Permalink
Cannot retrieve contributors at this time
Name already in use
A tag already exists with the provided branch name. Many Git commands accept both tag and branch names, so creating this branch may cause unexpected behavior. Are you sure you want to create this branch?
ME3255S2017/lecture_13/lecture_13.md
Go to fileThis commit does not belong to any branch on this repository, and may belong to a fork outside of the repository.
962 lines (624 sloc)
17.4 KB
This file contains bidirectional Unicode text that may be interpreted or compiled differently than what appears below. To review, open the file in an editor that reveals hidden Unicode characters.
Learn more about bidirectional Unicode characters
```octave | |
%plot --format svg | |
``` | |
```octave | |
setdefaults | |
``` | |
## My question from last class | |
 | |
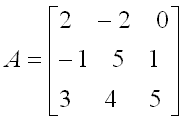 | |
 | |
## Your questions from last class | |
1. Do we have to submit a link for HW #4 somewhere or is uploading to Github sufficient? | |
-no, your submission from HW 3 is sufficient | |
2. How do I get the formulas/formatting in markdown files to show up on github? | |
-no luck for markdown equations in github, this is an ongoing request | |
3. Confused about the p=1 norm part and ||A||_1 | |
4. When's the exam? | |
-next week (3/9) | |
5. What do you recommend doing to get better at figuring out the homeworks? | |
-time and experimenting (try going through the lecture examples, verify my work) | |
6. Could we have an hw or extra credit with a video lecture to learn some simple python? | |
-Sounds great! how simple? | |
-[Installing Python and Jupyter Notebook (via Anaconda) - https://www.continuum.io/downloads](https://www.continuum.io/downloads) | |
-[Running Matlab kernel in Jupyter - https://anneurai.net/2015/11/12/matlab-based-ipython-notebooks/](https://anneurai.net/2015/11/12/matlab-based-ipython-notebooks/) | |
-[Running Octave kernel in Jupyter - https://anaconda.org/pypi/octave_kernel](https://anaconda.org/pypi/octave_kernel) | |
# Markdown examples | |
` " ' ` ` | |
```matlab | |
x=linspace(0,1); | |
y=x.^2; | |
plot(x,y) | |
for i = 1:10 | |
fprintf('markdown is pretty') | |
end | |
``` | |
## Condition of a matrix | |
### *just checked in to see what condition my condition was in* | |
### Matrix norms | |
The Euclidean norm of a vector is measure of the magnitude (in 3D this would be: $|x|=\sqrt{x_{1}^{2}+x_{2}^{2}+x_{3}^{2}}$) in general the equation is: | |
$||x||_{e}=\sqrt{\sum_{i=1}^{n}x_{i}^{2}}$ | |
For a matrix, A, the same norm is called the Frobenius norm: | |
