')\n",
+ " {\n",
+ " if (event.which === this._key)\n",
+ " return;\n",
+ " else\n",
+ " this._key = event.which;\n",
+ " }\n",
+ " if (name == 'key_release')\n",
+ " this._key = null;\n",
+ "\n",
+ " var value = '';\n",
+ " if (event.ctrlKey && event.which != 17)\n",
+ " value += \"ctrl+\";\n",
+ " if (event.altKey && event.which != 18)\n",
+ " value += \"alt+\";\n",
+ " if (event.shiftKey && event.which != 16)\n",
+ " value += \"shift+\";\n",
+ "\n",
+ " value += 'k';\n",
+ " value += event.which.toString();\n",
+ "\n",
+ " this._key_event_extra(event, name);\n",
+ "\n",
+ " this.send_message(name, {key: value,\n",
+ " guiEvent: simpleKeys(event)});\n",
+ " return false;\n",
+ "}\n",
+ "\n",
+ "mpl.figure.prototype.toolbar_button_onclick = function(name) {\n",
+ " if (name == 'download') {\n",
+ " this.handle_save(this, null);\n",
+ " } else {\n",
+ " this.send_message(\"toolbar_button\", {name: name});\n",
+ " }\n",
+ "};\n",
+ "\n",
+ "mpl.figure.prototype.toolbar_button_onmouseover = function(tooltip) {\n",
+ " this.message.textContent = tooltip;\n",
+ "};\n",
+ "mpl.toolbar_items = [[\"Home\", \"Reset original view\", \"fa fa-home icon-home\", \"home\"], [\"Back\", \"Back to previous view\", \"fa fa-arrow-left icon-arrow-left\", \"back\"], [\"Forward\", \"Forward to next view\", \"fa fa-arrow-right icon-arrow-right\", \"forward\"], [\"\", \"\", \"\", \"\"], [\"Pan\", \"Pan axes with left mouse, zoom with right\", \"fa fa-arrows icon-move\", \"pan\"], [\"Zoom\", \"Zoom to rectangle\", \"fa fa-square-o icon-check-empty\", \"zoom\"], [\"\", \"\", \"\", \"\"], [\"Download\", \"Download plot\", \"fa fa-floppy-o icon-save\", \"download\"]];\n",
+ "\n",
+ "mpl.extensions = [\"eps\", \"jpeg\", \"pdf\", \"png\", \"ps\", \"raw\", \"svg\", \"tif\"];\n",
+ "\n",
+ "mpl.default_extension = \"png\";var comm_websocket_adapter = function(comm) {\n",
+ " // Create a \"websocket\"-like object which calls the given IPython comm\n",
+ " // object with the appropriate methods. Currently this is a non binary\n",
+ " // socket, so there is still some room for performance tuning.\n",
+ " var ws = {};\n",
+ "\n",
+ " ws.close = function() {\n",
+ " comm.close()\n",
+ " };\n",
+ " ws.send = function(m) {\n",
+ " //console.log('sending', m);\n",
+ " comm.send(m);\n",
+ " };\n",
+ " // Register the callback with on_msg.\n",
+ " comm.on_msg(function(msg) {\n",
+ " //console.log('receiving', msg['content']['data'], msg);\n",
+ " // Pass the mpl event to the overridden (by mpl) onmessage function.\n",
+ " ws.onmessage(msg['content']['data'])\n",
+ " });\n",
+ " return ws;\n",
+ "}\n",
+ "\n",
+ "mpl.mpl_figure_comm = function(comm, msg) {\n",
+ " // This is the function which gets called when the mpl process\n",
+ " // starts-up an IPython Comm through the \"matplotlib\" channel.\n",
+ "\n",
+ " var id = msg.content.data.id;\n",
+ " // Get hold of the div created by the display call when the Comm\n",
+ " // socket was opened in Python.\n",
+ " var element = $(\"#\" + id);\n",
+ " var ws_proxy = comm_websocket_adapter(comm)\n",
+ "\n",
+ " function ondownload(figure, format) {\n",
+ " window.open(figure.imageObj.src);\n",
+ " }\n",
+ "\n",
+ " var fig = new mpl.figure(id, ws_proxy,\n",
+ " ondownload,\n",
+ " element.get(0));\n",
+ "\n",
+ " // Call onopen now - mpl needs it, as it is assuming we've passed it a real\n",
+ " // web socket which is closed, not our websocket->open comm proxy.\n",
+ " ws_proxy.onopen();\n",
+ "\n",
+ " fig.parent_element = element.get(0);\n",
+ " fig.cell_info = mpl.find_output_cell(\"\");\n",
+ " if (!fig.cell_info) {\n",
+ " console.error(\"Failed to find cell for figure\", id, fig);\n",
+ " return;\n",
+ " }\n",
+ "\n",
+ " var output_index = fig.cell_info[2]\n",
+ " var cell = fig.cell_info[0];\n",
+ "\n",
+ "};\n",
+ "\n",
+ "mpl.figure.prototype.handle_close = function(fig, msg) {\n",
+ " var width = fig.canvas.width/mpl.ratio\n",
+ " fig.root.unbind('remove')\n",
+ "\n",
+ " // Update the output cell to use the data from the current canvas.\n",
+ " fig.push_to_output();\n",
+ " var dataURL = fig.canvas.toDataURL();\n",
+ " // Re-enable the keyboard manager in IPython - without this line, in FF,\n",
+ " // the notebook keyboard shortcuts fail.\n",
+ " IPython.keyboard_manager.enable()\n",
+ " $(fig.parent_element).html('
');\n",
+ " fig.close_ws(fig, msg);\n",
+ "}\n",
+ "\n",
+ "mpl.figure.prototype.close_ws = function(fig, msg){\n",
+ " fig.send_message('closing', msg);\n",
+ " // fig.ws.close()\n",
+ "}\n",
+ "\n",
+ "mpl.figure.prototype.push_to_output = function(remove_interactive) {\n",
+ " // Turn the data on the canvas into data in the output cell.\n",
+ " var width = this.canvas.width/mpl.ratio\n",
+ " var dataURL = this.canvas.toDataURL();\n",
+ " this.cell_info[1]['text/html'] = '
';\n",
+ "}\n",
+ "\n",
+ "mpl.figure.prototype.updated_canvas_event = function() {\n",
+ " // Tell IPython that the notebook contents must change.\n",
+ " IPython.notebook.set_dirty(true);\n",
+ " this.send_message(\"ack\", {});\n",
+ " var fig = this;\n",
+ " // Wait a second, then push the new image to the DOM so\n",
+ " // that it is saved nicely (might be nice to debounce this).\n",
+ " setTimeout(function () { fig.push_to_output() }, 1000);\n",
+ "}\n",
+ "\n",
+ "mpl.figure.prototype._init_toolbar = function() {\n",
+ " var fig = this;\n",
+ "\n",
+ " var nav_element = $('');\n",
+ " nav_element.attr('style', 'width: 100%');\n",
+ " this.root.append(nav_element);\n",
+ "\n",
+ " // Define a callback function for later on.\n",
+ " function toolbar_event(event) {\n",
+ " return fig.toolbar_button_onclick(event['data']);\n",
+ " }\n",
+ " function toolbar_mouse_event(event) {\n",
+ " return fig.toolbar_button_onmouseover(event['data']);\n",
+ " }\n",
+ "\n",
+ " for(var toolbar_ind in mpl.toolbar_items){\n",
+ " var name = mpl.toolbar_items[toolbar_ind][0];\n",
+ " var tooltip = mpl.toolbar_items[toolbar_ind][1];\n",
+ " var image = mpl.toolbar_items[toolbar_ind][2];\n",
+ " var method_name = mpl.toolbar_items[toolbar_ind][3];\n",
+ "\n",
+ " if (!name) { continue; };\n",
+ "\n",
+ " var button = $('');\n",
+ " button.click(method_name, toolbar_event);\n",
+ " button.mouseover(tooltip, toolbar_mouse_event);\n",
+ " nav_element.append(button);\n",
+ " }\n",
+ "\n",
+ " // Add the status bar.\n",
+ " var status_bar = $('');\n",
+ " nav_element.append(status_bar);\n",
+ " this.message = status_bar[0];\n",
+ "\n",
+ " // Add the close button to the window.\n",
+ " var buttongrp = $('');\n",
+ " var button = $('');\n",
+ " button.click(function (evt) { fig.handle_close(fig, {}); } );\n",
+ " button.mouseover('Stop Interaction', toolbar_mouse_event);\n",
+ " buttongrp.append(button);\n",
+ " var titlebar = this.root.find($('.ui-dialog-titlebar'));\n",
+ " titlebar.prepend(buttongrp);\n",
+ "}\n",
+ "\n",
+ "mpl.figure.prototype._root_extra_style = function(el){\n",
+ " var fig = this\n",
+ " el.on(\"remove\", function(){\n",
+ "\tfig.close_ws(fig, {});\n",
+ " });\n",
+ "}\n",
+ "\n",
+ "mpl.figure.prototype._canvas_extra_style = function(el){\n",
+ " // this is important to make the div 'focusable\n",
+ " el.attr('tabindex', 0)\n",
+ " // reach out to IPython and tell the keyboard manager to turn it's self\n",
+ " // off when our div gets focus\n",
+ "\n",
+ " // location in version 3\n",
+ " if (IPython.notebook.keyboard_manager) {\n",
+ " IPython.notebook.keyboard_manager.register_events(el);\n",
+ " }\n",
+ " else {\n",
+ " // location in version 2\n",
+ " IPython.keyboard_manager.register_events(el);\n",
+ " }\n",
+ "\n",
+ "}\n",
+ "\n",
+ "mpl.figure.prototype._key_event_extra = function(event, name) {\n",
+ " var manager = IPython.notebook.keyboard_manager;\n",
+ " if (!manager)\n",