$||A||_{f}=\sqrt{\sum_{i=1}^{n}\sum_{j=1}^{m}A_{i,j}^{2}}$ | |
In general we can calculate any $p$-norm where | |
$||A||_{p}=\sqrt{\sum_{i=1}^{n}\sum_{i=1}^{m}A_{i,j}^{p}}$ | |
so the p=1, 1-norm is | |
$||A||_{1}=\sqrt{\sum_{i=1}^{n}\sum_{i=1}^{m}A_{i,j}^{1}}=\sum_{i=1}^{n}\sum_{i=1}^{m}|A_{i,j}|$ | |
$||A||_{\infty}=\sqrt{\sum_{i=1}^{n}\sum_{i=1}^{m}A_{i,j}^{\infty}}=\max_{1\le i \le n}\sum_{j=1}^{m}|A_{i,j}|$ | |
### Condition of Matrix | |
The matrix condition is the product of | |
$Cond(A) = ||A||\cdot||A^{-1}||$ | |
So each norm will have a different condition number, but the limit is $Cond(A)\ge 1$ | |
An estimate of the rounding error is based on the condition of A: | |
$\frac{||\Delta x||}{x} \le Cond(A) \frac{||\Delta A||}{||A||}$ | |
So if the coefficients of A have accuracy to $10^{-t} | |
and the condition of A, $Cond(A)=10^{c}$ | |
then the solution for x can have rounding errors up to $10^{c-t}$ | |
```octave | |
A=[1,1/2,1/3;1/2,1/3,1/4;1/3,1/4,1/5] | |
[L,U]=LU_naive(A) | |
``` | |
A = | |
1.00000 0.50000 0.33333 | |
0.50000 0.33333 0.25000 | |
0.33333 0.25000 0.20000 | |
L = | |
1.00000 0.00000 0.00000 | |
0.50000 1.00000 0.00000 | |
0.33333 1.00000 1.00000 | |
U = | |
1.00000 0.50000 0.33333 | |
0.00000 0.08333 0.08333 | |
0.00000 -0.00000 0.00556 | |
Then, $A^{-1}=(LU)^{-1}=U^{-1}L^{-1}$ | |
$Ld_{1}=\left[\begin{array}{c} | |
1 \\ | |
0 \\ | |
0 \end{array}\right]$, $Ux_{1}=d_{1}$ ... | |
```octave | |
invA=zeros(3,3); | |
d1=L\[1;0;0]; | |
d2=L\[0;1;0]; | |
d3=L\[0;0;1]; | |
invA(:,1)=U\d1; % shortcut invA(:,1)=A\[1;0;0] | |
invA(:,2)=U\d2; | |
invA(:,3)=U\d3 | |
invA*A | |
``` | |
invA = | |
9.0000 -36.0000 30.0000 | |
-36.0000 192.0000 -180.0000 | |
30.0000 -180.0000 180.0000 | |
ans = | |
1.0000e+00 3.5527e-15 2.9976e-15 | |
-1.3249e-14 1.0000e+00 -9.1038e-15 | |
8.5117e-15 7.1054e-15 1.0000e+00 | |
Find the condition of A, $cond(A)$ | |
```octave | |
% Frobenius norm | |
normf_A = sqrt(sum(sum(A.^2))) | |
normf_invA = sqrt(sum(sum(invA.^2))) | |
cond_f_A = normf_A*normf_invA | |
norm(A,'fro') | |
% p=1, column sum norm | |
norm1_A = max(sum(A,2)) | |
norm1_invA = max(sum(invA,2)) | |
norm(A,1) | |
cond_1_A=norm1_A*norm1_invA | |
% p=inf, row sum norm | |
norminf_A = max(sum(A,1)) | |
norminf_invA = max(sum(invA,1)) | |
norm(A,inf) | |
cond_inf_A=norminf_A*norminf_invA | |
``` | |
normf_A = 1.4136 | |
normf_invA = 372.21 | |
cond_f_A = 526.16 | |
ans = 1.4136 | |
norm1_A = 1.8333 | |
norm1_invA = 30.000 | |
ans = 1.8333 | |
cond_1_A = 55.000 | |
norminf_A = 1.8333 | |
norminf_invA = 30.000 | |
ans = 1.8333 | |
cond_inf_A = 55.000 | |
Consider the problem again from the intro to Linear Algebra, 4 masses are connected in series to 4 springs with spring constants $K_{i}$. What does a high condition number mean for this problem? | |
 | |
The masses haves the following amounts, 1, 2, 3, and 4 kg for masses 1-4. Using a FBD for each mass: | |
$m_{1}g+k_{2}(x_{2}-x_{1})-k_{1}x_{1}=0$ | |
$m_{2}g+k_{3}(x_{3}-x_{2})-k_{2}(x_{2}-x_{1})=0$ | |
$m_{3}g+k_{4}(x_{4}-x_{3})-k_{3}(x_{3}-x_{2})=0$ | |
$m_{4}g-k_{4}(x_{4}-x_{3})=0$ | |
in matrix form: | |
$\left[ \begin{array}{cccc} | |
k_{1}+k_{2} & -k_{2} & 0 & 0 \\ | |
-k_{2} & k_{2}+k_{3} & -k_{3} & 0 \\ | |
0 & -k_{3} & k_{3}+k_{4} & -k_{4} \\ | |
0 & 0 & -k_{4} & k_{4} \end{array} \right] | |
\left[ \begin{array}{c} | |
x_{1} \\ | |
x_{2} \\ | |
x_{3} \\ | |
x_{4} \end{array} \right]= | |
\left[ \begin{array}{c} | |
m_{1}g \\ | |
m_{2}g \\ | |
m_{3}g \\ | |
m_{4}g \end{array} \right]$ | |
```octave | |
k1=10; % N/m | |
k2=100000; | |
k3=10; | |
k4=1; | |
m1=1; % kg | |
m2=2; | |
m3=3; | |
m4=4; | |
g=9.81; % m/s^2 | |
K=[k1+k2 -k2 0 0; -k2 k2+k3 -k3 0; 0 -k3 k3+k4 -k4; 0 0 -k4 k4] | |
y=[m1*g;m2*g;m3*g;m4*g] | |
``` | |
K = | |
100010 -100000 0 0 | |
-100000 100010 -10 0 | |
0 -10 11 -1 | |
0 0 -1 1 | |
y = | |
9.8100 | |
19.6200 | |
29.4300 | |
39.2400 | |
```octave | |
cond(K,inf) | |
cond(K,1) | |
cond(K,'fro') | |
cond(K,2) | |
``` | |
ans = 3.2004e+05 | |
ans = 3.2004e+05 | |
ans = 2.5925e+05 | |
ans = 2.5293e+05 | |
```octave | |
e=eig(K) | |
max(e)/min(e) | |
``` | |
e = | |
7.9078e-01 | |
3.5881e+00 | |
1.7621e+01 | |
2.0001e+05 | |
ans = 2.5293e+05 | |
## P=2 norm is ratio of biggest eigenvalue to smallest eigenvalue! | |
no need to calculate the inv(K) | |
# Iterative Methods | |
## Gauss-Seidel method | |
If we have an intial guess for each value of a vector $x$ that we are trying to solve, then it is easy enough to solve for one component given the others. | |
Take a 3$\times$3 matrix | |
$Ax=b$ | |
$\left[ \begin{array}{ccc} | |
3 & -0.1 & -0.2 \\ | |
0.1 & 7 & -0.3 \\ | |
0.3 & -0.2 & 10 \end{array} \right] | |
\left[ \begin{array}{c} | |
x_{1} \\ | |
x_{2} \\ | |
x_{3} \end{array} \right]= | |
\left[ \begin{array}{c} | |
7.85 \\ | |
-19.3 \\ | |
71.4\end{array} \right]$ | |
$x_{1}=\frac{7.85+0.1x_{2}+0.2x_{3}}{3}$ | |
$x_{2}=\frac{-19.3-0.1x_{1}+0.3x_{3}}{7}$ | |
$x_{3}=\frac{71.4+0.1x_{1}+0.2x_{2}}{10}$ | |
```octave | |
A=[3 -0.1 -0.2;0.1 7 -0.3;0.3 -0.2 10] | |
b=[7.85;-19.3;71.4] | |
x=A\b | |
``` | |
A = | |
3.00000 -0.10000 -0.20000 | |
0.10000 7.00000 -0.30000 | |
0.30000 -0.20000 10.00000 | |