+ " manager = IPython.keyboard_manager;\n",
+ "\n",
+ " // Check for shift+enter\n",
+ " if (event.shiftKey && event.which == 13) {\n",
+ " this.canvas_div.blur();\n",
+ " event.shiftKey = false;\n",
+ " // Send a \"J\" for go to next cell\n",
+ " event.which = 74;\n",
+ " event.keyCode = 74;\n",
+ " manager.command_mode();\n",
+ " manager.handle_keydown(event);\n",
+ " }\n",
+ "}\n",
+ "\n",
+ "mpl.figure.prototype.handle_save = function(fig, msg) {\n",
+ " fig.ondownload(fig, null);\n",
+ "}\n",
+ "\n",
+ "\n",
+ "mpl.find_output_cell = function(html_output) {\n",
+ " // Return the cell and output element which can be found *uniquely* in the notebook.\n",
+ " // Note - this is a bit hacky, but it is done because the \"notebook_saving.Notebook\"\n",
+ " // IPython event is triggered only after the cells have been serialised, which for\n",
+ " // our purposes (turning an active figure into a static one), is too late.\n",
+ " var cells = IPython.notebook.get_cells();\n",
+ " var ncells = cells.length;\n",
+ " for (var i=0; i= 3 moved mimebundle to data attribute of output\n",
+ " data = data.data;\n",
+ " }\n",
+ " if (data['text/html'] == html_output) {\n",
+ " return [cell, data, j];\n",
+ " }\n",
+ " }\n",
+ " }\n",
+ " }\n",
+ "}\n",
+ "\n",
+ "// Register the function which deals with the matplotlib target/channel.\n",
+ "// The kernel may be null if the page has been refreshed.\n",
+ "if (IPython.notebook.kernel != null) {\n",
+ " IPython.notebook.kernel.comm_manager.register_target('matplotlib', mpl.mpl_figure_comm);\n",
+ "}\n"
+ ],
+ "text/plain": [
+ ""
+ ]
+ },
+ "metadata": {},
+ "output_type": "display_data"
+ },
+ {
+ "data": {
+ "text/html": [
+ "
"
+ ],
+ "text/plain": [
+ ""
+ ]
+ },
+ "metadata": {},
+ "output_type": "display_data"
+ },
+ {
+ "data": {
+ "text/plain": [
+ ""
+ ]
+ },
+ "execution_count": 7,
+ "metadata": {},
+ "output_type": "execute_result"
+ }
+ ],
+ "source": [
+ "im1 = imread('../data/target_01.jpg')\n",
+ "im2 = imread('../data/target_02.jpg')\n",
+ "plt.figure()\n",
+ "plt.imshow(im1)\n"
+ ]
+ },
+ {
+ "cell_type": "markdown",
+ "metadata": {},
+ "source": [
+ "### Capture mouse clicks on the frame\n",
+ "\n",
+ "Okay! Here is where things get really interesting. Matplotlib has the ability to create [event connections](https://matplotlib.org/devdocs/users/event_handling.html?highlight=mpl_connect), that is, connect the figure canvas to user-interface events on it, like mouse clicks. \n",
+ "\n",
+ "To use this ability, you write a function with the events you want to capture, and then connect this function to the Matplotlib \"event manager\" using [`mpl_connect()`](https://matplotlib.org/devdocs/api/backend_bases_api.html#matplotlib.backend_bases.FigureCanvasBase.mpl_connect). In this case, we connect the `'button_press_event'` to the function named `onclick()`, which captures the $(x,y)$ coordinates of the mouse click on the figure. Magic!"
+ ]
+ },
+ {
+ "cell_type": "code",
+ "execution_count": 14,
+ "metadata": {},
+ "outputs": [],
+ "source": [
+ "def onclick(event):\n",
+ " '''Capture the x,y coordinates of a mouse click on the image'''\n",
+ " ix, iy = event.xdata, event.ydata\n",
+ " coords.append([ix, iy]) "
+ ]
+ },
+ {
+ "cell_type": "code",
+ "execution_count": 15,
+ "metadata": {},
+ "outputs": [
+ {
+ "data": {
+ "application/javascript": [
+ "/* Put everything inside the global mpl namespace */\n",
+ "window.mpl = {};\n",
+ "\n",
+ "\n",
+ "mpl.get_websocket_type = function() {\n",
+ " if (typeof(WebSocket) !== 'undefined') {\n",
+ " return WebSocket;\n",
+ " } else if (typeof(MozWebSocket) !== 'undefined') {\n",
+ " return MozWebSocket;\n",
+ " } else {\n",
+ " alert('Your browser does not have WebSocket support. ' +\n",
+ " 'Please try Chrome, Safari or Firefox ≥ 6. ' +\n",
+ " 'Firefox 4 and 5 are also supported but you ' +\n",
+ " 'have to enable WebSockets in about:config.');\n",
+ " };\n",
+ "}\n",
+ "\n",
+ "mpl.figure = function(figure_id, websocket, ondownload, parent_element) {\n",
+ " this.id = figure_id;\n",
+ "\n",
+ " this.ws = websocket;\n",
+ "\n",
+ " this.supports_binary = (this.ws.binaryType != undefined);\n",
+ "\n",
+ " if (!this.supports_binary) {\n",
+ " var warnings = document.getElementById(\"mpl-warnings\");\n",
+ " if (warnings) {\n",
+ " warnings.style.display = 'block';\n",
+ " warnings.textContent = (\n",
+ " \"This browser does not support binary websocket messages. \" +\n",
+ " \"Performance may be slow.\");\n",
+ " }\n",
+ " }\n",
+ "\n",
+ " this.imageObj = new Image();\n",
+ "\n",
+ " this.context = undefined;\n",
+ " this.message = undefined;\n",
+ " this.canvas = undefined;\n",
+ " this.rubberband_canvas = undefined;\n",
+ " this.rubberband_context = undefined;\n",
+ " this.format_dropdown = undefined;\n",
+ "\n",
+ " this.image_mode = 'full';\n",
+ "\n",
+ " this.root = $('');\n",
+ " this._root_extra_style(this.root)\n",
+ " this.root.attr('style', 'display: inline-block');\n",
+ "\n",
+ " $(parent_element).append(this.root);\n",
+ "\n",
+ " this._init_header(this);\n",
+ " this._init_canvas(this);\n",
+ " this._init_toolbar(this);\n",
+ "\n",
+ " var fig = this;\n",
+ "\n",
+ " this.waiting = false;\n",
+ "\n",
+ " this.ws.onopen = function () {\n",
+ " fig.send_message(\"supports_binary\", {value: fig.supports_binary});\n",
+ " fig.send_message(\"send_image_mode\", {});\n",
+ " if (mpl.ratio != 1) {\n",
+ " fig.send_message(\"set_dpi_ratio\", {'dpi_ratio': mpl.ratio});\n",
+ " }\n",
+ " fig.send_message(\"refresh\", {});\n",
+ " }\n",
+ "\n",
+ " this.imageObj.onload = function() {\n",
+ " if (fig.image_mode == 'full') {\n",
+ " // Full images could contain transparency (where diff images\n",
+ " // almost always do), so we need to clear the canvas so that\n",
+ " // there is no ghosting.\n",
+ " fig.context.clearRect(0, 0, fig.canvas.width, fig.canvas.height);\n",
+ " }\n",
+ " fig.context.drawImage(fig.imageObj, 0, 0);\n",
+ " };\n",
+ "\n",
+ " this.imageObj.onunload = function() {\n",
+ " fig.ws.close();\n",
+ " }\n",
+ "\n",
+ " this.ws.onmessage = this._make_on_message_function(this);\n",
+ "\n",
+ " this.ondownload = ondownload;\n",
+ "}\n",
+ "\n",
+ "mpl.figure.prototype._init_header = function() {\n",
+ " var titlebar = $(\n",
+ " '');\n",
+ " var titletext = $(\n",
+ " '');\n",
+ " titlebar.append(titletext)\n",
+ " this.root.append(titlebar);\n",
+ " this.header = titletext[0];\n",
+ "}\n",
+ "\n",
+ "\n",
+ "\n",
+ "mpl.figure.prototype._canvas_extra_style = function(canvas_div) {\n",
+ "\n",
+ "}\n",
+ "\n",
+ "\n",
+ "mpl.figure.prototype._root_extra_style = function(canvas_div) {\n",
+ "\n",
+ "}\n",
+ "\n",
+ "mpl.figure.prototype._init_canvas = function() {\n",
+ " var fig = this;\n",
+ "\n",
+ " var canvas_div = $('');\n",
+ "\n",
+ " canvas_div.attr('style', 'position: relative; clear: both; outline: 0');\n",
+ "\n",
+ " function canvas_keyboard_event(event) {\n",
+ " return fig.key_event(event, event['data']);\n",
+ " }\n",
+ "\n",
+ " canvas_div.keydown('key_press', canvas_keyboard_event);\n",
+ " canvas_div.keyup('key_release', canvas_keyboard_event);\n",
+ " this.canvas_div = canvas_div\n",
+ " this._canvas_extra_style(canvas_div)\n",
+ " this.root.append(canvas_div);\n",
+ "\n",
+ " var canvas = $('');\n",
+ " canvas.addClass('mpl-canvas');\n",
+ " canvas.attr('style', \"left: 0; top: 0; z-index: 0; outline: 0\")\n",
+ "\n",
+ " this.canvas = canvas[0];\n",
+ " this.context = canvas[0].getContext(\"2d\");\n",
+ "\n",
+ " var backingStore = this.context.backingStorePixelRatio ||\n",
+ "\tthis.context.webkitBackingStorePixelRatio ||\n",
+ "\tthis.context.mozBackingStorePixelRatio ||\n",
+ "\tthis.context.msBackingStorePixelRatio ||\n",
+ "\tthis.context.oBackingStorePixelRatio ||\n",