b = | |
7.8500 | |
-19.3000 | |
71.4000 | |
x = | |
3.0000 | |
-2.5000 | |
7.0000 | |
### Gauss-Seidel Iterative approach | |
As a first guess, we can use $x_{1}=x_{2}=x_{3}=0$ | |
$x_{1}=\frac{7.85+0.1(0)+0.3(0)}{3}=2.6167$ | |
$x_{2}=\frac{-19.3-0.1(2.6167)+0.3(0)}{7}=-2.7945$ | |
$x_{3}=\frac{71.4+0.1(2.6167)+0.2(-2.7945)}{10}=7.0056$ | |
Then, we update the guess: | |
$x_{1}=\frac{7.85+0.1(-2.7945)+0.3(7.0056)}{3}=2.9906$ | |
$x_{2}=\frac{-19.3-0.1(2.9906)+0.3(7.0056)}{7}=-2.4996$ | |
$x_{3}=\frac{71.4+0.1(2.9906)+0.2(-2.4966)}{10}=7.00029$ | |
The results are conveerging to the solution we found with `\` of $x_{1}=3,~x_{2}=-2.5,~x_{3}=7$ | |
We could also use an iterative method that solves for all of the x-components in one step: | |
### Jacobi method | |
$x_{1}^{i}=\frac{7.85+0.1x_{2}^{i-1}+0.3x_{3}^{i-1}}{3}$ | |
$x_{2}^{i}=\frac{-19.3-0.1x_{1}^{i-1}+0.3x_{3}^{i-1}}{7}$ | |
$x_{3}^{i}=\frac{71.4+0.1x_{1}^{i-1}+0.2x_{2}^{i-1}}{10}$ | |
Here the solution is a matrix multiplication and vector addition | |
$\left[ \begin{array}{c} | |
x_{1}^{i} \\ | |
x_{2}^{i} \\ | |
x_{3}^{i} \end{array} \right]= | |
\left[ \begin{array}{c} | |
7.85/3 \\ | |
-19.3/7 \\ | |
71.4/10\end{array} \right]- | |
\left[ \begin{array}{ccc} | |
0 & 0.1/3 & 0.2/3 \\ | |
0.1/7 & 0 & -0.3/7 \\ | |
0.3/10 & -0.2/10 & 0 \end{array} \right] | |
\left[ \begin{array}{c} | |
x_{1}^{i-1} \\ | |
x_{2}^{i-1} \\ | |
x_{3}^{i-1} \end{array} \right]$ | |
|x_{j}|Jacobi method |vs| Gauss-Seidel | | |
|--------|------------------------------|---|-------------------------------| | |
|$x_{1}^{i}=$ | $\frac{7.85+0.1x_{2}^{i-1}+0.3x_{3}^{i-1}}{3}$ | | $\frac{7.85+0.1x_{2}^{i-1}+0.3x_{3}^{i-1}}{3}$| | |
|$x_{2}^{i}=$ | $\frac{-19.3-0.1x_{1}^{i-1}+0.3x_{3}^{i-1}}{7}$ | | $\frac{-19.3-0.1x_{1}^{i}+0.3x_{3}^{i-1}}{7}$ | | |
|$x_{3}^{i}=$ | $\frac{71.4+0.1x_{1}^{i-1}+0.2x_{2}^{i-1}}{10}$ | | $\frac{71.4+0.1x_{1}^{i}+0.2x_{2}^{i}}{10}$| | |
```octave | |
ba=b./diag(A) % or ba=b./[A(1,1);A(2,2);A(3,3)] | |
sA=A-diag(diag(A)) % A with zeros on diagonal | |
sA(1,:)=sA(1,:)/A(1,1); | |
sA(2,:)=sA(2,:)/A(2,2); | |
sA(3,:)=sA(3,:)/A(3,3) | |
x0=[0;0;0]; | |
x1=ba-sA*x0 | |
x2=ba-sA*x1 | |
x3=ba-sA*x2 | |
fprintf('solution is converging to [3,-2.5,7]]\n') | |
``` | |
ba = | |
2.6167 | |
-2.7571 | |
7.1400 | |
sA = | |
0.00000 -0.10000 -0.20000 | |
0.10000 0.00000 -0.30000 | |
0.30000 -0.20000 0.00000 | |
sA = | |
0.000000 -0.033333 -0.066667 | |
0.014286 0.000000 -0.042857 | |
0.030000 -0.020000 0.000000 | |
x1 = | |
2.6167 | |
-2.7571 | |
7.1400 | |
x2 = | |
3.0008 | |
-2.4885 | |
7.0064 | |