+ "\tthis.context.backingStorePixelRatio || 1;\n",
+ "\n",
+ " mpl.ratio = (window.devicePixelRatio || 1) / backingStore;\n",
+ "\n",
+ " var rubberband = $('');\n",
+ " rubberband.attr('style', \"position: absolute; left: 0; top: 0; z-index: 1;\")\n",
+ "\n",
+ " var pass_mouse_events = true;\n",
+ "\n",
+ " canvas_div.resizable({\n",
+ " start: function(event, ui) {\n",
+ " pass_mouse_events = false;\n",
+ " },\n",
+ " resize: function(event, ui) {\n",
+ " fig.request_resize(ui.size.width, ui.size.height);\n",
+ " },\n",
+ " stop: function(event, ui) {\n",
+ " pass_mouse_events = true;\n",
+ " fig.request_resize(ui.size.width, ui.size.height);\n",
+ " },\n",
+ " });\n",
+ "\n",
+ " function mouse_event_fn(event) {\n",
+ " if (pass_mouse_events)\n",
+ " return fig.mouse_event(event, event['data']);\n",
+ " }\n",
+ "\n",
+ " rubberband.mousedown('button_press', mouse_event_fn);\n",
+ " rubberband.mouseup('button_release', mouse_event_fn);\n",
+ " // Throttle sequential mouse events to 1 every 20ms.\n",
+ " rubberband.mousemove('motion_notify', mouse_event_fn);\n",
+ "\n",
+ " rubberband.mouseenter('figure_enter', mouse_event_fn);\n",
+ " rubberband.mouseleave('figure_leave', mouse_event_fn);\n",
+ "\n",
+ " canvas_div.on(\"wheel\", function (event) {\n",
+ " event = event.originalEvent;\n",
+ " event['data'] = 'scroll'\n",
+ " if (event.deltaY < 0) {\n",
+ " event.step = 1;\n",
+ " } else {\n",
+ " event.step = -1;\n",
+ " }\n",
+ " mouse_event_fn(event);\n",
+ " });\n",
+ "\n",
+ " canvas_div.append(canvas);\n",
+ " canvas_div.append(rubberband);\n",
+ "\n",
+ " this.rubberband = rubberband;\n",
+ " this.rubberband_canvas = rubberband[0];\n",
+ " this.rubberband_context = rubberband[0].getContext(\"2d\");\n",
+ " this.rubberband_context.strokeStyle = \"#000000\";\n",
+ "\n",
+ " this._resize_canvas = function(width, height) {\n",
+ " // Keep the size of the canvas, canvas container, and rubber band\n",
+ " // canvas in synch.\n",
+ " canvas_div.css('width', width)\n",
+ " canvas_div.css('height', height)\n",
+ "\n",
+ " canvas.attr('width', width * mpl.ratio);\n",
+ " canvas.attr('height', height * mpl.ratio);\n",
+ " canvas.attr('style', 'width: ' + width + 'px; height: ' + height + 'px;');\n",
+ "\n",
+ " rubberband.attr('width', width);\n",
+ " rubberband.attr('height', height);\n",
+ " }\n",
+ "\n",
+ " // Set the figure to an initial 600x600px, this will subsequently be updated\n",
+ " // upon first draw.\n",
+ " this._resize_canvas(600, 600);\n",
+ "\n",
+ " // Disable right mouse context menu.\n",
+ " $(this.rubberband_canvas).bind(\"contextmenu\",function(e){\n",
+ " return false;\n",
+ " });\n",
+ "\n",
+ " function set_focus () {\n",
+ " canvas.focus();\n",
+ " canvas_div.focus();\n",
+ " }\n",
+ "\n",
+ " window.setTimeout(set_focus, 100);\n",
+ "}\n",
+ "\n",
+ "mpl.figure.prototype._init_toolbar = function() {\n",
+ " var fig = this;\n",
+ "\n",
+ " var nav_element = $('');\n",
+ " nav_element.attr('style', 'width: 100%');\n",
+ " this.root.append(nav_element);\n",
+ "\n",
+ " // Define a callback function for later on.\n",
+ " function toolbar_event(event) {\n",
+ " return fig.toolbar_button_onclick(event['data']);\n",
+ " }\n",
+ " function toolbar_mouse_event(event) {\n",
+ " return fig.toolbar_button_onmouseover(event['data']);\n",
+ " }\n",
+ "\n",
+ " for(var toolbar_ind in mpl.toolbar_items) {\n",
+ " var name = mpl.toolbar_items[toolbar_ind][0];\n",
+ " var tooltip = mpl.toolbar_items[toolbar_ind][1];\n",
+ " var image = mpl.toolbar_items[toolbar_ind][2];\n",
+ " var method_name = mpl.toolbar_items[toolbar_ind][3];\n",
+ "\n",
+ " if (!name) {\n",
+ " // put a spacer in here.\n",
+ " continue;\n",
+ " }\n",
+ " var button = $('');\n",
+ " button.addClass('ui-button ui-widget ui-state-default ui-corner-all ' +\n",
+ " 'ui-button-icon-only');\n",
+ " button.attr('role', 'button');\n",
+ " button.attr('aria-disabled', 'false');\n",
+ " button.click(method_name, toolbar_event);\n",
+ " button.mouseover(tooltip, toolbar_mouse_event);\n",
+ "\n",
+ " var icon_img = $('');\n",
+ " icon_img.addClass('ui-button-icon-primary ui-icon');\n",
+ " icon_img.addClass(image);\n",
+ " icon_img.addClass('ui-corner-all');\n",
+ "\n",
+ " var tooltip_span = $('');\n",
+ " tooltip_span.addClass('ui-button-text');\n",
+ " tooltip_span.html(tooltip);\n",
+ "\n",
+ " button.append(icon_img);\n",
+ " button.append(tooltip_span);\n",
+ "\n",
+ " nav_element.append(button);\n",
+ " }\n",
+ "\n",
+ " var fmt_picker_span = $('');\n",
+ "\n",
+ " var fmt_picker = $('');\n",
+ " fmt_picker.addClass('mpl-toolbar-option ui-widget ui-widget-content');\n",
+ " fmt_picker_span.append(fmt_picker);\n",
+ " nav_element.append(fmt_picker_span);\n",
+ " this.format_dropdown = fmt_picker[0];\n",
+ "\n",
+ " for (var ind in mpl.extensions) {\n",
+ " var fmt = mpl.extensions[ind];\n",
+ " var option = $(\n",
+ " '', {selected: fmt === mpl.default_extension}).html(fmt);\n",
+ " fmt_picker.append(option);\n",
+ " }\n",
+ "\n",
+ " // Add hover states to the ui-buttons\n",
+ " $( \".ui-button\" ).hover(\n",
+ " function() { $(this).addClass(\"ui-state-hover\");},\n",
+ " function() { $(this).removeClass(\"ui-state-hover\");}\n",
+ " );\n",
+ "\n",
+ " var status_bar = $('');\n",
+ " nav_element.append(status_bar);\n",
+ " this.message = status_bar[0];\n",
+ "}\n",
+ "\n",
+ "mpl.figure.prototype.request_resize = function(x_pixels, y_pixels) {\n",
+ " // Request matplotlib to resize the figure. Matplotlib will then trigger a resize in the client,\n",
+ " // which will in turn request a refresh of the image.\n",
+ " this.send_message('resize', {'width': x_pixels, 'height': y_pixels});\n",
+ "}\n",
+ "\n",
+ "mpl.figure.prototype.send_message = function(type, properties) {\n",
+ " properties['type'] = type;\n",
+ " properties['figure_id'] = this.id;\n",
+ " this.ws.send(JSON.stringify(properties));\n",
+ "}\n",
+ "\n",
+ "mpl.figure.prototype.send_draw_message = function() {\n",
+ " if (!this.waiting) {\n",
+ " this.waiting = true;\n",
+ " this.ws.send(JSON.stringify({type: \"draw\", figure_id: this.id}));\n",
+ " }\n",
+ "}\n",
+ "\n",
+ "\n",
+ "mpl.figure.prototype.handle_save = function(fig, msg) {\n",
+ " var format_dropdown = fig.format_dropdown;\n",
+ " var format = format_dropdown.options[format_dropdown.selectedIndex].value;\n",
+ " fig.ondownload(fig, format);\n",
+ "}\n",
+ "\n",
+ "\n",
+ "mpl.figure.prototype.handle_resize = function(fig, msg) {\n",
+ " var size = msg['size'];\n",
+ " if (size[0] != fig.canvas.width || size[1] != fig.canvas.height) {\n",
+ " fig._resize_canvas(size[0], size[1]);\n",
+ " fig.send_message(\"refresh\", {});\n",
+ " };\n",
+ "}\n",
+ "\n",
+ "mpl.figure.prototype.handle_rubberband = function(fig, msg) {\n",
+ " var x0 = msg['x0'] / mpl.ratio;\n",
+ " var y0 = (fig.canvas.height - msg['y0']) / mpl.ratio;\n",
+ " var x1 = msg['x1'] / mpl.ratio;\n",
+ " var y1 = (fig.canvas.height - msg['y1']) / mpl.ratio;\n",
+ " x0 = Math.floor(x0) + 0.5;\n",
+ " y0 = Math.floor(y0) + 0.5;\n",
+ " x1 = Math.floor(x1) + 0.5;\n",
+ " y1 = Math.floor(y1) + 0.5;\n",
+ " var min_x = Math.min(x0, x1);\n",
+ " var min_y = Math.min(y0, y1);\n",
+ " var width = Math.abs(x1 - x0);\n",
+ " var height = Math.abs(y1 - y0);\n",
+ "\n",
+ " fig.rubberband_context.clearRect(\n",
+ " 0, 0, fig.canvas.width / mpl.ratio, fig.canvas.height / mpl.ratio);\n",
+ "\n",
+ " fig.rubberband_context.strokeRect(min_x, min_y, width, height);\n",
+ "}\n",
+ "\n",
+ "mpl.figure.prototype.handle_figure_label = function(fig, msg) {\n",
+ " // Updates the figure title.\n",
+ " fig.header.textContent = msg['label'];\n",
+ "}\n",
+ "\n",
+ "mpl.figure.prototype.handle_cursor = function(fig, msg) {\n",
+ " var cursor = msg['cursor'];\n",
+ " switch(cursor)\n",
+ " {\n",
+ " case 0:\n",
+ " cursor = 'pointer';\n",
+ " break;\n",
+ " case 1:\n",
+ " cursor = 'default';\n",
+ " break;\n",
+ " case 2:\n",
+ " cursor = 'crosshair';\n",
+ " break;\n",
+ " case 3:\n",
+ " cursor = 'move';\n",
+ " break;\n",
+ " }\n",
+ " fig.rubberband_canvas.style.cursor = cursor;\n",
+ "}\n",
+ "\n",
+ "mpl.figure.prototype.handle_message = function(fig, msg) {\n",
+ " fig.message.textContent = msg['message'];\n",
+ "}\n",
+ "\n",
+ "mpl.figure.prototype.handle_draw = function(fig, msg) {\n",
+ " // Request the server to send over a new figure.\n",
+ " fig.send_draw_message();\n",
+ "}\n",
+ "\n",
+ "mpl.figure.prototype.handle_image_mode = function(fig, msg) {\n",
+ " fig.image_mode = msg['mode'];\n",
+ "}\n",
+ "\n",
+ "mpl.figure.prototype.updated_canvas_event = function() {\n",
+ " // Called whenever the canvas gets updated.\n",
+ " this.send_message(\"ack\", {});\n",
+ "}\n",
+ "\n",
+ "// A function to construct a web socket function for onmessage handling.\n",
+ "// Called in the figure constructor.\n",
+ "mpl.figure.prototype._make_on_message_function = function(fig) {\n",
+ " return function socket_on_message(evt) {\n",
+ " if (evt.data instanceof Blob) {\n",
+ " /* FIXME: We get \"Resource interpreted as Image but\n",
+ " * transferred with MIME type text/plain:\" errors on\n",
+ " * Chrome. But how to set the MIME type? It doesn't seem\n",
+ " * to be part of the websocket stream */\n",
+ " evt.data.type = \"image/png\";\n",
+ "\n",
+ " /* Free the memory for the previous frames */\n",
+ " if (fig.imageObj.src) {\n",
+ " (window.URL || window.webkitURL).revokeObjectURL(\n",
+ " fig.imageObj.src);\n",
+ " }\n",
+ "\n",
+ " fig.imageObj.src = (window.URL || window.webkitURL).createObjectURL(\n",
+ " evt.data);\n",
+ " fig.updated_canvas_event();\n",
+ " fig.waiting = false;\n",
+ " return;\n",
+ " }\n",
+ " else if (typeof evt.data === 'string' && evt.data.slice(0, 21) == \"data:image/png;base64\") {\n",
+ " fig.imageObj.src = evt.data;\n",
+ " fig.updated_canvas_event();\n",
+ " fig.waiting = false;\n",
+ " return;\n",
+ " }\n",
+ "\n",
+ " var msg = JSON.parse(evt.data);\n",
+ " var msg_type = msg['type'];\n",
+ "\n",
+ " // Call the \"handle_{type}\" callback, which takes\n",
+ " // the figure and JSON message as its only arguments.\n",
+ " try {\n",
+ " var callback = fig[\"handle_\" + msg_type];\n",
+ " } catch (e) {\n",
+ " console.log(\"No handler for the '\" + msg_type + \"' message type: \", msg);\n",
+ " return;\n",
+ " }\n",
+ "\n",
+ " if (callback) {\n",
+ " try {\n",
+ " // console.log(\"Handling '\" + msg_type + \"' message: \", msg);\n",
+ " callback(fig, msg);\n",
+ " } catch (e) {\n",
+ " console.log(\"Exception inside the 'handler_\" + msg_type + \"' callback:\", e, e.stack, msg);\n",
+ " }\n",
+ " }\n",
+ " };\n",
+ "}\n",
+ "\n",
+ "// from http://stackoverflow.com/questions/1114465/getting-mouse-location-in-canvas\n",
+ "mpl.findpos = function(e) {\n",
+ " //this section is from http://www.quirksmode.org/js/events_properties.html\n",
+ " var targ;\n",
+ " if (!e)\n",
+ " e = window.event;\n",
+ " if (e.target)\n",
+ " targ = e.target;\n",
+ " else if (e.srcElement)\n",
+ " targ = e.srcElement;\n",
+ " if (targ.nodeType == 3) // defeat Safari bug\n",
+ " targ = targ.parentNode;\n",
+ "\n",
+ " // jQuery normalizes the pageX and pageY\n",
+ " // pageX,Y are the mouse positions relative to the document\n",
+ " // offset() returns the position of the element relative to the document\n",
+ " var x = e.pageX - $(targ).offset().left;\n",
+ " var y = e.pageY - $(targ).offset().top;\n",
+ "\n",
+ " return {\"x\": x, \"y\": y};\n",
+ "};\n",
+ "\n",
+ "/*\n",
+ " * return a copy of an object with only non-object keys\n",
+ " * we need this to avoid circular references\n",
+ " * http://stackoverflow.com/a/24161582/3208463\n",
+ " */\n",
+ "function simpleKeys (original) {\n",
+ " return Object.keys(original).reduce(function (obj, key) {\n",
+ " if (typeof original[key] !== 'object')\n",
+ " obj[key] = original[key]\n",
+ " return obj;\n",
+ " }, {});\n",
+ "}\n",
+ "\n",
+ "mpl.figure.prototype.mouse_event = function(event, name) {\n",
+ " var canvas_pos = mpl.findpos(event)\n",
+ "\n",
+ " if (name === 'button_press')\n",
+ " {\n",
+ " this.canvas.focus();\n",
+ " this.canvas_div.focus();\n",
+ " }\n",
+ "\n",
+ " var x = canvas_pos.x * mpl.ratio;\n",
+ " var y = canvas_pos.y * mpl.ratio;\n",
+ "\n",
+ " this.send_message(name, {x: x, y: y, button: event.button,\n",
+ " step: event.step,\n",
+ " guiEvent: simpleKeys(event)});\n",
+ "\n",
+ " /* This prevents the web browser from automatically changing to\n",
+ " * the text insertion cursor when the button is pressed. We want\n",
+ " * to control all of the cursor setting manually through the\n",
+ " * 'cursor' event from matplotlib */\n",
+ " event.preventDefault();\n",
+ " return false;\n",
+ "}\n",
+ "\n",
+ "mpl.figure.prototype._key_event_extra = function(event, name) {\n",
+ " // Handle any extra behaviour associated with a key event\n",
+ "}\n",
+ "\n",
+ "mpl.figure.prototype.key_event = function(event, name) {\n",
+ "\n",
+ " // Prevent repeat events\n",
+ " if (name == 'key_press')\n",
+ " {\n",
+ " if (event.which === this._key)\n",
+ " return;\n",
+ " else\n",
+ " this._key = event.which;\n",
+ " }\n",
+ " if (name == 'key_release')\n",
+ " this._key = null;\n",
+ "\n",
+ " var value = '';\n",
+ " if (event.ctrlKey && event.which != 17)\n",
+ " value += \"ctrl+\";\n",
+ " if (event.altKey && event.which != 18)\n",
+ " value += \"alt+\";\n",
+ " if (event.shiftKey && event.which != 16)\n",
+ " value += \"shift+\";\n",
+ "\n",
+ " value += 'k';\n",
+ " value += event.which.toString();\n",
+ "\n",
+ " this._key_event_extra(event, name);\n",
+ "\n",
+ " this.send_message(name, {key: value,\n",
+ " guiEvent: simpleKeys(event)});\n",
+ " return false;\n",
+ "}\n",
+ "\n",
+ "mpl.figure.prototype.toolbar_button_onclick = function(name) {\n",
+ " if (name == 'download') {\n",
+ " this.handle_save(this, null);\n",
+ " } else {\n",
+ " this.send_message(\"toolbar_button\", {name: name});\n",
+ " }\n",
+ "};\n",
+ "\n",
+ "mpl.figure.prototype.toolbar_button_onmouseover = function(tooltip) {\n",
+ " this.message.textContent = tooltip;\n",
+ "};\n",
+ "mpl.toolbar_items = [[\"Home\", \"Reset original view\", \"fa fa-home icon-home\", \"home\"], [\"Back\", \"Back to previous view\", \"fa fa-arrow-left icon-arrow-left\", \"back\"], [\"Forward\", \"Forward to next view\", \"fa fa-arrow-right icon-arrow-right\", \"forward\"], [\"\", \"\", \"\", \"\"], [\"Pan\", \"Pan axes with left mouse, zoom with right\", \"fa fa-arrows icon-move\", \"pan\"], [\"Zoom\", \"Zoom to rectangle\", \"fa fa-square-o icon-check-empty\", \"zoom\"], [\"\", \"\", \"\", \"\"], [\"Download\", \"Download plot\", \"fa fa-floppy-o icon-save\", \"download\"]];\n",
+ "\n",
+ "mpl.extensions = [\"eps\", \"jpeg\", \"pdf\", \"png\", \"ps\", \"raw\", \"svg\", \"tif\"];\n",
+ "\n",
+ "mpl.default_extension = \"png\";var comm_websocket_adapter = function(comm) {\n",
+ " // Create a \"websocket\"-like object which calls the given IPython comm\n",
+ " // object with the appropriate methods. Currently this is a non binary\n",
+ " // socket, so there is still some room for performance tuning.\n",
+ " var ws = {};\n",
+ "\n",
+ " ws.close = function() {\n",
+ " comm.close()\n",
+ " };\n",
+ " ws.send = function(m) {\n",
+ " //console.log('sending', m);\n",
+ " comm.send(m);\n",
+ " };\n",
+ " // Register the callback with on_msg.\n",
+ " comm.on_msg(function(msg) {\n",
+ " //console.log('receiving', msg['content']['data'], msg);\n",
+ " // Pass the mpl event to the overridden (by mpl) onmessage function.\n",
+ " ws.onmessage(msg['content']['data'])\n",