x3 = | |
3.0008 | |
-2.4997 | |
7.0002 | |
solution is converging to [3,-2.5,7]] | |
```octave | |
diag(A) | |
diag(diag(A)) | |
``` | |
ans = | |
3 | |
7 | |
10 | |
ans = | |
Diagonal Matrix | |
3 0 0 | |
0 7 0 | |
0 0 10 | |
This method works if problem is diagonally dominant, | |
$|a_{ii}|>\sum_{j=1,j\ne i}^{n}|a_{ij}|$ | |
If this condition is true, then Jacobi or Gauss-Seidel should converge | |
```octave | |
A=[0.1,1,3;1,0.2,3;5,2,0.3] | |
b=[12;2;4] | |
A\b | |
``` | |
A = | |
0.10000 1.00000 3.00000 | |
1.00000 0.20000 3.00000 | |
5.00000 2.00000 0.30000 | |
b = | |
12 | |
2 | |
4 | |
ans = | |
-2.9393 | |
9.1933 | |
1.0336 | |
```octave | |
ba=b./diag(A) % or ba=b./[A(1,1);A(2,2);A(3,3)] | |
sA=A-diag(diag(A)) % A with zeros on diagonal | |
sA(1,:)=sA(1,:)/A(1,1); | |
sA(2,:)=sA(2,:)/A(2,2); | |
sA(3,:)=sA(3,:)/A(3,3) | |
x0=[0;0;0]; | |
x1=ba-sA*x0 | |
x2=ba-sA*x1 | |
x3=ba-sA*x2 | |
fprintf('solution is not converging to [-2.93,9.19,1.03]\n') | |
``` | |
ba = | |
120.000 | |
10.000 | |
13.333 | |
sA = | |
0 1 3 | |
1 0 3 | |
5 2 0 | |
sA = | |
0.00000 10.00000 30.00000 | |
5.00000 0.00000 15.00000 | |
16.66667 6.66667 0.00000 | |
x1 = | |
120.000 | |
10.000 | |
13.333 | |
x2 = | |
-380.00 | |
-790.00 | |
-2053.33 | |
x3 = | |
6.9620e+04 | |
3.2710e+04 | |
1.1613e+04 | |
solution is not converging to [-2.93,9.19,1.03] | |
## Gauss-Seidel with Relaxation | |
In order to force the solution to converge faster, we can introduce a relaxation term $\lambda$. | |
where the new x values are weighted between the old and new: | |
$x^{i}=\lambda x^{i}+(1-\lambda)x^{i-1}$ | |
after solving for x, lambda weights the current approximation with the previous approximation for the updated x | |
```octave | |
% rearrange A and b | |
A=[3 -0.1 -0.2;0.1 7 -0.3;0.3 -0.2 10] | |
b=[7.85;-19.3;71.4] | |
iters=zeros(100,1); | |
for i=1:100 | |
lambda=2/100*i; | |
[x,ea,iters(i)]=Jacobi_rel(A,b,lambda); | |
end | |
plot([1:100]*2/100,iters) | |
``` | |
A = | |
3.00000 -0.10000 -0.20000 | |
0.10000 7.00000 -0.30000 | |
0.30000 -0.20000 10.00000 | |
b = | |
7.8500 | |
-19.3000 | |
71.4000 | |
 | |
```octave | |
l=fminbnd(@(l) lambda_fcn(A,b,l),0.5,1.5) | |
``` | |
l = 0.99158 | |
```octave | |
A\b | |
``` | |
ans = | |
3.0000 | |
-2.5000 | |
7.0000 | |
```octave | |
[x,ea,iter]=Jacobi_rel(A,b,l,0.000001) | |
[x,ea,iter]=Jacobi_rel(A,b,1,0.000001) | |
``` | |
x = | |
3.0000 | |
-2.5000 | |
7.0000 | |
ea = | |
1.8289e-07 | |
2.1984e-08 | |
2.3864e-08 | |
iter = 8 | |
x = | |
3.0000 | |
-2.5000 | |
7.0000 | |
ea = | |
1.9130e-08 | |
7.6449e-08 | |
3.3378e-08 | |