+ " });\n",
+ " return ws;\n",
+ "}\n",
+ "\n",
+ "mpl.mpl_figure_comm = function(comm, msg) {\n",
+ " // This is the function which gets called when the mpl process\n",
+ " // starts-up an IPython Comm through the \"matplotlib\" channel.\n",
+ "\n",
+ " var id = msg.content.data.id;\n",
+ " // Get hold of the div created by the display call when the Comm\n",
+ " // socket was opened in Python.\n",
+ " var element = $(\"#\" + id);\n",
+ " var ws_proxy = comm_websocket_adapter(comm)\n",
+ "\n",
+ " function ondownload(figure, format) {\n",
+ " window.open(figure.imageObj.src);\n",
+ " }\n",
+ "\n",
+ " var fig = new mpl.figure(id, ws_proxy,\n",
+ " ondownload,\n",
+ " element.get(0));\n",
+ "\n",
+ " // Call onopen now - mpl needs it, as it is assuming we've passed it a real\n",
+ " // web socket which is closed, not our websocket->open comm proxy.\n",
+ " ws_proxy.onopen();\n",
+ "\n",
+ " fig.parent_element = element.get(0);\n",
+ " fig.cell_info = mpl.find_output_cell(\"\");\n",
+ " if (!fig.cell_info) {\n",
+ " console.error(\"Failed to find cell for figure\", id, fig);\n",
+ " return;\n",
+ " }\n",
+ "\n",
+ " var output_index = fig.cell_info[2]\n",
+ " var cell = fig.cell_info[0];\n",
+ "\n",
+ "};\n",
+ "\n",
+ "mpl.figure.prototype.handle_close = function(fig, msg) {\n",
+ " var width = fig.canvas.width/mpl.ratio\n",
+ " fig.root.unbind('remove')\n",
+ "\n",
+ " // Update the output cell to use the data from the current canvas.\n",
+ " fig.push_to_output();\n",
+ " var dataURL = fig.canvas.toDataURL();\n",
+ " // Re-enable the keyboard manager in IPython - without this line, in FF,\n",
+ " // the notebook keyboard shortcuts fail.\n",
+ " IPython.keyboard_manager.enable()\n",
+ " $(fig.parent_element).html('
');\n",
+ " fig.close_ws(fig, msg);\n",
+ "}\n",
+ "\n",
+ "mpl.figure.prototype.close_ws = function(fig, msg){\n",
+ " fig.send_message('closing', msg);\n",
+ " // fig.ws.close()\n",
+ "}\n",
+ "\n",
+ "mpl.figure.prototype.push_to_output = function(remove_interactive) {\n",
+ " // Turn the data on the canvas into data in the output cell.\n",
+ " var width = this.canvas.width/mpl.ratio\n",
+ " var dataURL = this.canvas.toDataURL();\n",
+ " this.cell_info[1]['text/html'] = '
';\n",
+ "}\n",
+ "\n",
+ "mpl.figure.prototype.updated_canvas_event = function() {\n",
+ " // Tell IPython that the notebook contents must change.\n",
+ " IPython.notebook.set_dirty(true);\n",
+ " this.send_message(\"ack\", {});\n",
+ " var fig = this;\n",
+ " // Wait a second, then push the new image to the DOM so\n",
+ " // that it is saved nicely (might be nice to debounce this).\n",
+ " setTimeout(function () { fig.push_to_output() }, 1000);\n",
+ "}\n",
+ "\n",
+ "mpl.figure.prototype._init_toolbar = function() {\n",
+ " var fig = this;\n",
+ "\n",
+ " var nav_element = $('');\n",
+ " nav_element.attr('style', 'width: 100%');\n",
+ " this.root.append(nav_element);\n",
+ "\n",
+ " // Define a callback function for later on.\n",
+ " function toolbar_event(event) {\n",
+ " return fig.toolbar_button_onclick(event['data']);\n",
+ " }\n",
+ " function toolbar_mouse_event(event) {\n",
+ " return fig.toolbar_button_onmouseover(event['data']);\n",
+ " }\n",
+ "\n",
+ " for(var toolbar_ind in mpl.toolbar_items){\n",
+ " var name = mpl.toolbar_items[toolbar_ind][0];\n",
+ " var tooltip = mpl.toolbar_items[toolbar_ind][1];\n",
+ " var image = mpl.toolbar_items[toolbar_ind][2];\n",
+ " var method_name = mpl.toolbar_items[toolbar_ind][3];\n",
+ "\n",
+ " if (!name) { continue; };\n",
+ "\n",
+ " var button = $('');\n",
+ " button.click(method_name, toolbar_event);\n",
+ " button.mouseover(tooltip, toolbar_mouse_event);\n",
+ " nav_element.append(button);\n",
+ " }\n",
+ "\n",
+ " // Add the status bar.\n",
+ " var status_bar = $('');\n",
+ " nav_element.append(status_bar);\n",
+ " this.message = status_bar[0];\n",
+ "\n",
+ " // Add the close button to the window.\n",
+ " var buttongrp = $('');\n",
+ " var button = $('');\n",
+ " button.click(function (evt) { fig.handle_close(fig, {}); } );\n",
+ " button.mouseover('Stop Interaction', toolbar_mouse_event);\n",
+ " buttongrp.append(button);\n",
+ " var titlebar = this.root.find($('.ui-dialog-titlebar'));\n",
+ " titlebar.prepend(buttongrp);\n",
+ "}\n",
+ "\n",
+ "mpl.figure.prototype._root_extra_style = function(el){\n",
+ " var fig = this\n",
+ " el.on(\"remove\", function(){\n",
+ "\tfig.close_ws(fig, {});\n",
+ " });\n",
+ "}\n",
+ "\n",
+ "mpl.figure.prototype._canvas_extra_style = function(el){\n",
+ " // this is important to make the div 'focusable\n",
+ " el.attr('tabindex', 0)\n",
+ " // reach out to IPython and tell the keyboard manager to turn it's self\n",
+ " // off when our div gets focus\n",
+ "\n",
+ " // location in version 3\n",
+ " if (IPython.notebook.keyboard_manager) {\n",
+ " IPython.notebook.keyboard_manager.register_events(el);\n",
+ " }\n",
+ " else {\n",
+ " // location in version 2\n",
+ " IPython.keyboard_manager.register_events(el);\n",
+ " }\n",
+ "\n",
+ "}\n",
+ "\n",
+ "mpl.figure.prototype._key_event_extra = function(event, name) {\n",
+ " var manager = IPython.notebook.keyboard_manager;\n",
+ " if (!manager)\n",
+ " manager = IPython.keyboard_manager;\n",
+ "\n",
+ " // Check for shift+enter\n",
+ " if (event.shiftKey && event.which == 13) {\n",
+ " this.canvas_div.blur();\n",
+ " event.shiftKey = false;\n",
+ " // Send a \"J\" for go to next cell\n",
+ " event.which = 74;\n",
+ " event.keyCode = 74;\n",
+ " manager.command_mode();\n",
+ " manager.handle_keydown(event);\n",
+ " }\n",
+ "}\n",
+ "\n",
+ "mpl.figure.prototype.handle_save = function(fig, msg) {\n",
+ " fig.ondownload(fig, null);\n",
+ "}\n",
+ "\n",
+ "\n",
+ "mpl.find_output_cell = function(html_output) {\n",
+ " // Return the cell and output element which can be found *uniquely* in the notebook.\n",
+ " // Note - this is a bit hacky, but it is done because the \"notebook_saving.Notebook\"\n",
+ " // IPython event is triggered only after the cells have been serialised, which for\n",
+ " // our purposes (turning an active figure into a static one), is too late.\n",
+ " var cells = IPython.notebook.get_cells();\n",
+ " var ncells = cells.length;\n",
+ " for (var i=0; i= 3 moved mimebundle to data attribute of output\n",
+ " data = data.data;\n",
+ " }\n",
+ " if (data['text/html'] == html_output) {\n",
+ " return [cell, data, j];\n",
+ " }\n",
+ " }\n",
+ " }\n",
+ " }\n",
+ "}\n",
+ "\n",
+ "// Register the function which deals with the matplotlib target/channel.\n",
+ "// The kernel may be null if the page has been refreshed.\n",
+ "if (IPython.notebook.kernel != null) {\n",
+ " IPython.notebook.kernel.comm_manager.register_target('matplotlib', mpl.mpl_figure_comm);\n",
+ "}\n"
+ ],
+ "text/plain": [
+ ""
+ ]
+ },
+ "metadata": {},
+ "output_type": "display_data"
+ },
+ {
+ "data": {
+ "text/html": [
+ "
"
+ ],
+ "text/plain": [
+ ""
+ ]
+ },
+ "metadata": {},
+ "output_type": "display_data"
+ }
+ ],
+ "source": [
+ "fig = plt.figure(3)\n",
+ "plt.imshow(im1, interpolation='nearest')\n",
+ "\n",
+ "coords = []\n",
+ "connectId = fig.canvas.mpl_connect('button_press_event', onclick)"
+ ]
+ },
+ {
+ "cell_type": "markdown",
+ "metadata": {},
+ "source": [
+ "Notice that in the previous code cell, we created an empty list named `coords`, and inside the `onclick()` function, we are appending to it the $(x,y)$ coordinates of each mouse click on the figure. After executing the cell above, you have a connection to the figure, via the user interface: try clicking with your mouse on the endpoints of the white lines of the metered panel (click on the edge of the panel to get approximately equal $x$ coordinates), then print the contents of the `coords` list below."