iter = 8 | |
## Nonlinear Systems | |
Consider two simultaneous nonlinear equations with two unknowns: | |
$x_{1}^{2}+x_{1}x_{2}=10$ | |
$x_{2}+3x_{1}x_{2}^{2}=57$ | |
Graphically, we are looking for the solution: | |
```octave | |
x11=linspace(0.5,3); | |
x12=(10-x11.^2)./x11; | |
x22=linspace(2,8); | |
x21=(57-x22).*x22.^-2/3; | |
plot(x11,x12,x21,x22) | |
% Solution at x_1=2, x_2=3 | |
hold on; | |
plot(2,3,'o') | |
xlabel('x_1') | |
ylabel('x_2') | |
``` | |
 | |
## Newton-Raphson part II | |
Remember the first order approximation for the next point in a function is: | |
$f(x_{i+1})=f(x_{i})+(x_{i+1}-x_{i})f'(x_{i})$ | |
then, $f(x_{i+1})=0$ so we are left with: | |
$x_{i+1}=x_{i}-\frac{f(x_{i})}{f'(x_{i})}$ | |
We can use the same formula, but now we have multiple dimensions so we need to determine the Jacobian | |
$[J]=\left[ \begin{array}{cccc} | |
\frac{\partial f_{1,i}}{\partial x_{1}} & \frac{\partial f_{1,i}}{\partial x_{2}} & | |
\cdots & \frac{\partial f_{1,i}}{\partial x_{n}} \\ | |
\frac{\partial f_{2,i}}{\partial x_{1}} & \frac{\partial f_{2,i}}{\partial x_{2}} & | |
\cdots & \frac{\partial f_{2,i}}{\partial x_{n}} \\ | |
\vdots & \vdots & & \vdots \\ | |
\frac{\partial f_{n,i}}{\partial x_{1}} & \frac{\partial f_{n,i}}{\partial x_{2}} & | |
\cdots & \frac{\partial f_{n,i}}{\partial x_{n}} \\ | |
\end{array} \right]$ | |
$\left[ \begin{array}{c} | |
f_{1,i+1} \\ | |
f_{2,i+1} \\ | |
\vdots \\ | |
f_{n,i+1}\end{array} \right]= | |
\left[ \begin{array}{c} | |
f_{1,i} \\ | |
f_{2,i} \\ | |
\vdots \\ | |
f_{n,i}\end{array} \right]+ | |
\left[ \begin{array}{cccc} | |
\frac{\partial f_{1,i}}{\partial x_{1}} & \frac{\partial f_{1,i}}{\partial x_{2}} & | |
\cdots & \frac{\partial f_{1,i}}{\partial x_{n}} \\ | |
\frac{\partial f_{2,i}}{\partial x_{1}} & \frac{\partial f_{2,i}}{\partial x_{2}} & | |
\cdots & \frac{\partial f_{2,i}}{\partial x_{n}} \\ | |
\vdots & \vdots & & \vdots \\ | |
\frac{\partial f_{n,i}}{\partial x_{1}} & \frac{\partial f_{n,i}}{\partial x_{2}} & | |
\cdots & \frac{\partial f_{n,i}}{\partial x_{n}} \\ | |
\end{array} \right] | |
\left( \left[ \begin{array}{c} | |
x_{i+1} \\ | |
x_{i+1} \\ | |
\vdots \\ | |
x_{i+1}\end{array} \right]- | |
\left[ \begin{array}{c} | |
x_{1,i} \\ | |
x_{2,i} \\ | |
\vdots \\ | |
x_{n,i}\end{array} \right]\right)$ | |
### Solution is again in the form Ax=b | |
$[J]([x_{i+1}]-[x_{i}])=-[f]$ | |
so | |
$[x_{i+1}]= [x_{i}]-[J]^{-1}[f]$ | |
## Example of Jacobian calculation | |
### Nonlinear springs supporting two masses in series | |
Two springs are connected to two masses, with $m_1$=1 kg and $m_{2}$=2 kg. The springs are identical, but they have nonlinear spring constants, of $k_1$=100 N/m and $k_2$=-10 N/m | |
We want to solve for the final position of the masses ($x_1$ and $x_2$) | |
$m_{1}g+k_{1}(x_{2}-x_{1})+k_{2}(x_{2}-x_{1})^{2}+k_{1}x_{1}+k_{2}x_{1}^{2}=0$ | |
$m_{2}g-k_{1}(x_{2}-x_{1})-k_{2}(x_2-x_1)^{2}=0$ | |
$J(1,1)=\frac{\partial f_{1}}{\partial x_{1}}=-k_{1}-2k_{2}(x_{2}-x_{1})+k_{1}+2k_{2}x_{1}$ | |
$J(1,2)=\frac{\partial f_1}{\partial x_{2}}=k_{1}+2k_{2}(x_{2}-x_{1})$ | |
$J(2,1)=\frac{\partial f_2}{\partial x_{1}}=k_{1}+2k_{2}(x_{2}-x_{1})$ | |
$J(2,2)=\frac{\partial f_2}{\partial x_{2}}=-k_{1}-2k_{2}(x_{2}-x_{1})$ | |
```octave | |
m1=1; % kg | |
m2=2; % kg | |
k1=100; % N/m | |
k2=-10; % N/m^2 | |
``` | |
```octave | |
function [f,J]=mass_spring(x) | |
% Function to calculate function values f1 and f2 as well as Jacobian | |
% for 2 masses and 2 identical nonlinear springs | |
m1=1; % kg | |
m2=2; % kg | |
k1=100; % N/m | |
k2=-10; % N/m^2 | |
g=9.81; % m/s^2 | |
x1=x(1); | |
x2=x(2); | |
J=[-k1-2*k2*(x2-x1)-k1-2*k2*x1,k1+2*k2*(x2-x1); | |
k1+2*k2*(x2-x1),-k1-2*k2*(x2-x1)]; | |
f=[m1*g+k1*(x2-x1)+k2*(x2-x1).^2-k1*x1-k2*x1^2; | |
m2*g-k1*(x2-x1)-k2*(x2-x1).^2]; | |
end | |
``` | |
```octave | |
[f,J]=mass_spring([1,0]) | |
``` | |
f = | |
-190.19 | |
129.62 | |
J = | |
-200 120 | |
120 -120 | |
```octave | |
x0=[3;2]; | |
[f0,J0]=mass_spring(x0); | |
x1=x0-J0\f0 | |
ea=(x1-x0)./x1 | |
[f1,J1]=mass_spring(x1); | |
x2=x1-J1\f1 | |
ea=(x2-x1)./x2 | |
[f2,J2]=mass_spring(x2); | |
x3=x2-J2\f2 | |
ea=(x3-x2)./x3 | |
x=x3 | |
for i=1:3 | |
xold=x; | |
[f,J]=mass_spring(x); | |
x=x-J\f; | |
ea=(x-xold)./x | |
end | |
``` | |
x1 = | |
-1.5142 | |
-1.4341 | |
ea = | |
2.9812 | |
2.3946 | |
x2 = | |
0.049894 | |
0.248638 | |
ea = | |
31.3492 | |
6.7678 | |
x3 = | |
0.29701 | |
0.49722 | |
ea = | |
0.83201 | |
0.49995 | |
x = | |
0.29701 | |
0.49722 | |
ea = | |
0.021392 | |
0.012890 | |
ea = | |
1.4786e-05 | |
8.9091e-06 | |
ea = | |
7.0642e-12 | |
4.2565e-12 | |
```octave | |
x | |
X0=fsolve(@(x) mass_spring(x),[3;5]) | |
``` | |
x = | |
0.30351 | |
0.50372 | |
X0 = | |
0.30351 | |
0.50372 | |
```octave | |
[X,Y]=meshgrid(linspace(0,10,20),linspace(0,10,20)); | |
[N,M]=size(X); | |
F=zeros(size(X)); | |
for i=1:N | |
for j=1:M | |
[f,~]=mass_spring([X(i,j),Y(i,j)]); | |
F1(i,j)=f(1); | |
F2(i,j)=f(2); | |
end | |
end | |
mesh(X,Y,F1) | |
xlabel('x_1') | |
ylabel('x_2') | |
colorbar() | |
figure() | |
mesh(X,Y,F2) | |
xlabel('x_1') | |
ylabel('x_2') | |
colorbar() | |
``` | |
 | |
 | |
```octave | |
``` |