+ ]
+ },
+ {
+ "cell_type": "code",
+ "execution_count": 16,
+ "metadata": {},
+ "outputs": [
+ {
+ "data": {
+ "text/plain": [
+ "[]"
+ ]
+ },
+ "execution_count": 16,
+ "metadata": {},
+ "output_type": "execute_result"
+ }
+ ],
+ "source": [
+ "coords"
+ ]
+ },
+ {
+ "cell_type": "markdown",
+ "metadata": {},
+ "source": [
+ "The $x$ coordinates are pretty close, but there is some variation due to our shaky hand (or bad eyesight), and perhaps because the metered panel is not perfectly vertical. We can cast the `coords` list to a NumPy array, then grab all the first elements of the coordinate pairs, then get the standard deviation as an indication of our error in the mouse-click captures."
+ ]
+ },
+ {
+ "cell_type": "markdown",
+ "metadata": {},
+ "source": [
+ "## Save your work to a file\n",
+ "\n",
+ "In the next two code cells, we assign the clicks that were in the list `coords` to a numpy array `hits1`. Then, we save this array to the file `hits_im1.npy`. This is a numpy-readable file that we can load back into our workspace later as `np.load('../data/hits_im1.npy')`."
+ ]
+ },
+ {
+ "cell_type": "code",
+ "execution_count": 17,
+ "metadata": {},
+ "outputs": [],
+ "source": [
+ "hits1 = np.array(coords)"
+ ]
+ },
{
"cell_type": "code",
- "execution_count": 2,
+ "execution_count": 18,
"metadata": {},
"outputs": [],
"source": [
- "import matplotlib.pyplot as plt\n",
- "from matplotlib.image import imread\n",
- "import numpy as np\n",
- "%matplotlib notebook"
+ "np.save('../data/hits_im1.npy',hits1)"
]
},
{
"cell_type": "code",
- "execution_count": 3,
+ "execution_count": 47,
"metadata": {},
"outputs": [
{
@@ -800,7 +2529,7 @@
{
"data": {
"text/html": [
- "
"
+ "
"
],
"text/plain": [
""
@@ -812,21 +2541,44 @@
{
"data": {
"text/plain": [
- "[]"
+ "[]"
]
},
- "execution_count": 3,
+ "execution_count": 47,
"metadata": {},
"output_type": "execute_result"
}
],
"source": [
- "plt.plot(np.random.rand(10))"
+ "plt.figure()\n",
+ "plt.imshow(im1)\n",
+ "\n",
+ "plt.plot(hits1[:,0],hits1[:,1],'x')\n",
+ "#plt.plot(rings[:,0],rings[:,1],'s')"
+ ]
+ },
+ {
+ "cell_type": "markdown",
+ "metadata": {},
+ "source": [
+ "# Rinse and repeat\n",
+ "\n",
+ "Now we will repeat the process for `hits2` collecting the hit locations in target_02.jpg."
+ ]
+ },
+ {
+ "cell_type": "markdown",
+ "metadata": {},
+ "source": [
+ "### Capture mouse clicks on the frame\n",
+ "\n",
+ "Once again we create [event connections](https://matplotlib.org/devdocs/users/event_handling.html?highlight=mpl_connect), that is, connect the figure canvas to user-interface events on it, like mouse clicks. \n",
+ "We connect the `'button_press_event'` to the function named `onclick()`, which captures the $(x,y)$ coordinates of the mouse click on the figure. Magic!"
]
},
{
"cell_type": "code",
- "execution_count": 6,
+ "execution_count": 20,
"metadata": {},
"outputs": [
{
@@ -1612,7 +3364,7 @@
{
"data": {
"text/html": [
- "
"
+ "
"
],
"text/plain": [
""
@@ -1620,50 +3372,53 @@
},
"metadata": {},
"output_type": "display_data"
- },
- {
- "data": {
- "text/plain": [
- ""
- ]
- },
- "execution_count": 6,
- "metadata": {},
- "output_type": "execute_result"
}
],
"source": [
- "im = imread('../data/archery.jpg')\n",
- "plt.figure()\n",
- "plt.imshow(im)\n"
+ "fig = plt.figure()\n",
+ "plt.imshow(im2, interpolation='nearest')\n",
+ "\n",
+ "coords = []\n",
+ "connectId = fig.canvas.mpl_connect('button_press_event', onclick)"
]
},
{
"cell_type": "markdown",
"metadata": {},
"source": [
- "### Capture mouse clicks on the frame\n",
- "\n",
- "Okay! Here is where things get really interesting. Matplotlib has the ability to create [event connections](https://matplotlib.org/devdocs/users/event_handling.html?highlight=mpl_connect), that is, connect the figure canvas to user-interface events on it, like mouse clicks. \n",
+ "The $x$ coordinates are pretty close, but there is some variation due to our shaky hand (or bad eyesight), and perhaps because the metered panel is not perfectly vertical. We can cast the `coords` list to a NumPy array, then grab all the first elements of the coordinate pairs, then get the standard deviation as an indication of our error in the mouse-click captures."
+ ]
+ },
+ {
+ "cell_type": "code",
+ "execution_count": 21,
+ "metadata": {},
+ "outputs": [],
+ "source": [
+ "hits2 = np.array(coords)"
+ ]
+ },
+ {
+ "cell_type": "markdown",
+ "metadata": {},
+ "source": [
+ "## Save your work to a file\n",
"\n",
- "To use this ability, you write a function with the events you want to capture, and then connect this function to the Matplotlib \"event manager\" using [`mpl_connect()`](https://matplotlib.org/devdocs/api/backend_bases_api.html#matplotlib.backend_bases.FigureCanvasBase.mpl_connect). In this case, we connect the `'button_press_event'` to the function named `onclick()`, which captures the $(x,y)$ coordinates of the mouse click on the figure. Magic!"
+ "In the next two code cells, we assign the clicks that were in the list `coords` to a numpy array `hits2`. Then, we save this array to the file `hits_im2.npy`. This is a numpy-readable file that we can load back into our workspace later as `np.load('../data/hits_im2.npy')`."
]
},
{
"cell_type": "code",
- "execution_count": 10,
+ "execution_count": 44,
"metadata": {},
"outputs": [],
"source": [
- "def onclick(event):\n",
- " '''Capture the x,y coordinates of a mouse click on the image'''\n",
- " ix, iy = event.xdata, event.ydata\n",
- " coords.append([ix, iy]) "
+ "np.save('../data/hits_im2.npy',hits2)"
]
},
{
"cell_type": "code",
- "execution_count": 63,
+ "execution_count": 45,
"metadata": {},
"outputs": [
{
@@ -2449,7 +4204,7 @@
{
"data": {
"text/html": [
- "
"
+ "
"
],
"text/plain": [
""
@@ -2457,81 +4212,41 @@
},
"metadata": {},
"output_type": "display_data"
- }
- ],
- "source": [
- "fig = plt.figure(3)\n",
- "plt.imshow(im, interpolation='nearest')\n",
- "\n",
- "coords = []\n",
- "connectId = fig.canvas.mpl_connect('button_press_event', onclick)"
- ]
- },
- {
- "cell_type": "markdown",
- "metadata": {},
- "source": [
- "Notice that in the previous code cell, we created an empty list named `coords`, and inside the `onclick()` function, we are appending to it the $(x,y)$ coordinates of each mouse click on the figure. After executing the cell above, you have a connection to the figure, via the user interface: try clicking with your mouse on the endpoints of the white lines of the metered panel (click on the edge of the panel to get approximately equal $x$ coordinates), then print the contents of the `coords` list below."
- ]
- },
- {
- "cell_type": "code",
- "execution_count": 66,
- "metadata": {},
- "outputs": [
+ },
{
"data": {
"text/plain": [
- "[[229.51449092741933, 245.74974798387098],\n",
- " [229.51449092741933, 324.3787802419355],\n",
- " [325.44191028225805, 292.9271673387097],\n",
- " [364.7564264112903, 300.7900705645161],\n",
- " [380.4822328629032, 308.65297379032256],\n",
- " [367.90158770161287, 329.09652217741933],\n",
- " [339.59513608870964, 346.39490927419354],\n",
- " [339.59513608870964, 371.5561995967742],\n",
- " [364.7564264112903, 363.69329637096774],\n",
- " [309.7161038306451, 393.57232862903226],\n",
- " [308.14352318548384, 443.89490927419354],\n",
- " [408.7886844758064, 421.8787802419355],\n",
- " [473.26449092741933, 376.27394153225805],\n",
- " [465.40158770161287, 280.34652217741933],\n",
- " [496.8532006048387, 267.765877016129],\n",
- " [452.8209425403225, 242.6045866935484],\n",
- " [430.80481350806446, 220.5884576612903],\n",
- " [627.3773941532259, 187.56426411290323],\n",
- " [350.60320060483866, 176.5561995967742],\n",
- " [364.7564264112903, 206.43523185483872]]"
+ "[]"
]
},
- "execution_count": 66,
+ "execution_count": 45,
"metadata": {},
"output_type": "execute_result"
}
],
"source": [
- "coords"
+ "plt.figure()\n",
+ "plt.imshow(im2)\n",
+ "\n",
+ "plt.plot(hits2[:,0],hits2[:,1],'x')"
]
},
{
"cell_type": "markdown",
"metadata": {},
"source": [
- "The $x$ coordinates are pretty close, but there is some variation due to our shaky hand (or bad eyesight), and perhaps because the metered panel is not perfectly vertical. We can cast the `coords` list to a NumPy array, then grab all the first elements of the coordinate pairs, then get the standard deviation as an indication of our error in the mouse-click captures."
- ]
- },
- {
- "cell_type": "code",
- "execution_count": null,
- "metadata": {},
- "outputs": [],
- "source": [
- "hits = np.array(coords)"
+ "## Set the scale to convert pixels -> meters\n",
+ "\n",
+ "In the next two `imshow` commands, we will choose the corners of the target shown below as location 1, 2, 3, and 4 and the center we will determine from the average locations of locations 1-4 in $\\times$ . \n",
+ "\n",
+ "\n",
+ "\n",
+ "We use the same `onclick` function to create the `corners1` array for target_01.jpg and `corners2` for targete_02.jpg. "
]
},
{
"cell_type": "code",
- "execution_count": 81,
+ "execution_count": 25,
"metadata": {},
"outputs": [
{
@@ -3317,7 +5032,7 @@
{
"data": {
"text/html": [
- "
"
+ "
"
],
"text/plain": [
""
@@ -3325,49 +5040,28 @@
},
"metadata": {},
"output_type": "display_data"
- },
- {
- "data": {
- "text/plain": [
- "[]"
- ]
- },
- "execution_count": 81,
- "metadata": {},
- "output_type": "execute_result"
}
],
"source": [
- "plt.figure()\n",
- "plt.imshow(im)\n",
+ "fig = plt.figure()\n",
+ "plt.imshow(im1, interpolation='nearest')\n",
"\n",
- "plt.plot(hits[:,0],hits[:,1],'x')\n",
- "plt.plot(rings[:,0],rings[:,1],'s')"
+ "coords = []\n",
+ "connectId = fig.canvas.mpl_connect('button_press_event', onclick)"
]
},
{
"cell_type": "code",
- "execution_count": 73,
+ "execution_count": 26,
"metadata": {},
- "outputs": [
- {
- "data": {
- "text/plain": [
- "array([ 0, 8, 16, 24])"
- ]
- },
- "execution_count": 73,
- "metadata": {},
- "output_type": "execute_result"
- }
- ],
+ "outputs": [],
"source": [
- "rings"
+ "corners1 =np.array(coords)"
]
},
{
"cell_type": "code",
- "execution_count": 68,
+ "execution_count": 27,
"metadata": {},
"outputs": [
{
@@ -4153,7 +5847,7 @@
{
"data": {
"text/html": [
- "
"
+ "
"
],
"text/plain": [
""
@@ -4165,7 +5859,7 @@
],
"source": [
"fig = plt.figure()\n",
- "plt.imshow(im, interpolation='nearest')\n",
+ "plt.imshow(im2, interpolation='nearest')\n",
"\n",
"coords = []\n",
"connectId = fig.canvas.mpl_connect('button_press_event', onclick)"
@@ -4173,97 +5867,25 @@
},
{
"cell_type": "code",
- "execution_count": 78,
- "metadata": {},
- "outputs": [
- {
- "data": {
- "text/plain": [
- "array([[352.17578125, 283.00025202],\n",
- " [468.54674899, 240.5405746 ],\n",
- " [597.4983619 , 223.2421875 ],\n",
- " [728.02255544, 207.51638105]])"
- ]
- },
- "execution_count": 78,
- "metadata": {},
- "output_type": "execute_result"
- }
- ],
- "source": [
- "rings = np.array(coords)\n",
- "rings"
- ]
- },
- {
- "cell_type": "code",
- "execution_count": 79,
+ "execution_count": 28,
"metadata": {},
- "outputs": [
- {
- "data": {
- "text/plain": [
- "0.06351808135606307"
- ]
- },
- "execution_count": 79,
- "metadata": {},
- "output_type": "execute_result"
- }
- ],
+ "outputs": [],
"source": [
- "bull=rings[0,:]\n",
- "distances=np.sqrt((rings[:,0]-bull[0])**2+(rings[:,1]-bull[1])**2)\n",
- "ring_cm = np.array([0,8,16,24]) # ring distance from Bullseye cm\n",
- "scale =np.mean(ring_cm[1:]/distances[1:]) # scale for distances to cm/px\n",
- "scale"
+ "corners2 =np.array(coords)"
]
},
{
- "cell_type": "code",
- "execution_count": 82,
+ "cell_type": "markdown",
"metadata": {},
- "outputs": [
- {
- "data": {
- "text/plain": [
- "array([[ 7.79120982, 2.36608055],\n",
- " [ 7.79120982, -2.62828472],\n",
- " [ 1.69808419, -0.63053862],\n",
- " [ -0.79909844, -1.12997514],\n",
- " [ -1.7979715 , -1.62941167],\n",
- " [ -0.99887305, -2.92794664],\n",
- " [ 0.79909844, -4.026707 ],\n",
- " [ 0.79909844, -5.62490388],\n",
- " [ -0.79909844, -5.12546736],\n",
- " [ 2.69695724, -7.02332616],\n",
- " [ 2.79684455, -10.21971993],\n",
- " [ -3.59594299, -8.82129765],\n",
- " [ -7.69132251, -5.9245658 ],\n",
- " [ -7.19188599, 0.16855983],\n",
- " [ -9.18963209, 0.96765827],\n",
- " [ -6.39278754, 2.56585516],\n",
- " [ -4.99436527, 3.96427743],\n",
- " [-17.48027844, 6.06191084],\n",
- " [ 0.09988731, 6.76112198],\n",
- " [ -0.79909844, 4.86326318]])"
- ]
- },
- "execution_count": 82,
- "metadata": {},
- "output_type": "execute_result"
- }
- ],
"source": [
- "hit_scale=hits\n",
- "hit_scale[:,0]=(bull[0]-hits[:,0])*scale\n",
- "hit_scale[:,1]=(bull[1]-hits[:,1])*scale\n",
- "hits"
+ "## Set the absolute position and scale results\n",
+ "\n",
+ "With the corner locations save in the `corners1` and `corners2`, we locate the target center as the average x-position and average y-position of all four chosen points. Below, we plot the corners and centers for both images, you can change the `imshow` command to check target_01.jpg and target_02.jpg. "
]
},
{
"cell_type": "code",
- "execution_count": 83,
+ "execution_count": 33,
"metadata": {},
"outputs": [
{
@@ -5049,7 +6671,7 @@
{
"data": {
"text/html": [
- "
"
+ "
"
],
"text/plain": [
""
@@ -5061,23 +6683,97 @@
{
"data": {
"text/plain": [
- "[]"
+ "Text(0, 0.5, 'x-axis')"
]
},
- "execution_count": 83,
+ "execution_count": 33,
"metadata": {},
"output_type": "execute_result"
}
],
"source": [
"plt.figure()\n",
- "plt.plot(hit_scale[:,0],hit_scale[:,1],'o')\n",
- "plt.plot(np.mean(hit_scale[:,0]),np.mean(hit_scale[:,1]),'s')"
+ "\n",
+ "center1_x = np.mean(corners1[:,0])\n",
+ "center1_y = np.mean(corners1[:,1])\n",
+ "\n",
+ "center2_x = np.mean(corners2[:,0])\n",
+ "center2_y = np.mean(corners2[:,1])\n",
+ "\n",
+ "plt.imshow(im1)\n",
+ "\n",
+ "plt.plot(corners1[:,0],corners1[:,1],'o')\n",
+ "plt.plot(corners2[:,0],corners2[:,1],'s')\n",
+ "\n",
+ "plt.plot(center1_x,center1_y,'+')\n",
+ "plt.plot(center2_x,center2_y,'x')\n",
+ "plt.plot(1500,2500,'s')\n",
+ "plt.xlabel('y-axis')\n",
+ "plt.ylabel('x-axis')"
+ ]
+ },
+ {
+ "cell_type": "markdown",
+ "metadata": {},
+ "source": [
+ "You can see above that each image will have a different scale to convert from position in pixels to position in meters. This is the case for all quantitative image data, every time a camera is repositioned you will have a new scale to convert pixels to meters. \n",
+ "\n",
+ "Below, we use the 0.9144-m distance between corner locations to set oour `im1_scale` and `im2_scale` for target_01.jpg and target_02.jpg, respectively. "
+ ]
+ },
+ {
+ "cell_type": "code",
+ "execution_count": 39,
+ "metadata": {},
+ "outputs": [
+ {
+ "name": "stdout",
+ "output_type": "stream",
+ "text": [
+ "target_01.jpg has conversion 0.00059 meter/pixel\n",
+ "target_02.jpg has conversion 0.00048 meter/pixel\n"
+ ]
+ }
+ ],
+ "source": [
+ "d1=np.sqrt((corners1[0,0]-corners1[1,0])**2+(corners1[0,1]-corners1[1,1])**2)\n",
+ "d2=np.sqrt((corners1[2,0]-corners1[3,0])**2+(corners1[2,1]-corners1[3,1])**2)\n",
+ "\n",
+ "\n",
+ "im1_scale = 0.9144/np.mean([d1,d2]) # in 0.9144 meters/ pixel distance\n",
+ "print('target_01.jpg has conversion {:.2} meter/pixel'.format(im1_scale))\n",
+ "d1=np.sqrt((corners2[0,0]-corners2[1,0])**2+(corners2[0,1]-corners2[1,1])**2)\n",
+ "d2=np.sqrt((corners2[2,0]-corners2[3,0])**2+(corners2[2,1]-corners2[3,1])**2)\n",
+ "\n",
+ "\n",
+ "im2_scale = 0.9144/np.mean([d1,d2]) # in 0.9144 meters/ pixel distance\n",
+ "print('target_02.jpg has conversion {:.2} meter/pixel'.format(im2_scale))"
+ ]
+ },
+ {
+ "cell_type": "markdown",
+ "metadata": {},
+ "source": [
+ "# Final Data preparation\n",
+ "\n",
+ "So far, we have\n",
+ "\n",
+ "1. Chosen hit points on two images\n",
+ "\n",
+ "2. Chosen calibration points on two images\n",
+ "\n",
+ "2. Determined a local reference in each image i.e. point (0,0)\n",
+ "\n",
+ "3. Determined the scale for each image i.e. convert pixels -> meters\n",
+ "\n",
+ "The final data preparation is to convert the chosen hit points on the two images into (x,y) locations in meters. We will save some other information in case we need to check our work later _(part of creating reproducible research)_.\n",
+ "\n",
+ "The first step is to plot our scaled and centered data from targets 01 and 02. We can also plot the mean location of our hits from the class. "
]
},
{
"cell_type": "code",
- "execution_count": 98,
+ "execution_count": 42,
"metadata": {},
"outputs": [
{
@@ -5863,7 +7559,7 @@
{
"data": {
"text/html": [
- "
"
+ "
"
],
"text/plain": [
""
@@ -5875,89 +7571,83 @@
{
"data": {
"text/plain": [
- "(array([1., 1., 0., 2., 0., 1., 0., 1., 1., 1.]),\n",
- " array([0.44381555, 1.12382265, 1.80382975, 2.48383685, 3.16384395,\n",
- " 3.84385105, 4.52385815, 5.20386525, 5.88387235, 6.56387946,\n",
- " 7.24388656]),\n",
- " )"
+ ""
]
},
- "execution_count": 98,
+ "execution_count": 42,
"metadata": {},
"output_type": "execute_result"
}
],
"source": [
- "angle=np.arctan2(hit_scale[:,1],hit_scale[:,0])\n",
- "radius=np.sqrt(hit_scale[:,1]**2+hit_scale[:,0]**2)\n",
+ "hits1=np.load('./hits_im1.npy')\n",
+ "hits2=np.load('./hits_im2.npy')\n",
+ "\n",
+ "center_hits1=hits1\n",
+ "center_hits1[:,0]= hits1[:,0]-center1_x\n",
+ "center_hits1[:,1]= hits1[:,1]-center1_y\n",
+ "\n",
+ "center_hits2=hits2\n",
+ "center_hits2[:,0]= hits2[:,0]-center2_x\n",
+ "center_hits2[:,1]= hits2[:,1]-center2_y\n",
+ "\n",
+ "scaled_hits1 = center_hits1*im1_scale\n",
+ "scaled_hits2 = center_hits2*im2_scale\n",
+ "\n",
"plt.figure()\n",
- "#plt.hist(angle)\n",
- "plt.hist(radius)\n"
+ "plt.plot(scaled_hits1[:,0],scaled_hits1[:,1],'x')\n",
+ "plt.plot(scaled_hits2[:,0],scaled_hits2[:,1],'x')\n",
+ "\n",
+ "plt.plot(np.mean(scaled_hits1[:,0]),np.mean(scaled_hits1[:,1]),'o',label='mean hit target_01')\n",
+ "plt.plot(np.mean(scaled_hits2[:,0]),np.mean(scaled_hits2[:,1]),'o',label='mean hit target_02')\n",
+ "plt.legend()\n"
]
},
{
"cell_type": "markdown",
"metadata": {},
"source": [
- "Looking ahead, what we'll do is repeat the process of capturing mouse clicks on the image, but clicking on the ball positions. Then, we will want to have the vertical positions converted to physical length (in meters), from the pixel numbers on the image.\n",
+ "The locations look good so far, now we export the data to a csv file so we can access it later. \n",
"\n",
- "You can get the scaling from pixels to meters via the distance between two white lines on the metered panel, which we know is $0.25\\rm{m}$. \n",
+ "The csv file will save the following information:\n",
"\n",
- "Let's get the average vertical distance between two while lines, which we can calculate as:\n",
- "\n",
- "\\begin{equation}\n",
- "\\overline{\\Delta y} = \\sum_{i=0}^N \\frac{y_{i+1}-y_i}{N-1}\n",
- "\\end{equation}"
+ "|throw #| x position (m)| y position (m)|picture x position (pixel)| picture y position (pixel)|target x position (pixel)| target y position (pixel)| image #|\n",
+ "|-------|--------------|---------------|-------|----------|---------|----------|--------|\n",
+ "|arbitrary hit #|centered x position|centered y position|absolute x pixel location|absoluter y pixel location|target center x pixel location|target center y pixel location |either 1 or 2 for target_01.jpg or target_02.jpg|\n",
+ "\n"
]
},
{
"cell_type": "code",
- "execution_count": 17,
+ "execution_count": 43,
"metadata": {},
- "outputs": [
- {
- "data": {
- "text/plain": [
- "123.56215213358072"
- ]
- },
- "execution_count": 17,
- "metadata": {},
- "output_type": "execute_result"
- }
- ],
+ "outputs": [],
"source": [
- "gap_lines = y_lines[1:] - y_lines[0:-1]\n",
- "gap_lines.mean()"
+ "hits1=np.load('../data/hits_im1.npy')\n",
+ "hits2=np.load('../data/hits_im2.npy')\n",
+ "file = open('../data/target_data.csv','w')\n",
+ "file.write('throw #, x position (m), y position (m),')\n",
+ "file.write('picture x position (pixel), picture y position (pixel),')\n",
+ "file.write('target x position (pixel), target y position (pixel), image #\\n')\n",
+ "for i in range(0,len(scaled_hits1)):\n",
+ " file.write('{},{},{},'.format(i,scaled_hits1[i,0],scaled_hits1[i,1]))\n",
+ " file.write('{},{},'.format(hits1[i,0],hits1[i,1]))\n",
+ " file.write('{},{},{}\\n'.format(center1_x,center1_y,1))\n",
+ "for i in range(0,len(scaled_hits2)):\n",
+ " file.write('{},{},{},'.format(len(scaled_hits1)+i,scaled_hits2[i,0],scaled_hits2[i,1]))\n",
+ " file.write('{},{},'.format(hits2[i,0],hits2[i,1]))\n",
+ " file.write('{},{},{}\\n'.format(center2_x,center2_y,2))\n",
+ "file.close()"
]
},
{
- "cell_type": "code",
- "execution_count": null,
- "metadata": {},
- "outputs": [],
- "source": []
- },
- {
- "cell_type": "code",
- "execution_count": null,
- "metadata": {},
- "outputs": [],
- "source": []
- },
- {
- "cell_type": "code",
- "execution_count": null,
- "metadata": {},
- "outputs": [],
- "source": []
- },
- {
- "cell_type": "code",
- "execution_count": null,
+ "cell_type": "markdown",
"metadata": {},
- "outputs": [],
- "source": []
+ "source": [
+ "# Congratulations\n",
+ "\n",
+ "Now you can do some statistics on accuracy and precision of the class by loading the csv into a `pandas` dataframe. "
+ ]
}
],
"metadata": {